Open Access
ARTICLE
TLERAD: Transfer Learning for Enhanced Ransomware Attack Detection
School of Information Technology, Rajiv Gandhi Proudyogiki Vishwavidyalaya, Bhopal, 462033, India
* Corresponding Author: Isha Sood. Email:
Computers, Materials & Continua 2024, 81(2), 2791-2818. https://doi.org/10.32604/cmc.2024.055463
Received 27 June 2024; Accepted 05 September 2024; Issue published 18 November 2024
Abstract
Ransomware has emerged as a critical cybersecurity threat, characterized by its ability to encrypt user data or lock devices, demanding ransom for their release. Traditional ransomware detection methods face limitations due to their assumption of similar data distributions between training and testing phases, rendering them less effective against evolving ransomware families. This paper introduces TLERAD (Transfer Learning for Enhanced Ransomware Attack Detection), a novel approach that leverages unsupervised transfer learning and co-clustering techniques to bridge the gap between source and target domains, enabling robust detection of both known and unknown ransomware variants. The proposed method achieves high detection accuracy, with an AUC of 0.98 for known ransomware and 0.93 for unknown ransomware, significantly outperforming baseline methods. Comprehensive experiments demonstrate TLERAD’s effectiveness in real-world scenarios, highlighting its adaptability to the rapidly evolving ransomware landscape. The paper also discusses future directions for enhancing TLERAD, including real-time adaptation, integration with lightweight and post-quantum cryptography, and the incorporation of explainable AI techniques.Keywords
Cite This Article
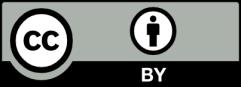
This work is licensed under a Creative Commons Attribution 4.0 International License , which permits unrestricted use, distribution, and reproduction in any medium, provided the original work is properly cited.