Open Access
ARTICLE
Position-Aware and Subgraph Enhanced Dynamic Graph Contrastive Learning on Discrete-Time Dynamic Graph
College of Computer Science & Technology, Xi’an University of Science and Technology, Xi’an, 710054, China
* Corresponding Author: Jian Feng. Email:
Computers, Materials & Continua 2024, 81(2), 2895-2909. https://doi.org/10.32604/cmc.2024.056434
Received 23 July 2024; Accepted 10 October 2024; Issue published 18 November 2024
Abstract
Unsupervised learning methods such as graph contrastive learning have been used for dynamic graph representation learning to eliminate the dependence of labels. However, existing studies neglect positional information when learning discrete snapshots, resulting in insufficient network topology learning. At the same time, due to the lack of appropriate data augmentation methods, it is difficult to capture the evolving patterns of the network effectively. To address the above problems, a position-aware and subgraph enhanced dynamic graph contrastive learning method is proposed for discrete-time dynamic graphs. Firstly, the global snapshot is built based on the historical snapshots to express the stable pattern of the dynamic graph, and the random walk is used to obtain the position representation by learning the positional information of the nodes. Secondly, a new data augmentation method is carried out from the perspectives of short-term changes and long-term stable structures of dynamic graphs. Specifically, subgraph sampling based on snapshots and global snapshots is used to obtain two structural augmentation views, and node structures and evolving patterns are learned by combining graph neural network, gated recurrent unit, and attention mechanism. Finally, the quality of node representation is improved by combining the contrastive learning between different structural augmentation views and between the two representations of structure and position. Experimental results on four real datasets show that the performance of the proposed method is better than the existing unsupervised methods, and it is more competitive than the supervised learning method under a semi-supervised setting.Keywords
Cite This Article
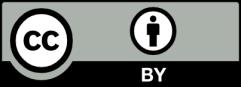
This work is licensed under a Creative Commons Attribution 4.0 International License , which permits unrestricted use, distribution, and reproduction in any medium, provided the original work is properly cited.