Open Access
ARTICLE
Mural Anomaly Region Detection Algorithm Based on Hyperspectral Multiscale Residual Attention Network
1 Key Laboratory of Spectral Imaging Technology CAS, Xi’an Institute of Optics and Precision Mechanics, Chinese Academy of Sciences, Xi’an, 710119, China
2 School of Optoelectronics, University of Chinese Academy of Sciences, Beijing, 100408, China
3 Institute of Culture and Heritage, Northwest Polytechnic University, Xi’an, 710072, China
* Corresponding Author: Shi Qiu. Email:
Computers, Materials & Continua 2024, 81(1), 1809-1833. https://doi.org/10.32604/cmc.2024.056706
Received 29 July 2024; Accepted 23 September 2024; Issue published 15 October 2024
Abstract
Mural paintings hold significant historical information and possess substantial artistic and cultural value. However, murals are inevitably damaged by natural environmental factors such as wind and sunlight, as well as by human activities. For this reason, the study of damaged areas is crucial for mural restoration. These damaged regions differ significantly from undamaged areas and can be considered abnormal targets. Traditional manual visual processing lacks strong characterization capabilities and is prone to omissions and false detections. Hyperspectral imaging can reflect the material properties more effectively than visual characterization methods. Thus, this study employs hyperspectral imaging to obtain mural information and proposes a mural anomaly detection algorithm based on a hyperspectral multi-scale residual attention network (HM-MRANet). The innovations of this paper include: (1) Constructing mural painting hyperspectral datasets. (2) Proposing a multi-scale residual spectral-spatial feature extraction module based on a 3D CNN (Convolutional Neural Networks) network to better capture multi-scale information and improve performance on small-sample hyperspectral datasets. (3) Proposing the Enhanced Residual Attention Module (ERAM) to address the feature redundancy problem, enhance the network’s feature discrimination ability, and further improve abnormal area detection accuracy. The experimental results show that the AUC (Area Under Curve), Specificity, and Accuracy of this paper’s algorithm reach 85.42%, 88.84%, and 87.65%, respectively, on this dataset. These results represent improvements of 3.07%, 1.11% and 2.68% compared to the SSRN algorithm, demonstrating the effectiveness of this method for mural anomaly detection.Keywords
Cite This Article
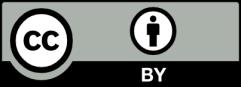
This work is licensed under a Creative Commons Attribution 4.0 International License , which permits unrestricted use, distribution, and reproduction in any medium, provided the original work is properly cited.