Open Access
REVIEW
AI-Powered Innovations in High-Tech Research and Development: From Theory to Practice
1 Department of Arts, Communications and Social Sciences, University Canada West, Vancouver, BC V6Z 0E5, Canada
2 GUS Institute, Global University Systems, London, EC1N 2LX, UK
3 Hamta Group, Hamta Business Corporation, Vancouver, BC V6E 1C9, Canada
4 Q Minded, Quark Minded Technology Inc., Vancouver, BC V6E 1C9, Canada
* Corresponding Author: Mitra Madanchian. Email:
(This article belongs to the Special Issue: AI and Advanced High-Tech Research and Development)
Computers, Materials & Continua 2024, 81(2), 2133-2159. https://doi.org/10.32604/cmc.2024.057094
Received 07 August 2024; Accepted 24 September 2024; Issue published 18 November 2024
Abstract
This comparative review explores the dynamic and evolving landscape of artificial intelligence (AI)-powered innovations within high-tech research and development (R&D). It delves into both theoretical models and practical applications across a broad range of industries, including biotechnology, automotive, aerospace, and telecommunications. By examining critical advancements in AI algorithms, machine learning, deep learning models, simulations, and predictive analytics, the review underscores the transformative role AI has played in advancing theoretical research and shaping cutting-edge technologies. The review integrates both qualitative and quantitative data derived from academic studies, industry reports, and real-world case studies to showcase the tangible impacts of AI on product innovation, process optimization, and strategic decision-making. Notably, it discusses the challenges of integrating AI within complex industrial systems, such as ethical concerns, technical limitations, and the need for regulatory oversight. The findings reveal a mixed landscape where AI has significantly accelerated R&D processes, reduced costs, and enabled more precise simulations and predictions, but also highlighted gaps in knowledge transfer, skills adaptation, and cross-industry standardization. By bridging the gap between AI theory and practice, the review offers insights into the effectiveness, successes, and obstacles faced by organizations as they implement AI-driven solutions. Concluding with a forward-looking perspective, the review identifies emerging trends, future challenges, and promising opportunities in AI-powered R&D, such as the rise of autonomous systems, AI-driven drug discovery, and sustainable energy solutions. It offers a holistic understanding of how AI is shaping the future of technological innovation and provides actionable insights for researchers, engineers, and policymakers involved in high-tech Research and Development (R&D).Keywords
The continuous change known as digitalization greatly impacts modern society [1]. Artificial intelligence (AI) is a comprehensive branch of computer science that is dedicated to the development of intelligent machines that are capable of completing tasks that are typically associated with human intelligence [2]. Deep and machine learning (ML) development is the primary factor influencing this perception [3–5]. These developments lower the expenses involved in changing each field or data type and enable the application of AI across various topic areas and data kinds. As a result, general AI is developing more broadly [6,7], opening up new applications for multiple purposes and data types. Given these noteworthy developments, scientists, businesspeople, and legislators view AI as vital for advancing economic and technical improvement [8,9]. Global economic change and the latest wave of scientific and technological upheaval are largely driven by AI [10]. Like the Internet did thirty years ago or electricity a century ago, AI is predicted to revolutionize every industry, generating an estimated $13 trillion in GDP growth between now and 2030 [11].
The discussion pertains to the definition of efficiency and productivity in research and the epistemological benefit of expediting the process to remain current. Although AI is perceived as having significant potential to facilitate the exchange of interdisciplinary knowledge, the potential consequences of utilizing AI to advance research policy and funders’ agendas may be more profound. These concepts may challenge conventional notions of a university and the definition of an academic, which may or may not have beneficial repercussions [12]. When it comes to technology-driven innovation, management scholars have so far mostly addressed issues like the challenges facing organizations when implementing AI systems and how AI can assist organizational decision-making, business models, operations, and processes [13].
The last ten years have seen a boom in AI and ML thanks to ground-breaking developments in computational technology. The capacity to gather and handle massive amounts of data has significantly improved [14]. Fig. 1 shows the main AI R&D milestones from early expert systems to powerful ML and deep learning models. Given AI’s capacity to do conventional “human” functions in organizations, one can wonder if AI has a place in the pursuit of innovation, one of the key activities influencing a firm’s long-term survival and competitive advantage [15].
Figure 1: Evolution of AI technologies in R&D
AI is an emerging industry with significant competition and high entry barriers. Enterprises can only endure in a highly competitive environment by consistently investing in research and development (R&D). Nevertheless, the same level of R&D investment does not necessarily result in the same proportion of innovation performance enhancement [16]. Businesses may incorporate AI into their innovation processes for several important reasons, even though it may have drawbacks compared to people [15].
Digital transformation applications are prevalent due to the rapid development of digital network infrastructures and smart devices. Big data from smart digital gadgets has grown significantly. AI-driven big data processing systems that use pattern recognition, ML, and deep learning can handle large-scale heterogeneous data [17]. Given that the various R&D modes are typically linked to distinct managerial practices and organizational frameworks, examining how AI affects R&D could have significant managerial ramifications [18,19]. Using paradox theory as support, a recent study on whether AI leads to automation or augmentation contends that the two outcomes are linked over time and across functions rather than mutually exclusive [20].
Beyond encouraging both exploratory and exploitative breakthroughs, AI has the ability to improve organizational performance as a whole. Nevertheless, little is known about how R&D performance and AI capabilities interact [21]. High-tech R&D involves developing new goods or processes using cutting-edge technology in industries with high innovation and complexity [22,23]. High-tech products and industries are defined by high levels of R&D intensity, sophisticated technology, and the capacity to develop quickly, rendering outdated technologies obsolete [24,25]. High-tech R&D uses modern technologies like AI to innovate and address complicated issues across sectors [26,27]. AI-powered innovations use AI to improve processes, goods, and services, improving efficiency, effectiveness, and value generation for enterprises and their consumers [28]. This review will focus on how high-tech fields conceptualize and implement AI-powered breakthroughs.
The principal aim of this review is to close the current gap between theoretical developments and real-world applications of AI in high-tech R&D. Though a lot of research has been done on the theoretical possibilities of AI technologies like neural networks and ML algorithms, there aren’t many thorough examinations that compare these theoretical models to actual applications and results. For example, theoretical frameworks frequently highlight how AI may improve predictive analytics and optimize intricate processes [29,30]. The rigorous examination of how these theoretical potentials translate into real-world advantages and difficulties in various high-tech industries, including biotechnology, automotive, and aerospace, nevertheless needs to be explored in the literature.
To close this gap, this review offers a thorough comparative analysis that examines both cases when AI has performed as expected and successful applications, giving a balanced viewpoint. This review will add to a more sophisticated understanding of the usefulness of AI innovations in high-tech R&D by combining insights from academic research and industry case studies.
R&D projects in high-tech sectors design new goods employing both established and innovative technologies. In fact, new technologies are often created concurrently with product design in such initiatives [22]. AI transforms conventional processes and makes new product development possible, therefore acting as both an inventor and facilitator of creativity [31]. Green radical innovation (GRI) resulting from AI integration in R&D is vital for high-tech companies’ sustainable development [10]. By means of predictive maintenance and effective resource allocation, AI technologies increase operational efficiency, so greatly lowering downtime and improving dependability [32]. Keystones that enable AI-driven innovation and enable companies to adapt and flourish in dynamic contexts include continuous learning and data analytics [17].
AI integration into R&D is based on numerous theories, including the Dynamic Capabilities Theory. This paradigm highlights an organization’s ability to integrate, grow, and reconfigure internal and external competences for quickly changing environments [33]. AI enables real-time data analysis and decision-making, helping firms adjust quickly to market demands. According to the Behavioral Theory of the Firm, organizations operate on bounded rationality and organizational learning, and AI systems may analyze large datasets to improve uncertainty-based decision-making [34]. AI automates regular work, generates ideas, and optimizes resource allocation, improving enterprises’ innovation capability [5].
AI has advantages but also assumptions and limitations. AI systems depend on data availability and quality, and poor data quality can lead to erroneous predictions and insights, reducing R&D AI effectiveness [34]. Organizations need to carefully handle ethical challenges including data privacy, algorithmic bias, and job displacement when integrating AI [33]. Over-reliance on AI can impede innovation and limit the importance of human intuition in the creative process, hence AI adoption in R&D must balance human creativity and machine efficiency [34].
AI-enabled systems at high-tech firms are changing their culture, but how this affects professional work is not understood [35]. AI’s involvement in high-tech R&D, from its early implementations in specific jobs to its current integration across varied industries, is explained in this section. It explains how ML, neural networks, and natural language processing have improved R&D efficiency and creativity. This section also discusses AI advances that enable complicated decision-making and predictive analytics, setting the framework for theoretical developments and practical implementations.
