Open Access
ARTICLE
A Deep Learning Approach to Industrial Corrosion Detection
1 Department of Computer Engineering (CE), College of Computer Science and Information Technology (CCSIT), Imam Abdulrahman Bin Faisal University, Dammam, 31441, Saudi Arabia
2 Department of Computer Science (CS), College of Computer Science and Information Technology (CCSIT), Imam Abdulrahman Bin Faisal University, Dammam, 31441, Saudi Arabia
3 Department of Computer Information Systems (CIS), College of Computer Science and Information Technology (CCSIT), Imam Abdulrahman Bin Faisal University, Dammam, 31441, Saudi Arabia
* Corresponding Author: Atta Rahman. Email:
(This article belongs to the Special Issue: Industrial Big Data and Artificial Intelligence-Driven Intelligent Perception, Maintenance, and Decision Optimization in Industrial Systems)
Computers, Materials & Continua 2024, 81(2), 2587-2605. https://doi.org/10.32604/cmc.2024.055262
Received 21 June 2024; Accepted 06 September 2024; Issue published 18 November 2024
Abstract
The proposed study focuses on the critical issue of corrosion, which leads to significant economic losses and safety risks worldwide. A key area of emphasis is the accuracy of corrosion detection methods. While recent studies have made progress, a common challenge is the low accuracy of existing detection models. These models often struggle to reliably identify corrosion tendencies, which are crucial for minimizing industrial risks and optimizing resource use. The proposed study introduces an innovative approach that significantly improves the accuracy of corrosion detection using a convolutional neural network (CNN), as well as two pretrained models, namely YOLOv8 and EfficientNetB0. By leveraging advanced technologies and methodologies, we have achieved high accuracies in identifying and managing the hazards associated with corrosion across various industrial settings. This advancement not only supports the overarching goals of enhancing safety and efficiency, but also sets a new benchmark for future research in the field. The results demonstrate a significant improvement in the ability to detect and mitigate corrosion-related concerns, providing a more accurate and comprehensive solution for industries facing these challenges. Both CNN and EfficientNetB0 exhibited 100% accuracy, precision, recall, and F1-score, followed by YOLOv8 with respective metrics of 95%, 100%, 90%, and 94.74%. Our approach outperformed state-of-the-art with similar datasets and methodologies.Keywords
Cite This Article
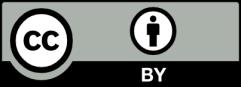
This work is licensed under a Creative Commons Attribution 4.0 International License , which permits unrestricted use, distribution, and reproduction in any medium, provided the original work is properly cited.