Open Access
ARTICLE
Human Interaction Recognition in Surveillance Videos Using Hybrid Deep Learning and Machine Learning Models
1 Department of ICT Convergence, Soonchunhyang University, Asan, 31538, Republic of Korea
2 ICT Convergence Research Center, Soonchunhyang University, Asan, 31538, Republic of Korea
3 Emotional and Intelligent Child Care Convergence Center, Soonchunhyang University, Asan, 31538, Republic of Korea
4 Department of Occupational Therapy, Soonchunhyang University, Asan, 31538, Republic of Korea
5 College of Hyangsul Nanum, Soonchunhyang University, Asan, 31538, Republic of Korea
* Corresponding Author: Yunyoung Nam. Email:
Computers, Materials & Continua 2024, 81(1), 773-787. https://doi.org/10.32604/cmc.2024.056767
Received 29 July 2024; Accepted 29 August 2024; Issue published 15 October 2024
Abstract
Human Interaction Recognition (HIR) was one of the challenging issues in computer vision research due to the involvement of multiple individuals and their mutual interactions within video frames generated from their movements. HIR requires more sophisticated analysis than Human Action Recognition (HAR) since HAR focuses solely on individual activities like walking or running, while HIR involves the interactions between people. This research aims to develop a robust system for recognizing five common human interactions, such as hugging, kicking, pushing, pointing, and no interaction, from video sequences using multiple cameras. In this study, a hybrid Deep Learning (DL) and Machine Learning (ML) model was employed to improve classification accuracy and generalizability. The dataset was collected in an indoor environment with four-channel cameras capturing the five types of interactions among 13 participants. The data was processed using a DL model with a fine-tuned ResNet (Residual Networks) architecture based on 2D Convolutional Neural Network (CNN) layers for feature extraction. Subsequently, machine learning models were trained and utilized for interaction classification using six commonly used ML algorithms, including SVM, KNN, RF, DT, NB, and XGBoost. The results demonstrate a high accuracy of 95.45% in classifying human interactions. The hybrid approach enabled effective learning, resulting in highly accurate performance across different interaction types. Future work will explore more complex scenarios involving multiple individuals based on the application of this architecture.Keywords
Cite This Article
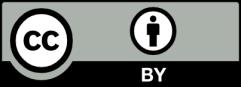
This work is licensed under a Creative Commons Attribution 4.0 International License , which permits unrestricted use, distribution, and reproduction in any medium, provided the original work is properly cited.