Open Access
ARTICLE
TGAIN: Geospatial Data Recovery Algorithm Based on GAIN-LSTM
1 School of Soft Engineering, Jinling Institute of Technology, Nanjing, 211169, China
2 School of Computer, Jinling Institute of Technology, Nanjing, 211169, China
3 School of Network Security, Jinling Institute of Technology, Nanjing, 211169, China
* Corresponding Author: Lechan Yang. Email:
Computers, Materials & Continua 2024, 81(1), 1471-1489. https://doi.org/10.32604/cmc.2024.056379
Received 21 July 2024; Accepted 14 September 2024; Issue published 15 October 2024
Abstract
Accurate geospatial data are essential for geographic information systems (GIS), environmental monitoring, and urban planning. The deep integration of the open Internet and geographic information technology has led to increasing challenges in the integrity and security of spatial data. In this paper, we consider abnormal spatial data as missing data and focus on abnormal spatial data recovery. Existing geospatial data recovery methods require complete datasets for training, resulting in time-consuming data recovery and lack of generalization. To address these issues, we propose a GAIN-LSTM-based geospatial data recovery method (TGAIN), which consists of two main works: (1) it uses a long-short-term recurrent neural network (LSTM) as a generator to analyze geospatial temporal data and capture its temporal correlation; (2) it constructs a complete TGAIN network using a cue-masked fusion matrix mechanism to obtain data that matches the original distribution of the input data. The experimental results on two publicly accessible datasets demonstrate that our proposed TGAIN approach surpasses four contemporary and traditional models in terms of mean absolute error (MAE), root mean square error (RMSE), mean square error (MSE), mean absolute percentage error (MAPE), coefficient of determination () and average computational time across various data missing rates. Concurrently, TGAIN exhibits superior accuracy and robustness in data recovery compared to existing models, especially when dealing with a high rate of missing data. Our model is of great significance in improving the integrity of geospatial data and provides data support for practical applications such as urban traffic optimization prediction and personal mobility analysis.Keywords
Cite This Article
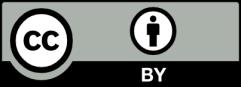
This work is licensed under a Creative Commons Attribution 4.0 International License , which permits unrestricted use, distribution, and reproduction in any medium, provided the original work is properly cited.