When a business or research organization creatively uses new information and technology to improve products and technologies, it’s referred to as R&D [36]. R&D involves systematic creative work to increase knowledge, including knowledge of man, culture, and society, and the use of this knowledge to create new applications. It spans basic research to application and development and can lead to competitive advantages [37].
Early on, AI applications in R&D were mostly restricted to particular chores such as data processing and pattern detection. Expert systems were among the first AI technologies used in scientific study and engineering, which replicate human decision-making using rule-based reasoning. These systems gave a basis for improving decision-making procedures and automating repetitive chores [38–40]. However, their limited adaptability and dependence on set guidelines could have enhanced their relevance in dynamic and sophisticated R&D settings.
Largely enhanced data quantities are anticipated for the upcoming generation of scientific investigations. AI will be required to automate the data-gathering processes and move the analysis of these experiments forward. Large-scale, interdisciplinary AI programs for science and engineering ought to receive significant funding for R&D from the government for all of these reasons [41]. Basic ML algorithms and expert systems first emerged in R&D in the middle of the 20th century, marking the beginning of AI. From a theoretical idea to a useful tool applied across many high-tech sectors, developments in processing power, data availability, and algorithmic sophistication throughout the decades have driven AI [42–44]. Deep learning, a subclass of ML comprising neural networks with several layers, has shown a great ability to manage vast amounts of data and reveal complex trends. This has produced discoveries in disciplines including genomics, materials science, and drug development. For example, deep learning algorithms have been applied to forecast protein structures, therefore hastening the discovery of possible drug targets and saving the time needed for experimental confirmation [45]. Fig. 2 shows how AI boosts creativity and efficiency, solves complicated problems, personalizes user experiences, and helps data-driven decision-making.
Figure 2: The impact of AI on high-tech industries
Beyond data analysis, AI affects R&D by using automation and optimizing experimental procedures. Labs now have robotics and AI-driven automation systems that provide exact control of experimental settings and high-throughput screening. This integration has simplified procedures, raised repeatability, and let scientists concentrate on their job’s more strategic and creative elements. AI-driven methods have been applied in materials research to forecast the features of new materials, thereby guiding experimental activities and lowering the trial-and-error phase [46].
In R&D, AI has also helped create predictive analytics and simulation models. Driven by ML, predictive analytics lets researchers project trends and results from past data. This capacity is absolutely important in disciplines like climate research, where accurate forecasts are vital for policy and planning. AI-enhanced simulation models offer virtual environments for testing hypotheses and investigating circumstances that would be expensive or unworkable for experimental study. For instance, AI-driven simulations are utilized in aerospace engineering to maximize aircraft designs and enhance safety without requiring large-scale physical prototypes [47–49].
These developments, notwithstanding, provide difficulties for incorporating AI into R&D. Still, important technical challenges include data quality, algorithm interpretability, and computer resource constraints. Ethical issues around data privacy, prejudice, and the possibility of job displacement also need attention. Their sustainable acceptance in R&D depends on AI systems being open, fair, and consistent with human values [50].
2.2 Importance of AI in High-Tech Industries
AI has become a major player in high-tech sectors since it drives unheard-of breakthroughs and changes in established procedures. High-tech sectors have been able to innovate quickly because of the combination of AI technologies, including ML, neural networks, and natural language processing. The diversity of research themes in bulks of scholarly literature published in key research channels reflects scholars’ and practitioners’ rising interest in AI [51].
Information exchange and efforts to create new goods and services have been mostly the domain of marketing and R&D departments for many years. It is doubtful that all pertinent information can be located in a single company or department, though, because knowledge is widely dispersed [52]. The innovation ecosystem is improved by natural language processing, which makes it easier for researchers to collaborate and share knowledge [36]. By processing enormous volumes of data, identifying trends, and making predictions, AI has transformed industries, including biotechnology, automotive, and aerospace, transforming R&D operations.
AI has sped drug discovery and development in biotechnology. Analyzing biological data and using ML techniques to find possible medication candidates, project their efficacy, and guide drug design. Along with accelerating the R&D process, this lowers expenses and raises the possibility of positive results. AI has been very helpful in the fight against diseases like COVID-19 [53] in uncovering fresh uses for current medications, a process sometimes referred to as drug repurposing.
Autonomous cars’ development greatly benefits the automobile sector from AI developments, which revolve around AI-powered systems that provide real-time data processing and decision-making needed for safe and effective self-driving cars. Through accident prediction and prevention, advanced driver-assistance systems (ADAS) use AI to improve vehicle safety. AI is also utilized in predictive maintenance, in which it examines vehicle data to forecast possible faults and schedule proactive repairs, hence lowering downtime and increasing dependability [54].
Beyond these illustrations, AI plays almost every function in highly advanced sectors. Intelligent systems and AI-driven automation have improved manufacturing techniques, raised product quality, and stimulated invention. Big data analysis has produced more informed decisions, strategic planning, and tailored client experiences. The influence of AI on high-tech sectors will only become more evident as it develops, therefore fostering new possibilities for investigation and growth as well as more advances.
Finally, it is impossible to overestimate the significance of AI in high-tech sectors. Its ability to transform R&D has promoted efficiency, creativity, and economy in many fields. High-tech companies embracing AI will be able to reach even more amazing technical innovations and achievements [55]. The fast developments in AI have significantly affected several fields, including R&D in many different sectors [56,57]. Understanding the fundamental theoretical concepts and frameworks influencing AI development would help one fully exploit its possibilities in R&D [58,59].
Early on in AI, the emphasis was on creating rule-based systems to address problems using explicit logical rules and heuristics based on. Still, as the availability of big datasets and processing capability grew, the area has shifted to statistical and machine-learning approaches, allowing AI systems to learn from data and make informed judgments [60]. DARPA has noted that this development has produced three separate waves of AI [61]. Whereas the second wave prioritized data-driven methods, the first stressed the value of handcrafted knowledge. Seeking more human-like decision-making and trustworthy human-AI cooperation, the third wave—which tries to mix the strengths of knowledge and data-driven approaches—is now beginning to emerge.
This study uses a comparative review to bridge theoretical models and practical applications to provide a balanced and comprehensive knowledge of AI-powered high-tech R&D developments. This approach is especially essential in AI, where rapid technology breakthroughs sometimes exceed real-world applications. This review provides a more holistic view of AI’s effectiveness and problems in R&D by comparing theoretical models, algorithms, and frameworks with their real implementations across high-tech domains. The comparative technique shows AI technology achievements and failures as well as contextual aspects that affect performance in diverse situations. The literature search method includes databases, keywords, and search parameters used to find relevant studies. This section details inclusion, exclusion, data collecting, and analysis. This methodology examines theoretical models and AI applications in high-tech R&D in a methodical manner.
To conduct a literature review on “AI-Powered Innovations in High-Tech R&D: From Theory to Practice” using the Scopus database, we first define primary concepts and keywords related to AI, high-tech R&D, and their theoretical and practical aspects. Keywords include “artificial intelligence,” “machine learning,” “neural networks,” “deep learning,” “high-tech,” “research and development,” “technology development,” “innovation,” “conceptual framework,” theory, and practice. We then construct comprehensive search queries combining these keywords using Boolean operators, such as: (“artificial intelligence” OR AI OR “machine learning” OR “neural networks” OR “deep learning”) AND (“high-tech” OR “research and development” OR R&D OR “technology development” OR innovation).
(Title/Abstract/Keywords (“artificial intelligence” OR AI OR “machine learning” OR “neural networks” OR “deep learning”) AND Title (“research and development” OR “R&D”)).
• Inclusion Criteria:
∘Peer-reviewed journal articles.
∘Studies and articles published within the last 10 years (2014–present).
∘The research focused on AI applications in R&D.
Exclusion Criteria:
∘ Reviews, books, book chapters, editorials, conference papers, surveys, etc.
∘ Research focused on AI R&D.
∘ Articles not available in full text.
Peer-reviewed journal articles on AI applications in high-tech R&D published within the last 10 years (2014–present) were carefully selected for inclusion. This criterion ensures that the assessment considers industry trends, fashions, and technologies. The emphasis on peer-reviewed publications ensures properly studied research, which strengthens the conclusions. We considered theoretical and practical research since the report evaluates AI-powered innovations’ real-world application. Exclusion criteria ensured review clarity and relevance. Reviews, books, book chapters, editorials, conference papers, and polls were ignored to focus on original AI R&D research. Conference papers that could be expanded in peer-reviewed publications were omitted to avoid repetition. Books and book chapters can dive into topics, but include them in a peer-reviewed research article may dilute the detail. We wanted to give a quick, concentrated study for academics, practitioners, and policymakers interested in the latest AI breakthroughs and uses in high-tech industries by eliminating these sources. We excluded studies that were not available in full text since critical evaluation of methodologies, data, results, and conclusions requires complete text. To avoid translation biases and ensure analytical consistency, non-English research was removed.
