Open Access
ARTICLE
YOLO-VSI: An Improved YOLOv8 Model for Detecting Railway Turnouts Defects in Complex Environments
School of Computer Science and Technology, Zhejiang Sci-Tech University, Hangzhou, 310018, China
* Corresponding Author: Zhilong Lu. Email:
Computers, Materials & Continua 2024, 81(2), 3261-3280. https://doi.org/10.32604/cmc.2024.056413
Received 22 July 2024; Accepted 24 September 2024; Issue published 18 November 2024
Abstract
Railway turnouts often develop defects such as chipping, cracks, and wear during use. If not detected and addressed promptly, these defects can pose significant risks to train operation safety and passenger security. Despite advances in defect detection technologies, research specifically targeting railway turnout defects remains limited. To address this gap, we collected images from railway inspectors and constructed a dataset of railway turnout defects in complex environments. To enhance detection accuracy, we propose an improved YOLOv8 model named YOLO-VSS-SOUP-Inner-CIoU (YOLO-VSI). The model employs a state-space model (SSM) to enhance the C2f module in the YOLOv8 backbone, proposed the C2f-VSS module to better capture long-range dependencies and contextual features, thus improving feature extraction in complex environments. In the network’s neck layer, we integrate SPDConv and Omni-Kernel Network (OKM) modules to improve the original PAFPN (Path Aggregation Feature Pyramid Network) structure, and proposed the Small Object Upgrade Pyramid (SOUP) structure to enhance small object detection capabilities. Additionally, the Inner-CIoU loss function with a scale factor is applied to further enhance the model’s detection capabilities. Compared to the baseline model, YOLO-VSI demonstrates a 3.5% improvement in average precision on our railway turnout dataset, showcasing increased accuracy and robustness. Experiments on the public NEU-DET dataset reveal a 2.3% increase in average precision over the baseline, indicating that YOLO-VSI has good generalization capabilities.Keywords
Cite This Article
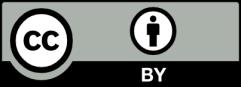
This work is licensed under a Creative Commons Attribution 4.0 International License , which permits unrestricted use, distribution, and reproduction in any medium, provided the original work is properly cited.