Open Access
ARTICLE
Two-Stage Client Selection Scheme for Blockchain-Enabled Federated Learning in IoT
1 Beijing Key Laboratory of Work Safety Intelligent Monitoring, School of Electronic Engineering, Beijing University of Posts and Telecommunications, Beijing, 100876, China
2 Institute of Industrial Internet and Internet of Things, China Academy of Information and Communications Technology, Beijing, 100191, China
* Corresponding Author: Chao Ma. Email:
(This article belongs to the Special Issue: Privacy-Preserving Deep Learning and its Advanced Applications)
Computers, Materials & Continua 2024, 81(2), 2317-2336. https://doi.org/10.32604/cmc.2024.055344
Received 24 June 2024; Accepted 15 August 2024; Issue published 18 November 2024
Abstract
Federated learning enables data owners in the Internet of Things (IoT) to collaborate in training models without sharing private data, creating new business opportunities for building a data market. However, in practical operation, there are still some problems with federated learning applications. Blockchain has the characteristics of decentralization, distribution, and security. The blockchain-enabled federated learning further improve the security and performance of model training, while also expanding the application scope of federated learning. Blockchain has natural financial attributes that help establish a federated learning data market. However, the data of federated learning tasks may be distributed across a large number of resource-constrained IoT devices, which have different computing, communication, and storage resources, and the data quality of each device may also vary. Therefore, how to effectively select the clients with the data required for federated learning task is a research hotspot. In this paper, a two-stage client selection scheme for blockchain-enabled federated learning is proposed, which first selects clients that satisfy federated learning task through attribute-based encryption, protecting the attribute privacy of clients. Then blockchain nodes select some clients for local model aggregation by proximal policy optimization algorithm. Experiments show that the model performance of our two-stage client selection scheme is higher than that of other client selection algorithms when some clients are offline and the data quality is poor.Keywords
Cite This Article
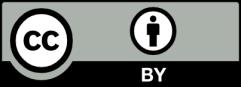
This work is licensed under a Creative Commons Attribution 4.0 International License , which permits unrestricted use, distribution, and reproduction in any medium, provided the original work is properly cited.