Open Access
ARTICLE
Privacy Preservation in IoT Devices by Detecting Obfuscated Malware Using Wide Residual Network
1 Applied College, Department of Computer Science and Information Technology, Princess Nourah bint Abdulrahman University, Riyadh, 11671, Saudi Arabia
2 College of Computer and Information Sciences, King Saud University, Riyadh, 11362, Saudi Arabia
3 College of Computer and Information Sciences, Prince Sultan University, Riyadh, 11586, Saudi Arabia
4 Department of Educational Technologies, College of Education, Imam Abdulrahman Bin Faisal University, Dammam, 31441, Saudi Arabia
* Corresponding Author: Syed Umar Amin. Email:
(This article belongs to the Special Issue: Privacy-Preserving Deep Learning and its Advanced Applications)
Computers, Materials & Continua 2024, 81(2), 2395-2436. https://doi.org/10.32604/cmc.2024.055469
Received 28 June 2024; Accepted 20 August 2024; Issue published 18 November 2024
Abstract
The widespread adoption of Internet of Things (IoT) devices has resulted in notable progress in different fields, improving operational effectiveness while also raising concerns about privacy due to their vulnerability to virus attacks. Further, the study suggests using an advanced approach that utilizes machine learning, specifically the Wide Residual Network (WRN), to identify hidden malware in IoT systems. The research intends to improve privacy protection by accurately identifying malicious software that undermines the security of IoT devices, using the MalMemAnalysis dataset. Moreover, thorough experimentation provides evidence for the effectiveness of the WRN-based strategy, resulting in exceptional performance measures such as accuracy, precision, F1-score, and recall. The study of the test data demonstrates highly impressive results, with a multiclass accuracy surpassing 99.97% and a binary class accuracy beyond 99.98%. The results emphasize the strength and dependability of using advanced deep learning methods such as WRN for identifying hidden malware risks in IoT environments. Furthermore, a comparison examination with the current body of literature emphasizes the originality and efficacy of the suggested methodology. This research builds upon previous studies that have investigated several machine learning methods for detecting malware on IoT devices. However, it distinguishes itself by showcasing exceptional performance metrics and validating its findings through thorough experimentation with real-world datasets. Utilizing WRN offers benefits in managing the intricacies of malware detection, emphasizing its capacity to enhance the security of IoT ecosystems. To summarize, this work proposes an effective way to address privacy concerns on IoT devices by utilizing advanced machine learning methods. The research provides useful insights into the changing landscape of IoT cybersecurity by emphasizing methodological rigor and conducting comparative performance analysis. Future research could focus on enhancing the recommended approach by adding more datasets and leveraging real-time monitoring capabilities to strengthen IoT devices’ defenses against new cybersecurity threats.Keywords
Cite This Article
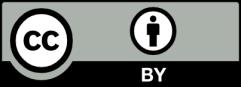
This work is licensed under a Creative Commons Attribution 4.0 International License , which permits unrestricted use, distribution, and reproduction in any medium, provided the original work is properly cited.