Fig. 3 illustrates the literature review process, depicting the stages from initial search and document identification. This search identified 593 documents. We then applied criteria to include only peer-reviewed journal articles from the last 10 years focusing on AI in R&D, excluding reviews, books, and non-full-text articles. This process filtered 136 articles and further narrowed them down to 35 based on Title and abstract review before final inclusion in the review.
Figure 3: Stages of selecting papers
∘ Articles are limited to those available in the Scopus database.
∘ Focused on high-tech industries, potentially excluding relevant studies from other fields.
∘ Potential bias due to the inclusion of only peer-reviewed articles.
∘ Studies published in languages other than English may be excluded.
AI can help solve climate change by promoting sustainable development [62]. Fig. 4 compares the performance of many AI methods (e.g., decision trees, reinforcement learning, and neural networks) across several high-tech industries, including renewable energy, biotechnology, automotive, and aerospace. Hermann et al. [62] proposed a unique chemical R&D process underpinned by AI and ethical principles to account for process- and outcome-related sustainability. In ethically salient contexts, AI R&D should promote social and environmental good and sustainability (beneficence) while preventing harm (non-maleficence) for all stakeholders (companies, individuals, and society at large). Several theoretical models and frameworks that direct its application and use serve as the foundation for the integration of AI in R&D. The Assignment Decision Support System (ADSS), put forth by Liu et al. [63], is one noteworthy framework that optimizes the reviewer assignment process in R&D project selection by fusing operations research methodologies with heuristic knowledge. By increasing assigned reviewers’ knowledge, balancing proposal distribution, and avoiding conflicts of interest, this system seeks to improve the quality of project selection. The ADSS exemplifies how AI can enhance R&D decision-making procedures, resulting in more fair and knowledgeable assessments. The R&D Process Improvement System, created by Lee et al. [64], is another important theoretical model that evaluates and improves R&D processes in small and medium-sized businesses (SMEs). To find and fix flaws in R&D operations, this system combines performance level analysis, comparison analysis, and performance simulation analysis. Especially for resource-constrained firms, this framework offers an organized method for increasing R&D management efficiency and effectiveness by modeling the influence of important processes on R&D outcomes.
Figure 4: AI algorithms for different high-tech sectors
Project selection is key to innovation management in R&D firms. Selection methods must be improved in public sector R&D. These enhancements should address these organizations’ unique obstacles to improve decision-making. Modern DSS can meet these needs. Effective DSS supported by good data management and AI can improve public sector R&D innovation management [65]. Jang’s [66] decision support framework maximizes R&D production in budget allocation by combining ML and optimization methodologies. This approach provides more effective and efficient resource allocation by projecting future R&D outcomes and includes robust optimization to control uncertainty. The approach shows how AI may significantly increase the expected returns on R&D investments by transforming budgetary decisions. Wang et al.’s exploration [67] of the idea of cascading failures in R&D networks presents a methodology to comprehend and reduce schedule risks by managing cascading failures. This framework aids in developing methods to lower the risk of delays in R&D projects by utilizing the BBV algorithm and the CA model. This theoretical advancement emphasizes how crucial it is to comprehend network dynamics and take preventative action to guarantee project deadlines are met.
Table 1 lists studies’ theoretical frameworks, R&D applications, and AI technologies’ roles in improving R&D. Zhang et al.’s Knowledge Spillover Effect model [68] uses spatial econometrics to examine how innovation spreads throughout different geographic areas. This approach captures the influence of proximities in terms of geography, technology, economy, and human capital on R&D activities by employing a variety of spatial weight matrices. The results emphasize the differing effects of local vs. transnational knowledge spillovers and stress the significance of spatial aspects in promoting innovation. Villani [69] demonstrated that the neural network methodology and Least Squares Monte Carlo approach can help value R&D investment opportunities. The start of the next phase of an R&D project depends on completing the previous phase. This sequential investment makes R&D initiatives compound choices. R&D investments frequently have high-cost unpredictability, making them an exchange alternative for an uncertain gross project value. Finally, production investment can be made before maturity, and R&D impacts diminish. Thus, R&D is a compound American exchange option. The Least Squares Monte Carlo approach is effective and adaptable for capital budgeting and valuing American-type options. But, using the simulated values as “targets,” a neural network can expand the results for any R&D valuation and reduce the least-squares Monte Carlo simulation time. Geerts et al.’s study [70] on Quantitative Systems Pharmacology (QSP) for CNS drug discovery similarly revolves mostly around simulations and predictive analytics. The QSP model offers an understanding of the possible clinical results of drug candidates by simulating the impact of therapeutic interventions on neural circuit activity. This method raises the success rate of CNS R&D projects and increases the predictive value of preclinical models.
AI is changing industry practices and spurring innovation in high-tech sectors. AI adoption success gives companies a competitive edge in their markets. Fig. 5 depicts data collection, preprocessing, model training, validation, and deployment of AI-driven predictive analytics for R&D. This section explores the practical applications of AI in high-tech industries, including important impediments to adoption, lessons learned from successful implementations, and the impact on industry practices and outcomes.
Figure 5: Flowchart of AI-driven predictive analytics in high-tech R&D
5.1 Implementation in Industry
From theory to practice, several studies have proposed a theoretical framework for analyzing cooperation dynamics and practical suggestions for improving R&D collaboration. Zhou et al. [72] used patent application data from 2337 Chinese biopharmaceutical enterprises to offer a combined impact framework to examine R&D team networks, knowledge diversity, and breakthrough technological innovation. It uses K-means clustering and decision tree models to identify three R&D team configurations—tight cooperation, knowledge expansion, and scale orientation—highlighting the crowding-out and complementary effects of knowledge diversity and team network features. Using data mining approaches to innovation management, the study addresses gaps in linear effect studies and improves social network theory, delivering actionable insights for organizations. A network model based on patent data and evolutionary game theory on complex networks was used by Guo et al. [73] to study how heterogeneous reference-point-based aspirations affect R&D alliance portfolio cooperation and recurrent project collaboration cooperation. It shows that aggressive decision-makers (DMs) are more likely to cooperate and that effort complementarity, knowledge rearrangement, and supervision affect cooperation tactics. It advises governments to identify and manage ambition to boost R&D cooperation and use detailed contracts with enterprises with high knowledge reorganization capabilities. Keding et al. [74] took a psychological approach to AI-augmented decision-making, examining theoretical and practical impacts. It theoretically examines how AI and human judgment interact, showing a transition from algorithm aversion to appreciation. The study uses vignettes to show that AI-based advising systems improve choice behavior and perception of decision quality among 150 senior executives. The findings imply that overreliance on AI is caused by increased trust in AI advisers and a perceived more structured decision-making process, providing strategic innovation management insights.
Operational efficiency and effectiveness have advanced greatly as AI and ML have been included in many sectors. For instance, Viberg et al.’s study [75] showed how ML improves knowledge integration inside technological companies. These firms increase their organizational performance and innovation capacity by using ML to transcribe spoken words and combine implicit and explicit knowledge. This useful application of ML shows how using cutting-edge data analysis techniques may simplify procedures and enable improved decision-making in high-tech companies.
Regarding energy management, Liao et al. [76] created a prediction model for air-conditioning energy usage using several ML approaches. Their research showed how highly accurate techniques—including random forest and deep neural networks—can estimate energy use. Apart from helping to lower energy usage, this model offers insightful analysis for building owners to maximize their energy management techniques. Industries driven by sustainability and resource efficiency are seeing a growing frequency of AI and ML use in forecasting and energy consumption control.
Lutnick et al. [77] first presented an AI system for histology image processing in pharmaceutical R&D. Through an open-source platform; this user-friendly solution lets non-data scientists—such as doctors—analyze and interpret challenging histology data. Using effective data cataloging, interactive visualization, and model deployment made possible by integrating AI tools, R&D is accelerated, and multidisciplinary cooperation is enhanced. Chen et al. [78] also used AI technology and computer molecular simulation to advance drug R&D. Their strategy greatly raised the safety and efficiency of medication development procedures, demonstrating how AI may transform the pharmaceutical sector by improving research methods and results. The research on the use of AI and cutting-edge technologies in several R&D fields is compiled in Table 2.
