Open Access
ARTICLE
A Comprehensive Image Processing Framework for Early Diagnosis of Diabetic Retinopathy
1 College of Computer Science and Engineering, University of Ha’il, Ha’il, 81481, Saudi Arabia
2 Department of CSE, Chandigarh University, Mohali, Punjab, 140413, India
3 Department of Electronics and Communication Engineering, Amity University, Noida, 201301, India
4 Department of Information Technology, Indira Gandhi Delhi Technical University for Women, New Delhi, 110006, India
5 Chief Manager, State Bank of India, Panchkula, Haryana, 134109, India
6 Department of Computer Science and Information Engineering, Asia University, Taichung City, 413, Taiwan
7 Symbiosis Centre for Information Technology (SCIT), Symbiosis International University, Pune, 411057, India
8 Center for Interdisciplinary Research, University of Petroleum and Energy Studies (UPES), Dehradun, 248007, India
* Corresponding Author: Brij B. Gupta. Email:
(This article belongs to the Special Issue: Deep Learning in Medical Imaging-Disease Segmentation and Classification)
Computers, Materials & Continua 2024, 81(2), 2665-2683. https://doi.org/10.32604/cmc.2024.053565
Received 04 May 2024; Accepted 19 September 2024; Issue published 18 November 2024
Abstract
In today’s world, image processing techniques play a crucial role in the prognosis and diagnosis of various diseases due to the development of several precise and accurate methods for medical images. Automated analysis of medical images is essential for doctors, as manual investigation often leads to inter-observer variability. This research aims to enhance healthcare by enabling the early detection of diabetic retinopathy through an efficient image processing framework. The proposed hybridized method combines Modified Inertia Weight Particle Swarm Optimization (MIWPSO) and Fuzzy C-Means clustering (FCM) algorithms. Traditional FCM does not incorporate spatial neighborhood features, making it highly sensitive to noise, which significantly affects segmentation output. Our method incorporates a modified FCM that includes spatial functions in the fuzzy membership matrix to eliminate noise. The results demonstrate that the proposed FCM-MIWPSO method achieves highly precise and accurate medical image segmentation. Furthermore, segmented images are classified as benign or malignant using the Decision Tree-Based Temporal Association Rule (DT-TAR) Algorithm. Comparative analysis with existing state-of-the-art models indicates that the proposed FCM-MIWPSO segmentation technique achieves a remarkable accuracy of 98.42% on the dataset, highlighting its significant impact on improving diagnostic capabilities in medical imaging.Keywords
Cite This Article
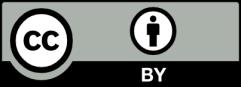
This work is licensed under a Creative Commons Attribution 4.0 International License , which permits unrestricted use, distribution, and reproduction in any medium, provided the original work is properly cited.