Open Access
ARTICLE
LQTTrack: Multi-Object Tracking by Focusing on Low-Quality Targets Association
1 Henan Key Laboratory of Big Data Analysis and Processing, School of Computer and Information Engineering, Henan University, Kaifeng, 475001, China
2 College of Information Science and Technology & Artificial Intelligence, Nanjing Forestry University, Nanjing, 210037, China
3 School of Computer, Nanjing University of Posts and Telecommunications, Nanjing, 210023, China
* Corresponding Author: Ying Cao. Email:
(This article belongs to the Special Issue: Machine Vision Detection and Intelligent Recognition, 2nd Edition)
Computers, Materials & Continua 2024, 81(1), 1449-1470. https://doi.org/10.32604/cmc.2024.056824
Received 31 July 2024; Accepted 18 September 2024; Issue published 15 October 2024
Abstract
Multi-object tracking (MOT) has seen rapid improvements in recent years. However, frequent occlusion remains a significant challenge in MOT, as it can cause targets to become smaller or disappear entirely, resulting in low-quality targets, leading to trajectory interruptions and reduced tracking performance. Different from some existing methods, which discarded the low-quality targets or ignored low-quality target attributes. LQTTrack, with a low-quality association strategy (LQA), is proposed to pay more attention to low-quality targets. In the association scheme of LQTTrack, firstly, multi-scale feature fusion of FPN (MSFF-FPN) is utilized to enrich the feature information and assist in subsequent data association. Secondly, the normalized Wasserstein distance (NWD) is integrated to replace the original Inter over Union (IoU), thus overcoming the limitations of the traditional IoU-based methods that are sensitive to low-quality targets with small sizes and enhancing the robustness of low-quality target tracking. Moreover, the third association stage is proposed to improve the matching between the current frame’s low-quality targets and previously interrupted trajectories from earlier frames to reduce the problem of track fragmentation or error tracking, thereby increasing the association success rate and improving overall multi-object tracking performance. Extensive experimental results demonstrate the competitive performance of LQTTrack on benchmark datasets (MOT17, MOT20, and DanceTrack).Keywords
Cite This Article
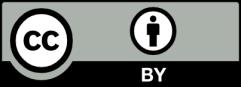
This work is licensed under a Creative Commons Attribution 4.0 International License , which permits unrestricted use, distribution, and reproduction in any medium, provided the original work is properly cited.