The study by Jun et al. [80] on a hybrid recommendation model for R&D cooperation showed how ML may greatly improve the success rate of SMEs’ alliances with different R&D companies. The approach has helped SMEs choose suitable partners and enhance their R&D results by precisely estimating the possible success of partnerships with public research institutes, academic institutions, and other companies. The creation of a decision assistance system by Kocak et al. [84] for choosing reviewers for R&D projects shows still another noteworthy result. Classifying projects and ranking reviewers using natural language processing and fuzzy multi-criteria decision-making techniques, this AI-based system, the effective application of the system has raised the accuracy and efficiency of the review process, thereby proving how AI may simplify difficult decision-making responsibilities and increase the quality of R&D project evaluations.
Regarding drug research, Huang et al. [85] presented HiRAND, a new deep learning-based system for drug response prediction. Using gene and sample data integration via graph convolutional networks, HiRAND beats conventional techniques. The framework’s capacity to identify important genes engaged in drug reactions and forecast drug responses with great accuracy highlights its possibilities to progress personalized medicine and drug development. The achievements of HiRAND show how AI and ML could improve predicted accuracy and support innovative drug development ideas.
5.2 Challenges in Practical Implementation
Despite the encouraging developments, applying AI and ML ideas in real-world environments presents various difficulties. Behr et al. [89] underlined in their research the challenges in interpreting RWD in pharmaceutical R&D. Managing limited and skewed data is one of the difficulties; this might result in errors in AI-driven findings. They underline the requirement of strict approaches and domain knowledge to solve these problems and guarantee that AI studies yield accurate and practical findings. The use of ML to forecast energy demand also presents difficulties. Although deep neural networks and random forests are among ML models that exhibit great accuracy, their efficacy relies on the quality and volume of the data. Maintaining their predictive ability depends on constant updates and validation for the models, which can be challenging and costly for resources. Including these models in current energy management systems presents technological and logistical difficulties that need to be resolved if effective deployment is to take place [76].
Gutierrez et al. [86], on AI in R&D, reviewed the difficulties of hastening R&D using intelligent experiment design. The study emphasizes that although AI can maximize data management and experiment design, the shift from conventional trial-and-error procedures to data-driven approaches calls for overcoming cultural and procedural constraints inside research organizations. Adopting AI-driven solutions may be greatly hampered by researchers’ adaptation to new technology and methods.
AI and ML implementation comes with difficulties and mistakes, with no exceptions. Tafferner et al. [83] expressed some limits of ChatGPT in electronics R&D, namely in offering correct recommendations for sensors and hardware design. The sporadic mistakes in the ideas and created references draw attention to the requirement of careful and critical review of AI-generated content. This event emphasizes the need to confirm AI outputs and add knowledge to support them in front of false information and guarantee the dependability of technical advice. In low-performance R&D project prediction, Ma et al. [90] discovered that although their ML model showed promise, their inability to forecast project outcomes precisely and adequately account for all influencing elements limited it. The framework’s reliance on several elements, including money and organizational traits, may not adequately reflect the complexity of R&D initiatives. This work emphasizes the need to always improve prediction models and include extra factors to raise applicability and accuracy.
Sterlacchini [93] investigated the economic effects of AI patenting and employment, exposing a paradox whereby high AI patenting corresponds with job declines in several industries. This result shows the complicated and even unexpected effects of AI developments on job trends. This study teaches the need to consider AI technologies’ wider social and economic impact so that their deployment corresponds with sustainable and fair development objectives.
AI has a theoretically limitless potential that offers transforming effects in sectors from finance to healthcare. Projections and theoretical models indicate that AI could transform difficult systems, alter decision-making processes, and reach superhuman performance in some tasks. For pattern recognition, predictive analytics, and automation, for example, AI theories hold that ML algorithms could finally surpass human capacity [77]. These ideas stress how quickly and precisely AI can process enormous volumes of data, fostering innovative ideas and efficiency.
Practical results, meantime, expose a more complex reality. Although AI has great theoretical potential, actual uses sometimes run up restrictions that lower expectations. For instance, the study by Behr et al. [89] emphasized the difficulties in evaluating RWD inside pharmaceutical R&D. Data sparsity, prejudice, and the necessity for domain expertise highlight how theoretically capable AI may only sometimes fit pragmatic limitations. Data quality problems and the complexity of real-world situations often impede the actual performance of AI in practice, although developed algorithms and models.
In the same vein, Liao et al. [76] showed in the context of energy consumption prediction that although ML models such as random forests and deep neural networks can achieve high accuracy, their practical implementation depends mostly on the quality of the input data and the model’s continuous verification. Though practical issues like data integration and model maintenance can influence their efficacy, theoretical models indicate that these algorithms can offer exact forecasts. This difference between theoretical potential and actual performance emphasizes how difficult it is to implement AI ideas from theory.
Evaluating the impact and success of AI developments depends critically on their practical application efficiency. One well-known example is an easy-to-use AI system for image analysis of histograms [77]. Designed for non-data scientists like doctors, this system highlights how effectively AI is included in medical research and treatment. The practical advantages of AI developments in improving research efficiency and multidisciplinary cooperation are shown by the capacity to effectively catalog data, visualize results, and employ models in a user-friendly manner. Combining computer molecular simulation with AI technology has simplified medication development, improving efficiency and safety. This success narrative shows how AI developments are expediting R&D operations and enhancing medication development approaches [78].
Predicting the success rate of alliances between SMEs and R&D companies has helped the model enable more strategic cooperation choices, thereby enhancing innovation output. This application emphasizes that AI is important in improving R&D initiatives and encouraging effective teamwork [80]. Even with these achievements, the impact of AI developments is general. For electronics R&D, for example, Tafferner et al. [83] discovered limits in ChatGPT’s ability to provide correct hardware design and sensor recommendations. The investigation exposed sporadic mistakes and fake references, therefore stressing the need for accuracy in the outputs of AI to ensure its efficacy. Such restrictions highlight the need to carefully evaluate i-generated content with professional knowledge and supplement to guarantee consistent results.
To make AI technologies effective and usable across high-tech sectors, a systematic methodology that blends theoretical insights with practical considerations is needed to bridge the gap between theoretical AI concepts and actual R&D implementations. AI algorithms are contextually adapted to R&D environments to manage domain-specific data, regulatory constraints, and operational requirements. Customizing AI models for real-world applications requires interdisciplinary collaboration from computer science, engineering, domain-specific sciences, and business management. Through small-scale pilot studies, AI models are refined through an iterative cycle of design, testing, and validation to address data quality and algorithmic biases. AI technologies must integrate seamlessly with R&D procedures and systems to improve workflows. AI systems must be scalable and flexible, leveraging transfer learning to adapt models across projects. To earn stakeholder trust and comply with rules, practical implementation must include ethical issues including data protection and fairness. Finally, measuring AI impact with measures like time-to-market, cost savings, and quality improvements allows continual monitoring, learning, and development.
AI and ML have great future possibilities and could revolutionize many industries. As AI technologies develop, unprecedented changes in healthcare, banking, transportation, education, and other sectors are predicted. Improvement of AI algorithms and models is one major area of growth. Future AI systems will be more advanced and able to manage difficult tasks with better accuracy and efficiency. AI-driven automation, quantum computing integration, and sustainable R&D may be future AI breakthroughs (Fig. 6).
Figure 6: Future mind map of AI in high-tech R&D
Advances in natural language processing and computer vision will allow AI to more successfully grasp and interpret human languages and visual inputs, improving human-machine interactions and generating more user-friendly AI-driven apps. In the healthcare sector, AI could transform medical research and patient treatment. Future AI systems could offer real-time diagnosis and customized treatment programs by leveraging enormous volumes of patient data and sophisticated analysis tools. Predictive analytics could enable preventative actions to predict illness outbreaks, greatly enhancing public health results. AI-driven drug discovery and development procedures are projected to quicken, saving time and money for launching new drugs. This will be vital to guarantee quick reactions to new ailments and to handle world health issues.
AI models will have automated trading systems, improved risk assessment, and fraud detection. These technologies will examine financial data and market patterns with hitherto unheard-of speed and accuracy, guiding wiser investments. AI-driven customer support systems such as virtual assistants and chatbots will also offer tailored and quick services, raising operational effectiveness and customer happiness for financial institutions. AI integration into financial technologies (Fintech) will inspire creativity, creating fresh financial products and services that meet changing customer demand.
Another industry where AI will be quite important is transportation. Autonomous cars driven by AI change our way of transportation. To properly and effectively negotiate challenging surroundings, these vehicles depend on sophisticated sensors, ML algorithms, and real-time data processing. In addition to lowering human error, this will improve traffic control and help ease congestion, enhancing road safety. AI will also maximize supply chains and logistics, facilitating more effective delivery, inventory control, and routing. More environmentally friendly and reasonably priced mobility options will follow from the general acceptance of AI in transportation.
