Open Access
ARTICLE
A Novel Hybrid Architecture for Superior IoT Threat Detection through Real IoT Environments
1 Department of Computer Science, Faculty of Computer Science and Information Technology, Jerash University, Jerash, 26150, Jordan
2 Computer Engineering Department, College of Engineering & Information Technology Buraydah Colleges, Buraydah, 51418, Saudi Arabia
3 Faculty of Architecture and Design, Al-Zaytoonah University of Jordan, Amman, 11733, Jordan
4 Department of Computer Science and Information Systems, College of Applied Sciences, AlMaarefa University, Diriyah, Riyadh, 13713, Saudi Arabia
5 Applied College, King Faisal University, Al-Ahsa, 31982, Saudi Arabia
6 Department of Business Intelligence and Data Analysis, Faculty of Financial Sciences and Business, Irbid National University, Irbid, 21110, Jordan
7 Faculty of Information Technology, Ajloun National University, Ajlun, 26810, Jordan
8 Department of Management Information Systems, College of Business Administration, King Faisal University, Al-Ahsa, 31982, Saudi Arabia
* Corresponding Author: Nisreen Innab. Email:
Computers, Materials & Continua 2024, 81(2), 2299-2316. https://doi.org/10.32604/cmc.2024.054836
Received 08 June 2024; Accepted 22 August 2024; Issue published 18 November 2024
Abstract
As the Internet of Things (IoT) continues to expand, incorporating a vast array of devices into a digital ecosystem also increases the risk of cyber threats, necessitating robust defense mechanisms. This paper presents an innovative hybrid deep learning architecture that excels at detecting IoT threats in real-world settings. Our proposed model combines Convolutional Neural Networks (CNN), Bidirectional Long Short-Term Memory (BLSTM), Gated Recurrent Units (GRU), and Attention mechanisms into a cohesive framework. This integrated structure aims to enhance the detection and classification of complex cyber threats while accommodating the operational constraints of diverse IoT systems. We evaluated our model using the RT-IoT2022 dataset, which includes various devices, standard operations, and simulated attacks. Our research’s significance lies in the comprehensive evaluation metrics, including Cohen Kappa and Matthews Correlation Coefficient (MCC), which underscore the model’s reliability and predictive quality. Our model surpassed traditional machine learning algorithms and the state-of-the-art, achieving over 99.6% precision, recall, F1-score, False Positive Rate (FPR), Detection Time, and accuracy, effectively identifying specific threats such as Message Queuing Telemetry Transport (MQTT) Publish, Denial of Service Synchronize network packet crafting tool (DOS SYN Hping), and Network Mapper Operating System Detection (NMAP OS DETECTION). The experimental analysis reveals a significant improvement over existing detection systems, significantly enhancing IoT security paradigms. Through our experimental analysis, we have demonstrated a remarkable enhancement in comparison to existing detection systems, which significantly strengthens the security standards of IoT. Our model effectively addresses the need for advanced, dependable, and adaptable security solutions, serving as a symbol of the power of deep learning in strengthening IoT ecosystems amidst the constantly evolving cyber threat landscape. This achievement marks a significant stride towards protecting the integrity of IoT infrastructure, ensuring operational resilience, and building privacy in this groundbreaking technology.Keywords
Cite This Article
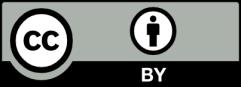
This work is licensed under a Creative Commons Attribution 4.0 International License , which permits unrestricted use, distribution, and reproduction in any medium, provided the original work is properly cited.