Open Access
ARTICLE
A Hybrid Deep Learning Approach for Green Energy Forecasting in Asian Countries
1 School of Computer Science, Chengdu University, Chengdu, 610106, China
2 Information Technology Services, University of Okara, Okara, 56310, Pakistan
3 MLC Research Lab, University of Okara, Okara, 56300, Pakistan
4 Department of Mathematics, University of Okara, Okara, 56310, Pakistan
5 School of Technology and Innovations, University of Vaasa, Vaasa, 65200, Finland
* Corresponding Author: Muhammad Faheem. Email:
Computers, Materials & Continua 2024, 81(2), 2685-2708. https://doi.org/10.32604/cmc.2024.058186
Received 06 September 2024; Accepted 30 September 2024; Issue published 18 November 2024
Abstract
Electricity is essential for keeping power networks balanced between supply and demand, especially since it costs a lot to store. The article talks about different deep learning methods that are used to guess how much green energy different Asian countries will produce. The main goal is to make reliable and accurate predictions that can help with the planning of new power plants to meet rising demand. There is a new deep learning model called the Green-electrical Production Ensemble (GP-Ensemble). It combines three types of neural networks: convolutional neural networks (CNNs), gated recurrent units (GRUs), and feedforward neural networks (FNNs). The model promises to improve prediction accuracy. The 1965–2023 dataset covers green energy generation statistics from ten Asian countries. Due to the rising energy supply-demand mismatch, the primary goal is to develop the best model for predicting future power production. The GP-Ensemble deep learning model outperforms individual models (GRU, FNN, and CNN) and alternative approaches such as fully convolutional networks (FCN) and other ensemble models in mean squared error (MSE), mean absolute error (MAE) and root mean squared error (RMSE) metrics. This study enhances our ability to predict green electricity production over time, with MSE of 0.0631, MAE of 0.1754, and RMSE of 0.2383. It may influence laws and enhance energy management.Keywords
Cite This Article
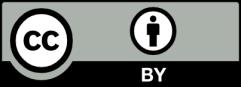
This work is licensed under a Creative Commons Attribution 4.0 International License , which permits unrestricted use, distribution, and reproduction in any medium, provided the original work is properly cited.