AI will transform teaching and learning strategies in education. Driven by AI, personalized learning systems will change to fit the demands of certain students and offer tailored materials and comments to improve learning results. These sites will use data analytics to pinpoint areas of strength and weakness in pupils, providing focused tools and assistance. AI-powered tools will also help teachers with administrative chores, including lesson preparation and grading, freeing them to concentrate more on student involvement and instructional quality. By including AI in the classroom, access to top-notch learning materials will be democratized, therefore closing gaps in educational possibilities and promoting lifetime learning.
In addition to improving decision-making, a hybrid strategy that blends human and machine intelligence also solves AI’s drawbacks. AI lacks contextual knowledge and ethical reasoning that human specialists supply, ensuring that innovations are in line with society ideals [96]. Human judgment may greatly enhance algorithmic predictions in difficult problem solving, especially in subtle situations where AI can struggle [97].
To overcome issues like algorithmic bias, a hybrid intelligence system promotes cooperation between human cognition and AI, increasing productivity and creativity [98]. Concerns about an over-reliance on AI, which might erode human intuition and creativity, highlight the necessity for a balanced approach [99]. According to Cohen et al. [100], the utilization of human experience in a “human in the loop” approach can enhance decision-making procedures while preserving efficiency.
The hybrid approach shows promise, but it’s important to be aware of the hazards associated with AI and make sure that human judgment plays a significant part in directing technology progress. Problems with data privacy, security, and algorithmic bias must be addressed as AI systems grow increasingly entwined into society. Maximizing the advantages of AI technology and reducing possible hazards will depend on how they are developed and used ethically. Strong legislative frameworks, multidisciplinary cooperation, and ongoing AI system monitoring and evaluation will all be needed. Moreover, addressing the possible social and financial effects of AI, such as inequality and job displacement, will be crucial to promote inclusive and sustainable development.
AI integration into R&D presents major ethical questions with wider society consequences going beyond specific initiatives (Fig. 7). The possible bias in AI algorithms, which can result in unfair results for certain demographic groups, is one of main worries. For example, if AI systems are taught on biased data, they could reinforce current disparities, therefore influencing hiring policies, healthcare availability, and even law enforcement actions. This prejudice not only compromises the fairness of AI applications but also aggravates social inequities since automated systems devoid of consideration for their particular circumstances might provide greater disadvantage to underprivileged people. Dealing with these prejudices calls for a dedication to openness and responsibility in AI development so that different datasets are used and that the justifiable and understandable AI system decision-making is ensured.
Figure 7: Ethical framework for AI deployment in R&D
AI deployment in R&D runs the danger of social manipulation and invasions of privacy. Concerns about monitoring and the degradation of individual privacy rights get more strong as AI systems grow abler of processing enormous volumes of personal data. Using AI for social manipulation—that is, to change public opinion or behavior by means of deliberate misinformation—has ethical ramifications that seriously jeopardize democratic processes and society confidence. Establishing clear ethical rules that give human rights and social justice first priority together with strong systems of responsibility would help to reduce these hazards. Including many stakeholders in the creation of AI technologies can help to guarantee that these systems are built with ethical issues first in mind, therefore advancing a fairer and equal society.
AI has the potential to revolutionize high-tech R&D across various industries, including biotechnology, automotive, aerospace, renewable energy, Fintech, and cybersecurity. This review has explored the theoretical foundations and practical applications of AI, highlighting its transformative capacity while acknowledging the associated challenges and limitations. Our analysis demonstrates that, while AI offers significant opportunities for innovation and efficiency, its deployment must be managed carefully to ensure ethical considerations and social equity.
The findings indicate that AI can significantly enhance R&D processes by automating repetitive tasks, optimizing decision-making, and enabling data-driven insights. However, these benefits are not without challenges. Technical barriers such as data quality, algorithmic biases, and the integration of AI systems into existing infrastructures can limit the effectiveness of AI-powered innovations. Moreover, social and ethical issues, including data privacy concerns, potential job displacement, and the risk of exacerbating social inequalities, need to be addressed to ensure the responsible use of AI.
Moving forward, several emerging trends and future directions are likely to shape the role of AI in high-tech R&D. AI-driven automation will continue to evolve, enabling more sophisticated and autonomous R&D processes. The integration of quantum computing could further enhance AI capabilities, allowing for the processing of vast datasets at unprecedented speeds, potentially unlocking new scientific discoveries and technological advancements. Moreover, there is a growing emphasis on sustainable R&D practices, where AI can play a critical role in optimizing resource use and reducing environmental impact.
To maximize the benefits of AI while mitigating its risks, we propose a roadmap for future R&D. This includes fostering multidisciplinary collaborations to enhance AI model development, ensuring transparency and fairness in AI algorithms, and developing comprehensive guidelines for ethical AI deployment in high-tech industries. Future research should also focus on understanding the long-term impacts of AI adoption on social structures, workforce dynamics, and economic development.
Acknowledgement: None.
Funding Statement: The authors received no specific funding for this study.
Author Contributions: The authors confirm contribution to the paper as follows: study conception and design: Mitra Madanchian, Hamed Taherdoost; data collection: Mitra Madanchian; analysis and interpretation of results: Mitra Madanchian, Hamed Taherdoost; draft manuscript preparation: Mitra Madanchian, Hamed Taherdoost. All authors reviewed the results and approved the final version of the manuscript.
Availability of Data and Materials: Not applicable.
Ethics Approval: Not applicable.
Conflicts of Interest: The authors declare that they have no conflicts of interest to report regarding the present study.
References
1. J. Alcácer, J. Cantwell, and L. Piscitello, “Internationalization in the information age: A new era for places, firms, and international business networks?” J. Int. Bus. Stud., vol. 47, pp. 499–512, 2016. doi: 10.1057/jibs.2016.22. [Google Scholar] [CrossRef]
2. I. H. Sarker, “AI-based modeling: Techniques, applications and research issues towards automation, intelligent and smart systems,” SN Comput. Sci., vol. 3, no. 2, 2022, Art. no. 158. doi: 10.1007/s42979-022-01043-x. [Google Scholar] [PubMed] [CrossRef]
3. M. Haenlein and A. Kaplan, “A brief history of artificial intelligence: On the past, present, and future of artificial intelligence,” Calif. Manage. Rev., vol. 61, no. 4, pp. 5–14, 2019. doi: 10.1177/0008125619864925. [Google Scholar] [CrossRef]
