Open Access
ARTICLE
GL-YOLOv5: An Improved Lightweight Non-Dimensional Attention Algorithm Based on YOLOv5
School of Software, Dalian Jiaotong University, Dalian, 116000, China
* Corresponding Author: Chen Guo. Email:
Computers, Materials & Continua 2024, 81(2), 3281-3299. https://doi.org/10.32604/cmc.2024.057294
Received 14 August 2024; Accepted 27 September 2024; Issue published 18 November 2024
Abstract
Craniocerebral injuries represent the primary cause of fatalities among riders involved in two-wheeler accidents; nevertheless, the prevalence of helmet usage among these riders remains alarmingly low. Consequently, the accurate identification of riders who are wearing safety helmets is of paramount importance. Current detection algorithms exhibit several limitations, including inadequate accuracy, substantial model size, and suboptimal performance in complex environments with small targets. To address these challenges, we propose a novel lightweight detection algorithm, termed GL-YOLOv5, which is an enhancement of the You Only Look Once version 5 (YOLOv5) framework. This model incorporates a Global DualPooling NoReduction Blend Attention (GDPB) module, which optimizes the MobileNetV3 architecture by reducing the number of channels by half and implementing a parallelized channel and spatial attention mechanism without dimensionality reduction. Additionally, it replaces the conventional convolutional layer with a channel shuffle approach to overcome the constraints associated with the Squeeze-and-Excitation (SE) attention module, thereby significantly improving both the efficiency and accuracy of feature extraction and decreasing computational complexity. Furthermore, we have optimized the Variable Normalization and Attention Channel Spatial Partitioning (VNACSP) within the C3 module of YOLOv5, which enhances sensitivity to small targets through the application of a lightweight channel attention mechanism, substituting it for the standard convolution in the necking network. The Parameter-Free Spatial Adaptive Feature Fusion (PSAFF) module is designed to adaptively modify the weights of each spatial position through spatial pooling and activation functions, thereby effectively enhancing the model’s ability to perceive contextual information over distances. Ultimately, GL-YOLOv5 performs remarkably in the custom dataset, achieving a model parameter count of 922,895 M, a computational load of 2.9 GFLOPS, and a mean average precision (mAP) of 92.1%. These advancements significantly improve the model’s detection capabilities and underscore its potential for practical applications.Keywords
Cite This Article
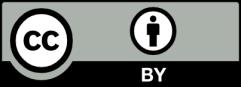
This work is licensed under a Creative Commons Attribution 4.0 International License , which permits unrestricted use, distribution, and reproduction in any medium, provided the original work is properly cited.