Open Access
ARTICLE
Enhancing Solar Energy Production Forecasting Using Advanced Machine Learning and Deep Learning Techniques: A Comprehensive Study on the Impact of Meteorological Data
1 Instutute of Computer Science and Information Technologies, Lviv Polytechnic National University, Lviv, 79013, Ukraine
2 Department of Applied Mathematics, University of Agriculture in Krakow, Krakow, 31-120, Poland
3 Blackthorn AI, Ltd., London, EC1V 2NX, UK
* Corresponding Author: Nataliya Shakhovska. Email:
(This article belongs to the Special Issue: Artificial Neural Networks and its Applications)
Computers, Materials & Continua 2024, 81(2), 3147-3163. https://doi.org/10.32604/cmc.2024.056542
Received 24 July 2024; Accepted 23 October 2024; Issue published 18 November 2024
Abstract
The increasing adoption of solar photovoltaic systems necessitates accurate forecasting of solar energy production to enhance grid stability, reliability, and economic benefits. This study explores advanced machine learning (ML) and deep learning (DL) techniques for predicting solar energy generation, emphasizing the significant impact of meteorological data. A comprehensive dataset, encompassing detailed weather conditions and solar energy metrics, was collected and preprocessed to improve model accuracy. Various models were developed and trained with different preprocessing stages. Finally, three datasets were prepared. A novel hour-based prediction wrapper was introduced, utilizing external sunrise and sunset data to restrict predictions to daylight hours, thereby enhancing model performance. A cascaded stacking model incorporating association rules, weak predictors, and a modified stacking aggregation procedure was proposed, demonstrating enhanced generalization and reduced prediction errors. Results indicated that models trained on raw data generally performed better than those on stripped data. The Long Short-Term Memory (LSTM) with Inception layers’ model was the most effective, achieving significant performance improvements through feature selection, data preprocessing, and innovative modeling techniques. The study underscores the potential to combine detailed meteorological data with advanced ML and DL methods to improve the accuracy of solar energy forecasting, thereby optimizing energy management and planning.Keywords
Cite This Article
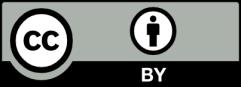
This work is licensed under a Creative Commons Attribution 4.0 International License , which permits unrestricted use, distribution, and reproduction in any medium, provided the original work is properly cited.