4. K. Miyazaki, “Adoption of AI in firms and the issues to be overcome—An empirical analyses of the evolutionary path of development by firms,” in 22nd ITS Biennial Conf., Seoul, Republic of Korea, 2018. [Google Scholar]
5. S. A. Kumar et al., “Machine learning and deep learning in data-driven decision making of drug discovery and challenges in high-quality data acquisition in the pharmaceutical industry,” Future Med. Chem., vol. 14, no. 4, pp. 245–270, 2022. doi: 10.4155/fmc-2021-0243. [Google Scholar] [PubMed] [CrossRef]
6. M. Taddy, “The technological elements of Artificial Intelligence,” in The Economics of Artificial Intelligence: An Agenda, Ajay Agrawal, Joshua Gans, Avi Goldfarb (Eds.Chicago: University of Chicago Press, 2019, pp. 61–88. doi: 10.7208/9780226613475-004. [Google Scholar] [CrossRef]
7. H. Taherdoost and M. Madanchian, “Artificial intelligence and knowledge management: Impacts, benefits, and implementation,” Computers, vol. 12, no. 4, 2023, Art. no. 72. doi: 10.3390/computers12040072. [Google Scholar] [CrossRef]
8. M. A. Goralski and T. K. Tan, “Artificial intelligence and sustainable development,” Int. J. Manage. Educ., vol. 18, no. 1, 2020, Art. no. 100330. doi: 10.1016/j.ijme.2019.100330. [Google Scholar] [CrossRef]
9. P. Chojecki, Artificial Intelligence Business: How you Can Profit from AI. Przemek Chojecki, 2020. [Google Scholar]
10. H. Su, X. Qu, S. Tian, Q. Ma, L. Li and Y. Chen, “Artificial intelligence empowerment: The impact of research and development investment on green radical innovation in high-tech enterprises,” Syst. Res. Behav. Sci., vol. 39, no. 3, pp. 489–502, 2022. doi: 10.1002/sres.2853. [Google Scholar] [CrossRef]
11. J. Füller, K. Hutter, J. Wahl, V. Bilgram, and Z. Tekic, “How AI revolutionizes innovation management-Perceptions and implementation preferences of AI-based innovators,” Technol. Forecast. Soc. Change, vol. 178, 2022, Art. no. 121598. doi: 10.1016/j.techfore.2022.121598. [Google Scholar] [CrossRef]
12. J. Chubb, P. Cowling, and D. Reed, “Speeding up to keep up: Exploring the use of AI in the research process,” AI Soc., vol. 37, no. 4, pp. 1439–1457, 2022. doi: 10.1007/s00146-021-01259-0. [Google Scholar] [PubMed] [CrossRef]
13. M. M. Mariani, I. Machado, V. Magrelli, and Y. K. Dwivedi, “Artificial intelligence in innovation research: A systematic review, conceptual framework, and future research directions,” Technovation, vol. 122, 2023, Art. no. 102623. doi: 10.1016/j.technovation.2022.102623. [Google Scholar] [CrossRef]
14. S. Kolluri, J. Lin, R. Liu, Y. Zhang, and W. Zhang, “Machine learning and artificial intelligence in pharmaceutical research and development: A review,” AAPS J., vol. 24, pp. 1–10, 2022. doi: 10.1208/s12248-021-00644-3. [Google Scholar] [PubMed] [CrossRef]
15. N. Haefner, J. Wincent, V. Parida, and O. Gassmann, “Artificial intelligence and innovation management: A review, framework, and research agenda,” Technol. Forecast. Soc. Change, vol. 162, 2021, Art. no. 120392. doi: 10.1016/j.techfore.2020.120392. [Google Scholar] [CrossRef]
16. Y. Dong, Z. Wei, T. Liu, and X. Xing, “The impact of R&D intensity on the innovation performance of artificial intelligence enterprises-based on the moderating effect of patent portfolio,” Sustainability, vol. 13, no. 1, pp. 1–17, 2021. [Google Scholar]
17. A. Aldoseri, K. N. Al-Khalifa, and A. M. Hamouda, “AI-powered innovation in digital transformation: Key pillars and industry impact,” Sustainability, vol. 16, no. 5, 2024, Art. no. 1790. doi: 10.3390/su16051790. [Google Scholar] [CrossRef]
18. P. C. Johnson, C. Laurell, M. Ots, and C. Sandström, “Digital innovation and the effects of artificial intelligence on firms’ research and development—Automation or augmentation, exploration or exploitation?,” Technol. Forecast. Soc. Change, vol. 179, 2022, Art. no. 121636. doi: 10.1016/j.techfore.2022.121636. [Google Scholar] [CrossRef]
19. H. Taherdoost, “Risk management in R&D projects,” in Innovation through Research and Development: Strategies for Success. Cham: Springer, 2024, pp. 247–269. [Google Scholar]
20. S. Raisch and S. Krakowski, “Artificial intelligence and management: The automation-augmentation paradox,” Acad. Manage. Rev., vol. 46, no. 1, pp. 192–210, 2021. doi: 10.5465/amr.2018.0072. [Google Scholar] [CrossRef]
21. V. Kumar, S. Kumar, S. Chatterjee, and M. Mariani, “Artificial Intelligence (AI) capabilities and the R&D performance of organizations: The moderating role of environmental dynamism,” IEEE Trans. Eng. Manage., vol. 71, pp. 1–16, 2024. doi: 10.1109/TEM.2024.3423669. [Google Scholar] [CrossRef]
22. C. Ilas, “Technology readiness impact on high-tech R&D projects,” UPB Sci. Bull. Ser. D: Mech. Eng., vol. 75, no. 2, pp. 241–250, 2013. [Google Scholar]
23. A. Chandrasekaran, K. Linderman, F. J. Sting, and M. J. Benner, “Managing R&D project shifts in high-tech organizations: A multi-method study,” Prod. Oper. Manage., vol. 25, no. 3, pp. 390–416, 2016. doi: 10.1111/poms.12410. [Google Scholar] [CrossRef]
24. A. Zandiatashbar and S. Hamidi, “Exploring the microgeography and typology of US high-tech clusters,” Cities, vol. 131, 2022, Art. no. 103973. doi: 10.1016/j.cities.2022.103973. [Google Scholar] [CrossRef]
25. T. Serediuk and Y. Vdovychenko, “Common features and distinctions between high technology and innovation,” Baltic J. Econ. Stud., vol. 4, no. 5, pp. 315–319, 2018. doi: 10.30525/2256-0742/2018-4-5-315-319. [Google Scholar] [CrossRef]
26. Y. Gao, G. Style, and B. Ambition, “Mapping global innovation,” in the Global Innovation Sweepstakes: A Quest to Win the Future, 2018, pp. 15–45. [Google Scholar]
27. J. M. Bauer, “Entrepreneurship and government in US high-tech policy,” in Innovation Policy and Governance in High-Tech Industries: The Complexity of Coordination. Berlin, Heidelberg: Springer Berlin Heidelberg, 2011, pp. 103–125. [Google Scholar]
28. R. Naeem, M. Kohtamäki, and V. Parida, “Artificial intelligence enabled product-service innovation: Past achievements and future directions,” Rev. Manage. Sci., vol. 157, pp. 1–44, 2024. doi: 10.1007/s11846-024-00757-x. [Google Scholar] [CrossRef]
29. M. I. Jordan and T. M. Mitchell, “Machine learning: Trends, perspectives, and prospects,” Science, vol. 349, no. 6245, pp. 255–260, 2015. doi: 10.1126/science.aaa8415. [Google Scholar] [PubMed] [CrossRef]
30. Y. LeCun, Y. Bengio, and G. Hinton, “Deep learning,” Nature, vol. 521, no. 7553, pp. 436–444, 2015. [Google Scholar] [PubMed]
31. A. Brem, F. Giones, and M. Werle, “The AI digital revolution in innovation: A conceptual framework of artificial intelligence technologies for the management of innovation,” IEEE Trans. Eng. Manage., vol. 70, no. 2, pp. 770–776, 2021. doi: 10.1109/TEM.2021.3109983. [Google Scholar] [CrossRef]
32. A. Chandratreya, “AI-powered innovations in electrical engineering: Enhancing efficiency, reliability, and sustainability,” J. Elec. Syst., vol. 20, no. 2, pp. 1580–1587, 2024. doi: 10.52783/jes.1463. [Google Scholar] [CrossRef]
33. Y. Liu, J. Sun, Z. Zhang, M. Wu, H. Sima and Y. M. Ooi, “How AI impacts companies’ dynamic capabilities: Lessons from six Chinese construction firms,” Res. Technol. Manage., vol. 67, no. 3, pp. 64–76, 2024. doi: 10.1080/08956308.2024.2324407. [Google Scholar] [CrossRef]
34. S. F. Wamba, M. M. Queiroz, and L. Trinchera, “The role of artificial intelligence-enabled dynamic capability on environmental performance: The mediation effect of a data-driven culture in France and the USA,” Int. J. Prod. Econ., vol. 268, 2024, Art. no. 109131. doi: 10.1016/j.ijpe.2023.109131. [Google Scholar] [CrossRef]
35. S. Verma and V. Singh, “Impact of artificial intelligence-enabled job characteristics and perceived substitution crisis on innovative work behavior of employees from high-tech firms,” Comput. Human Behav., vol. 131, 2022, Art. no. 107215. doi: 10.1016/j.chb.2022.107215. [Google Scholar] [CrossRef]
