Open Access
ARTICLE
Improving Generalization for Hyperspectral Image Classification: The Impact of Disjoint Sampling on Deep Models
1 Department of Computer Science, National University of Computer and Emerging Sciences, Chiniot, 35400, Pakistan
2 Institute of Software Development and Engineering, Innopolis University, Innopolis, 420500, Russia
3 Dipartimento di Matematica e Informatica—MIFT, University of Messina, Messina, 98121, Italy
4 School of Computer Science, University of Hull, Hull, HU6 7RX, UK
5 Department of Geography, College of Humanities and Social Sciences, King Saud University, Riyadh, 11451, Saudi Arabia
* Corresponding Author: Muhammad Ahmad. Email:
Computers, Materials & Continua 2024, 81(1), 503-532. https://doi.org/10.32604/cmc.2024.056318
Received 11 July 2024; Accepted 11 August 2024; Issue published 15 October 2024
Abstract
Disjoint sampling is critical for rigorous and unbiased evaluation of state-of-the-art (SOTA) models e.g., Attention Graph and Vision Transformer. When training, validation, and test sets overlap or share data, it introduces a bias that inflates performance metrics and prevents accurate assessment of a model’s true ability to generalize to new examples. This paper presents an innovative disjoint sampling approach for training SOTA models for the Hyperspectral Image Classification (HSIC). By separating training, validation, and test data without overlap, the proposed method facilitates a fairer evaluation of how well a model can classify pixels it was not exposed to during training or validation. Experiments demonstrate the approach significantly improves a model’s generalization compared to alternatives that include training and validation data in test data (A trivial approach involves testing the model on the entire Hyperspectral dataset to generate the ground truth maps. This approach produces higher accuracy but ultimately results in low generalization performance). Disjoint sampling eliminates data leakage between sets and provides reliable metrics for benchmarking progress in HSIC. Disjoint sampling is critical for advancing SOTA models and their real-world application to large-scale land mapping with Hyperspectral sensors. Overall, with the disjoint test set, the performance of the deep models achieves 96.36% accuracy on Indian Pines data, 99.73% on Pavia University data, 98.29% on University of Houston data, 99.43% on Botswana data, and 99.88% on Salinas data.Keywords
Cite This Article
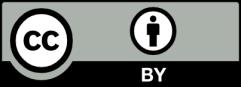
This work is licensed under a Creative Commons Attribution 4.0 International License , which permits unrestricted use, distribution, and reproduction in any medium, provided the original work is properly cited.