Open Access
ARTICLE
A Concise and Varied Visual Features-Based Image Captioning Model with Visual Selection
1 School of Computer Science and Engineering, Central South University, Changsha, 410083, China
2 Electronic Engineering and Information Science Department, University of Science and Technology of China, Hefei, 230026, China
3 EIAS Data Science Lab, College of Computer and Information Sciences, Prince Sultan University, Riyadh, 11586, Saudi Arabia
4 College of Computer and Information Sciences, Prince Sultan University, Riyadh, 11586, Saudi Arabia
5 College of Computer and Information Sciences, Imam Mohammad Ibn Saud Islamic University, Riyadh, 11432, Saudi Arabia
* Corresponding Author: Alaa Thobhani. Email:
Computers, Materials & Continua 2024, 81(2), 2873-2894. https://doi.org/10.32604/cmc.2024.054841
Received 09 June 2024; Accepted 29 September 2024; Issue published 18 November 2024
Abstract
Image captioning has gained increasing attention in recent years. Visual characteristics found in input images play a crucial role in generating high-quality captions. Prior studies have used visual attention mechanisms to dynamically focus on localized regions of the input image, improving the effectiveness of identifying relevant image regions at each step of caption generation. However, providing image captioning models with the capability of selecting the most relevant visual features from the input image and attending to them can significantly improve the utilization of these features. Consequently, this leads to enhanced captioning network performance. In light of this, we present an image captioning framework that efficiently exploits the extracted representations of the image. Our framework comprises three key components: the Visual Feature Detector module (VFD), the Visual Feature Visual Attention module (VFVA), and the language model. The VFD module is responsible for detecting a subset of the most pertinent features from the local visual features, creating an updated visual features matrix. Subsequently, the VFVA directs its attention to the visual features matrix generated by the VFD, resulting in an updated context vector employed by the language model to generate an informative description. Integrating the VFD and VFVA modules introduces an additional layer of processing for the visual features, thereby contributing to enhancing the image captioning model’s performance. Using the MS-COCO dataset, our experiments show that the proposed framework competes well with state-of-the-art methods, effectively leveraging visual representations to improve performance. The implementation code can be found here: (accessed on 30 July 2024).Keywords
Cite This Article
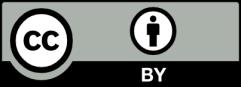
This work is licensed under a Creative Commons Attribution 4.0 International License , which permits unrestricted use, distribution, and reproduction in any medium, provided the original work is properly cited.