Open Access
ARTICLE
An Improved Distraction Behavior Detection Algorithm Based on YOLOv5
College of Information Engineering, Henan University of Science and Technology, Luoyang, 471023, China
* Corresponding Author: Guoqiang Zheng. Email:
(This article belongs to the Special Issue: Research on Deep Learning-based Object Detection and Its Derivative Key Technologies)
Computers, Materials & Continua 2024, 81(2), 2571-2585. https://doi.org/10.32604/cmc.2024.056863
Received 01 August 2024; Accepted 26 September 2024; Issue published 18 November 2024
Abstract
Distracted driving remains a primary factor in traffic accidents and poses a significant obstacle to advancing driver assistance technologies. Improving the accuracy of distracted driving can greatly reduce the occurrence of traffic accidents, thereby providing a guarantee for the safety of drivers. However, detecting distracted driving behaviors remains challenging in real-world scenarios with complex backgrounds, varying target scales, and different resolutions. Addressing the low detection accuracy of existing vehicle distraction detection algorithms and considering practical application scenarios, this paper proposes an improved vehicle distraction detection algorithm based on YOLOv5. The algorithm integrates Attention-based Intra-scale Feature Interaction (AIFI) into the backbone network, enabling it to focus on enhancing feature interactions within the same scale through the attention mechanism. By emphasizing important features, this approach improves detection accuracy, thereby enhancing performance in complex backgrounds. Additionally, a Triple Feature Encoding (TFE) module has been added to the neck network. This module utilizes multi-scale features, encoding and fusing them to create a more detailed and comprehensive feature representation, enhancing object detection and localization, and enabling the algorithm to fully understand the image. Finally, the shape-IoU (Intersection over Union) loss function is adopted to replace the original IoU for more precise bounding box regression. Comparative evaluation of the improved YOLOv5 distraction detection algorithm against the original YOLOv5 algorithm shows an average accuracy improvement of 1.8%, indicating significant advantages in solving distracted driving problems.Keywords
Cite This Article
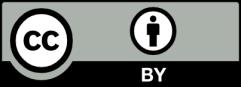
This work is licensed under a Creative Commons Attribution 4.0 International License , which permits unrestricted use, distribution, and reproduction in any medium, provided the original work is properly cited.