Open Access
ARTICLE
An Efficient Long Short-Term Memory and Gated Recurrent Unit Based Smart Vessel Trajectory Prediction Using Automatic Identification System Data
1 Department of Computer Engineering, Chungnam National University, Daejeon, 34134, Republic of Korea
2 Department of Environmental IT Engineering, Chungnam National University, Daejeon, 34134, Republic of Korea
3 Autonomous Ship Research Center, Samsung Heavy Industries, Daejeon, 34051, Republic of Korea
4 ICAROS Center (PRISMA Lab), University of Naples Federico II, Naples, 80131, Italy
5 Department of Computer Science, College of Computer Science and Information Technology, Jazan University, Jazan, 45142, Saudi Arabia
* Corresponding Author: Kyungsup Kim. Email:
Computers, Materials & Continua 2024, 81(1), 1789-1808. https://doi.org/10.32604/cmc.2024.056222
Received 17 July 2024; Accepted 20 September 2024; Issue published 15 October 2024
Abstract
Maritime transportation, a cornerstone of global trade, faces increasing safety challenges due to growing sea traffic volumes. This study proposes a novel approach to vessel trajectory prediction utilizing Automatic Identification System (AIS) data and advanced deep learning models, including Long Short-Term Memory (LSTM), Gated Recurrent Unit (GRU), Bidirectional LSTM (DBLSTM), Simple Recurrent Neural Network (SimpleRNN), and Kalman Filtering. The research implemented rigorous AIS data preprocessing, encompassing record deduplication, noise elimination, stationary simplification, and removal of insignificant trajectories. Models were trained using key navigational parameters: latitude, longitude, speed, and heading. Spatiotemporal aware processing through trajectory segmentation and topological data analysis (TDA) was employed to capture dynamic patterns. Validation using a three-month AIS dataset demonstrated significant improvements in prediction accuracy. The GRU model exhibited superior performance, achieving training losses of 0.0020 (Mean Squared Error, MSE) and 0.0334 (Mean Absolute Error, MAE), with validation losses of 0.0708 (MSE) and 0.1720 (MAE). The LSTM model showed comparable efficacy, with training losses of 0.0011 (MSE) and 0.0258 (MAE), and validation losses of 0.2290 (MSE) and 0.2652 (MAE). Both models demonstrated reductions in training and validation losses, measured by MAE, MSE, Average Displacement Error (ADE), and Final Displacement Error (FDE). This research underscores the potential of advanced deep learning models in enhancing maritime safety through more accurate trajectory predictions, contributing significantly to the development of robust, intelligent navigation systems for the maritime industry.Keywords
Cite This Article
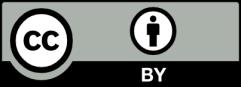
This work is licensed under a Creative Commons Attribution 4.0 International License , which permits unrestricted use, distribution, and reproduction in any medium, provided the original work is properly cited.