Open Access
ARTICLE
HGNN-ETC: Higher-Order Graph Neural Network Based on Chronological Relationships for Encrypted Traffic Classification
Key Laboratory of Aerospace Information Security and Trusted Computing, Ministry of Education, School of Cyber Science and Engineering, Wuhan University, Wuhan, 430072, China
* Corresponding Author: Xiya Guo. Email:
Computers, Materials & Continua 2024, 81(2), 2643-2664. https://doi.org/10.32604/cmc.2024.056165
Received 15 July 2024; Accepted 27 September 2024; Issue published 18 November 2024
Abstract
Encrypted traffic plays a crucial role in safeguarding network security and user privacy. However, encrypting malicious traffic can lead to numerous security issues, making the effective classification of encrypted traffic essential. Existing methods for detecting encrypted traffic face two significant challenges. First, relying solely on the original byte information for classification fails to leverage the rich temporal relationships within network traffic. Second, machine learning and convolutional neural network methods lack sufficient network expression capabilities, hindering the full exploration of traffic’s potential characteristics. To address these limitations, this study introduces a traffic classification method that utilizes time relationships and a higher-order graph neural network, termed HGNN-ETC. This approach fully exploits the original byte information and chronological relationships of traffic packets, transforming traffic data into a graph structure to provide the model with more comprehensive context information. HGNN-ETC employs an innovative k-dimensional graph neural network to effectively capture the multi-scale structural features of traffic graphs, enabling more accurate classification. We select the ISCXVPN and the USTC-TK2016 dataset for our experiments. The results show that compared with other state-of-the-art methods, our method can obtain a better classification effect on different datasets, and the accuracy rate is about 97.00%. In addition, by analyzing the impact of varying input specifications on classification performance, we determine the optimal network data truncation strategy and confirm the model’s excellent generalization ability on different datasets.Keywords
Cite This Article
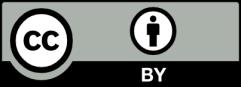
This work is licensed under a Creative Commons Attribution 4.0 International License , which permits unrestricted use, distribution, and reproduction in any medium, provided the original work is properly cited.