36. F. Xing, G. Peng, B. Zhang, S. Zuo, J. Tang and S. Li, “Driving innovation with the application of industrial AI in the R&D domain,” in Distributed, Ambient and Pervasive Interactions. Cham: Springer, 2020, vol. 12203, pp. 244–255. [Google Scholar]
37. M. de la Paz-Marín, P. Campoy-Muñoz, and C. Hervás-Martínez, “Non-linear multiclassifier model based on Artificial Intelligence to predict research and development performance in European countries,” Technol. Forecast. Soc. Change, vol. 79, no. 9, pp. 1731–1745, 2012. doi: 10.1016/j.techfore.2012.06.001. [Google Scholar] [CrossRef]
38. C. Krishnamoorthy and S. Rajeev, Artificial Intelligence and Expert Systems for Engineers. Boca Raton: CRC Press, 2018. [Google Scholar]
39. S. S. Cheema, L. Chand, and B. Singh, “Artificial Intelligence in machine learning,” in Factories of the Future: Technological Advancements in the Manufacturing Industry. 2023, pp. 161–194. doi: 10.1002/9781119865216.ch8. [Google Scholar] [CrossRef]
40. T. Marwala, “Artificial intelligence techniques for rational decision making,” in Advanced Information and Knowledge Processing. Cham: Springer, 2014. [Google Scholar]
41. K. Flanagan, B. Ribeiro, and P. Ferri, AI and Scientific Productivity: Considering Policy and Governance Challenges. Manchester: The University of Manchester, 2023. [Google Scholar]
42. R. Mishra, Artificial Intelligence. Delhi, India: PHI, 2010. [Google Scholar]
43. J. Liu, H. Chang, J. Y. -L. Forrest, and B. Yang, “Influence of artificial intelligence on technological innovation: Evidence from the panel data of China’s manufacturing sectors,” Technol. Forecast. Soc. Change, vol. 158, 2020, Art. no. 120142. doi: 10.1016/j.techfore.2020.120142. [Google Scholar] [CrossRef]
44. J. Bughin et al., Artificial Intelligence the Next Digital Frontier? McKinsey & Company, 2017. Accessed: Sep. 1, 2024. [Online]. Available: http://dln.jaipuria.ac.in:8080/jspui/bitstream/123456789/14268/1/MGI-artificial-intelligence-discussion-paper.pdf [Google Scholar]
45. J. Jumper et al., “Highly accurate protein structure prediction with AlphaFold,” Nature, vol. 596, no. 7873, pp. 583–589, 2021. [Google Scholar] [PubMed]
46. K. T. Butler, D. W. Davies, H. Cartwright, O. Isayev, and A. Walsh, “Machine learning for molecular and materials science,” Nature, vol. 559, no. 7715, pp. 547–555, 2018. doi: 10.1038/s41586-018-0337-2. [Google Scholar] [PubMed] [CrossRef]
47. Z. Qiu, H. Zhao, and S. Wang, “Applications and challenges of Artificial Intelligence in Aerospace Engineering,” in 2023 6th Int. Conf. Artif. Intell. Big Data (ICAIBD), Chengdu, China, 2023, pp. 970–974. [Google Scholar]
48. S. L. Brunton et al., “Data-driven aerospace engineering: Reframing the industry with machine learning,” AIAA J., vol. 59, no. 8, pp. 2820–2847, 2021. doi: 10.2514/1.J060131. [Google Scholar] [CrossRef]
49. S. Le Clainche, E. Ferrer, S. Gibson, E. Cross, A. Parente and R. Vinuesa, “Improving aircraft performance using machine learning: A review,” Aerosp. Sci. Technol., vol. 138, 2023, Art. no. 108354. doi: 10.1016/j.ast.2023.108354. [Google Scholar] [CrossRef]
50. R. Binns, “Fairness in machine learning: Lessons from political philosophy,” in Proc. 1st Conf. Fairness, Account. Transp., 2018, pp. 149–159. [Google Scholar]
51. Y. K. Dwivedi, A. Sharma, N. P. Rana, M. Giannakis, P. Goel and V. Dutot, “Evolution of artificial intelligence research in technological forecasting and social change: Research topics, trends, and future directions,” Technol. Forecast. Soc. Change, vol. 192, 2023, Art. no. 122579. doi: 10.1016/j.techfore.2023.122579. [Google Scholar] [CrossRef]
52. J. Just, “Natural language processing for innovation search–Reviewing an emerging non-human innovation intermediary,” Technovation, vol. 129, 2024, Art. no. 102883. doi: 10.1016/j.technovation.2023.102883. [Google Scholar] [CrossRef]
53. A. Zhavoronkov, “Artificial intelligence for drug discovery, biomarker development, and generation of novel chemistry,” Mol. Pharm., vol. 15, no. 10, pp. 4311–4313, 2018. [Google Scholar]
54. S. Thrun, “Toward robotic cars,” Commun. ACM, vol. 53, no. 4, pp. 99–106, 2010. doi: 10.1145/1721654.1721679. [Google Scholar] [CrossRef]
55. S. J. Russell and P. Norvig, Artificial Intelligence: A Modern Approach. London, UK: Pearson, 2016. [Google Scholar]
56. Y. Qin, Z. Xu, X. Wang, and M. Skare, “Artificial intelligence and economic development: An evolutionary investigation and systematic review,” J. Knowl. Econ., vol. 15, no. 1, pp. 1736–1770, 2024. doi: 10.1007/s13132-023-01183-2. [Google Scholar] [CrossRef]
57. R. Vinuesa et al., “The role of artificial intelligence in achieving the sustainable development goals,” Nat. Commun., vol. 11, no. 1, pp. 1–10, 2020. doi: 10.1038/s41467-019-14108-y. [Google Scholar] [PubMed] [CrossRef]
58. M. -H. Huang and R. T. Rust, “A framework for collaborative artificial intelligence in marketing,” J. Retailing, vol. 98, no. 2, pp. 209–223, 2022. doi: 10.1016/j.jretai.2021.03.001. [Google Scholar] [CrossRef]
59. P. Mikalef and M. Gupta, “Artificial intelligence capability: Conceptualization, measurement calibration, and empirical study on its impact on organizational creativity and firm performance,” Inform. Manage., vol. 58, no. 3, 2021, Art. no. 103434. doi: 10.1016/j.im.2021.103434. [Google Scholar] [CrossRef]
60. P. Radanliev, “Artificial intelligence: Reflecting on the past and looking towards the next paradigm shift,” J. Exp. Theor. Artif. Intell., pp. 1–18, 2024. doi: 10.1080/0952813X.2024.2323042. [Google Scholar] [CrossRef]
61. A. Sheth and K. Thirunarayan, “The duality of data and knowledge across the three waves of AI,” IT Prof., vol. 23, no. 3, pp. 35–45, 2021. doi: 10.1109/MITP.2021.3070985. [Google Scholar] [CrossRef]
62. E. Hermann, G. Hermann, and J. C. Tremblay, “Ethical Artificial Intelligence in chemical research and development: A dual advantage for sustainability,” Sci. Eng. Ethics, vol. 27, no. 4, 2021, Art. no. 45. doi: 10.1007/s11948-021-00325-6. [Google Scholar] [PubMed] [CrossRef]
63. O. Liu, J. Wang, J. Ma, and Y. Sun, “An intelligent decision support approach for reviewer assignment in R&D project selection,” Comput. Ind., vol. 76, pp. 1–10, 2016. doi: 10.1016/j.compind.2015.11.001. [Google Scholar] [CrossRef]
64. K. Lee, Y. Jeong, and B. Yoon, “Developing an research and development (R&D) process improvement system to simulate the performance of R&D activities,” Comput. Ind., vol. 92-93, pp. 178–193, 2017. doi: 10.1016/j.compind.2017.08.001. [Google Scholar] [CrossRef]
65. S. R. Havins, “Decision support systems for managing innovation through project selection in public sector R&D environments,” IEEE Eng. Manage. Rev., vol. 48, no. 4, pp. 28–31, 2020. doi: 10.1109/EMR.2020.3007748. [Google Scholar] [CrossRef]
66. H. Jang, “A decision support framework for robust R&D budget allocation using machine learning and optimization,” Decis. Support Syst., vol. 121, pp. 1–12, 2019. doi: 10.1016/j.dss.2019.03.010. [Google Scholar] [CrossRef]
67. J. Wang, N. Yang, Y. Zhang, and Y. Song, “Development of the mitigation strategy against the schedule risks of the R&D project through controlling the cascading failure of the R&D network,” Physica A: Stat. Mech. Appl., vol. 508, pp. 390–401, 2018. doi: 10.1016/j.physa.2018.05.108. [Google Scholar] [CrossRef]
68. X. Zhang and C. Liu, “Research on innovation knowledge spillover effect of China’s high-tech industry R&D-base on multidimensional spatial weight matrices,” J. Adv. Comput. Intell. Intell. Informat., vol. 22, no. 4, pp. 437–447, 2018. doi: 10.20965/jaciii.2018.p0437. [Google Scholar] [CrossRef]
69. G. Villani, “A neural network approach to value R&D compound american exchange option,” Comput. Econ., vol. 60, no. 1, pp. 305–324, 2022. doi: 10.1007/s10614-021-10150-5. [Google Scholar] [CrossRef]
70. H. Geerts and J. E. Barrett, “Neuronal circuit-based computer modeling as a phenotypic strategy for CNS R&D,” Front. Neurosci., vol. 13, 2019, Art. no. 723. doi: 10.3389/fnins.2019.00723. [Google Scholar] [PubMed] [CrossRef]
