Open Access
ARTICLE
Attribute Reduction on Decision Tables Based on Hausdorff Topology
1 Institute of Information Technology, Vietnam Academy of Science and Technology, Hanoi, 10000, Vietnam
2 Faculty of Information Technology, University of Economics-Technology for Industries, Hanoi, 10000, Vietnam
3 VNU Information Technology Institute, Vietnam National University, Hanoi, 10000, Vietnam
4 International School, Vietnam National University, Hanoi, 10000, Vietnam
5 Center of Science and Technology Research and Development, Thuongmai University, Hanoi, 10000, Vietnam
* Corresponding Author: Cu Nguyen Giap. Email:
(This article belongs to the Special Issue: Advanced Data Mining Techniques: Security, Intelligent Systems and Applications)
Computers, Materials & Continua 2024, 81(2), 3097-3124. https://doi.org/10.32604/cmc.2024.057383
Received 16 August 2024; Accepted 16 October 2024; Issue published 18 November 2024
Abstract
Attribute reduction through the combined approach of Rough Sets (RS) and algebraic topology is an open research topic with significant potential for applications. Several research works have introduced a strong relationship between RS and topology spaces for the attribute reduction problem. However, the mentioned recent methods followed a strategy to construct a new measure for attribute selection. Meanwhile, the strategy for searching for the reduct is still to select each attribute and gradually add it to the reduct. Consequently, those methods tended to be inefficient for high-dimensional datasets. To overcome these challenges, we use the separability property of Hausdorff topology to quickly identify distinguishable attributes, this approach significantly reduces the time for the attribute filtering stage of the algorithm. In addition, we propose the concept of Hausdorff topological homomorphism to construct candidate reducts, this method significantly reduces the number of candidate reducts for the wrapper stage of the algorithm. These are the two main stages that have the most effect on reducing computing time for the attribute reduction of the proposed algorithm, which we call the Cluster Filter Wrapper algorithm based on Hausdorff Topology. Experimental validation on the UCI Machine Learning Repository Data shows that the proposed method achieves efficiency in both the execution time and the size of the reduct.Keywords
Cite This Article
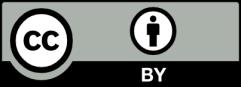
This work is licensed under a Creative Commons Attribution 4.0 International License , which permits unrestricted use, distribution, and reproduction in any medium, provided the original work is properly cited.