Open Access
REVIEW
A Review of Generative Adversarial Networks for Intrusion Detection Systems: Advances, Challenges, and Future Directions
Information Systems Department, King Saud University, Riyadh, 11495, Saudi Arabia
* Corresponding Author: Monirah Al-Ajlan. Email:
Computers, Materials & Continua 2024, 81(2), 2053-2076. https://doi.org/10.32604/cmc.2024.055891
Received 09 July 2024; Accepted 16 October 2024; Issue published 18 November 2024
Abstract
The ever-growing network traffic threat landscape necessitates adopting accurate and robust intrusion detection systems (IDSs). IDSs have become a research hotspot and have seen remarkable performance improvements. Generative adversarial networks (GANs) have also garnered increasing research interest recently due to their remarkable ability to generate data. This paper investigates the application of (GANs) in (IDS) and explores their current use within this research field. We delve into the adoption of GANs within signature-based, anomaly-based, and hybrid IDSs, focusing on their objectives, methodologies, and advantages. Overall, GANs have been widely employed, mainly focused on solving the class imbalance issue by generating realistic attack samples. While GANs have shown significant potential in addressing the class imbalance issue, there are still open opportunities and challenges to be addressed. Little attention has been paid to their applicability in distributed and decentralized domains, such as IoT networks. Efficiency and scalability have been mostly overlooked, and thus, future works must aim at addressing these gaps.Keywords
Cite This Article
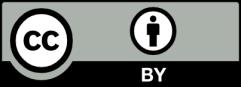
This work is licensed under a Creative Commons Attribution 4.0 International License , which permits unrestricted use, distribution, and reproduction in any medium, provided the original work is properly cited.