71. F. Graf, C. Amon, M. Blass, M. Fišer, F. Fuhrmann and F. Krebs, “Let’s teach computers to listen: 20 years of AI in acoustic research and development at JOANNEUM RESEARCH,” Elektrotechnik Und Informationstechnik, vol. 138, no. 3, pp. 139–147, 2021. doi: 10.1007/s00502-021-00882-5. [Google Scholar] [CrossRef]
72. W. Zhou and H. Li, “R&D team network configurations, knowledge diversity and breakthrough innovation: A combined effect framework,” Eur. J. Innov. Manage., 2024. doi: 10.1108/EJIM-11-2023-1004. [Google Scholar] [CrossRef]
73. P. Guo, D. Wang, and N. Guo, “Evolution of cooperation in R&D alliance portfolios considering aspirations,” Manage. Decis., 2024. doi: 10.1108/MD-06-2023-0978. [Google Scholar] [CrossRef]
74. C. Keding and P. Meissner, “Managerial overreliance on AI-augmented decision-making processes: How the use of AI-based advisory systems shapes choice behavior in R&D investment decisions,” Technol. Forecast. Soc. Change, vol. 171, 2021, Art. no. 120970. doi: 10.1016/j.techfore.2021.120970. [Google Scholar] [CrossRef]
75. D. Viberg and M. H. Eslami, “The effect of machine learning on knowledge-intensive R&D in the technology industry,” Technol. Innov. Manage. Rev., vol. 10, no. 3, pp. 87–97, 2020. [Google Scholar]
76. J. M. Liao, M. J. Chang, and L. M. Chang, “Prediction of air-conditioning energy consumption in R&D building using multiple machine learning techniques,” Energies, vol. 13, no. 7, 2020, Art. no. 1847. doi: 10.3390/en13071847. [Google Scholar] [CrossRef]
77. B. Lutnick et al., “Accelerating pharmaceutical R&D with a user-friendly AI system for histopathology image analysis,” J. Pathol. Inf., vol. 14, 2023, Art. no. 100337. doi: 10.1016/j.jpi.2023.100337. [Google Scholar] [PubMed] [CrossRef]
78. X. Chen et al., “Application and teaching of computer molecular simulation embedded technology and artificial intelligence in drug research and development,” Open Life Sci., vol. 18, no. 1, 2023. doi: 10.1515/biol-2022-0675. [Google Scholar] [PubMed] [CrossRef]
79. J. D. Piette et al., “Patient-centered pain care using artificial intelligence and mobile health tools: Protocol for a randomized study funded by the US Department of Veterans Affairs Health Services Research and Development Program,” JMIR Res. Protoc., vol. 5, no. 2, 2016, Art. no. e53. doi: 10.2196/resprot.4995. [Google Scholar] [PubMed] [CrossRef]
80. S. P. Jun, H. S. Yoo, and J. Hwang, “A hybrid recommendation model for successful R&D collaboration: Mixing machine learning and discriminant analysis,” Technol. Forecast. Soc. Change, vol. 170, 2021, Art. no. 120871. doi: 10.1016/j.techfore.2021.120871. [Google Scholar] [CrossRef]
81. H. Li, B. Yao, and X. Yan, “Data-driven public R&D project performance evaluation: Results from China,” Sustainability, vol. 13, no. 13, 2021, Art. no. 7147. doi: 10.3390/su13137147. [Google Scholar] [CrossRef]
82. N. Chukhray, N. Shakhovska, O. Mrykhina, L. Lisovska, and I. Izonin, “Stacking machine learning model for the assessment of R&D product’s readiness and method for its cost estimation,” Mathematics, vol. 10, no. 9, 2022, Art. no. 1466. doi: 10.3390/math10091466. [Google Scholar] [CrossRef]
83. Z. Tafferner, B. Illés, O. Krammer, and A. Géczy, “Can ChatGPT help in electronics research and development? A case study with applied sensors,” Sensors, vol. 23, no. 10, 2023, Art. no. 4879. doi: 10.3390/s23104879. [Google Scholar] [PubMed] [CrossRef]
84. S. Kocak, Y. T. Ic, M. Sert, K. D. Atalay, and B. Dengiz, “Development of a decision support system for selection of reviewers to evaluate research and development projects,” Int. J. Inf. Technol. Decis. Mak., vol. 22, no. 6, pp. 1991–2020, 2023. doi: 10.1142/S0219622022500961. [Google Scholar] [CrossRef]
85. Y. Huang et al., “HiRAND: A novel GCN semi-supervised deep learning-based framework for classification and feature selection in drug research and development,” Front. Oncol., vol. 13, pp. 13–24, 2023. doi: 10.36366/frontiers.v3i1.38. [Google Scholar] [CrossRef]
86. D. P. Gutierrez, L. M. Folkmann, H. Tribukait, and L. M. Roch, “How to accelerate R&D and optimize experiment planning with machine learning and data science,” Chimia, vol. 77, no. 1–2, pp. 7–16, 2023. doi: 10.2533/chimia.2023.7. [Google Scholar] [PubMed] [CrossRef]
87. S. Takemoto and Y. Okuno, “How to lead R&D digital transformation in a chemical corporation,” MRS Adv., vol. 8, no. 7, pp. 416–421, 2023. doi: 10.1557/s43580-023-00505-5. [Google Scholar] [CrossRef]
88. J. Jung, J. Lee, S. Choi, and W. Baek, “Information analysis on foreign institution for international R&D collaboration using natural language processing,” Energies, vol. 16, no. 1, 2023, Art. no. 33. doi: 10.3390/en16010033. [Google Scholar] [CrossRef]
89. M. Behr, R. Burghaus, C. Diedrich, and J. Lippert, “Opportunities and challenges for AI-based analysis of RWD in pharmaceutical R&D: A practical perspective,” Künstl. Intell., 2023. doi: 10.1007/s13218-023-00809-6. [Google Scholar] [CrossRef]
90. H. R. Ma, J. H. Lee, and C. J. Lee, “Predicting low-performance government-sponsored R&D project: Comprehensive framework and machine learning approach,” Technol. Anal. Strateg. Manage., vol. 25, pp. 1–14, 2023. doi: 10.1080/09537325.2023.2264961. [Google Scholar] [CrossRef]
91. H. S. Yoo, Y. L. Jung, and S. P. Jun, “Prediction of SMEs’ R&D performances by machine learning for project selection,” Sci. Rep., vol. 13, no. 1, 2023, Art. no. 7598. doi: 10.1038/s41598-023-34684-w. [Google Scholar] [PubMed] [CrossRef]
92. J. Lee and H. B. Kwon, “Synergistic effect of R&D and exports on performance in US manufacturing industries: High-tech vs low-tech,” J. Model. Manage., vol. 18, no. 2, pp. 343–371, 2023. doi: 10.1108/JM2-03-2021-0057. [Google Scholar] [CrossRef]
93. A. Sterlacchini, “AI patenting and employment: Evidence from the world’s top R&D investors,” Innovation: The Eur. J. Soc. Sci. Res., 2024. doi: 10.1080/13511610.2024.2339943. [Google Scholar] [CrossRef]
94. X. Wang, S. Lu, X. Qian, C. Hu, and X. Liu, “Dynamic scheduling of decentralized high-end equipment R&D projects via deep reinforcement learning,” Comput. Ind. Eng., vol. 190, 2024, Art. no. 110018. doi: 10.1016/j.cie.2024.110018. [Google Scholar] [CrossRef]
95. T. Besiroglu, N. Emery-Xu, and N. Thompson, “Economic impacts of AI-augmented R&D,” Res. Policy, vol. 53, no. 7, 2024, Art. no. 105037. doi: 10.1016/j.respol.2024.105037. [Google Scholar] [CrossRef]
96. A. Jo, “The intricate dance of knowledge, innovation, and AI: Navigating the human element,” J. Knowl. Learn. Sci. Technol., vol. 2, no. 3, pp. 392–400, 2023. doi: 10.60087/jklst.vol2.n3.p400. [Google Scholar] [CrossRef]
97. R. Alur, M. Raghavan, and D. Shah, “Distinguishing the indistinguishable: Human expertise in algorithmic prediction,” 2024, arXiv:2402.00793. [Google Scholar]
98. K. Venigandla, N. Vemuri, and N. Vemuri, “Hybrid intelligence systems combining human expertise and AI/RPA for complex problem solving,” Int. J. Innov. Sci. Res. Technol., vol. 9, no. 3, pp. 2066–2075, 2024. doi: 10.38124/IJISRT. [Google Scholar] [CrossRef]
99. A. M. Al-Zahrani, “Balancing act: Exploring the interplay between human judgment and Artificial Intelligence in problem-solving, creativity, and decision-making,” IgMin Res., vol. 2, no. 3, pp. 145–158, 2024. doi: 10.61927/igmin158. [Google Scholar] [CrossRef]
100. I. G. Cohen, B. Babic, S. Gerke, Q. Xia, T. Evgeniou and K. Wertenbroch, “How AI can learn from the law: Putting humans in the loop only on appeal,” npj Digital Med., vol. 6, no. 1, 2023, Art. no. 160. doi: 10.1038/s41746-023-00906-8. [Google Scholar] [PubMed] [CrossRef]
Cite This Article
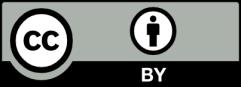
This work is licensed under a Creative Commons Attribution 4.0 International License , which permits unrestricted use, distribution, and reproduction in any medium, provided the original work is properly cited.