Open Access
ARTICLE
Integrating Ontology-Based Approaches with Deep Learning Models for Fine-Grained Sentiment Analysis
1 The Knowledge-Intensive Software Engineering (NiSE) Research Group, Department of Artificial Intelligence, Ajou University, Suwon City, 16499, Republic of Korea
2 Department of Software and Computer Engineering, Ajou University, Suwon City, 16499, Republic of Korea
* Corresponding Author: Seok-Won Lee. Email:
Computers, Materials & Continua 2024, 81(1), 1855-1877. https://doi.org/10.32604/cmc.2024.056215
Received 17 July 2024; Accepted 20 September 2024; Issue published 15 October 2024
Abstract
Although sentiment analysis is pivotal to understanding user preferences, existing models face significant challenges in handling context-dependent sentiments, sarcasm, and nuanced emotions. This study addresses these challenges by integrating ontology-based methods with deep learning models, thereby enhancing sentiment analysis accuracy in complex domains such as film reviews and restaurant feedback. The framework comprises explicit topic recognition, followed by implicit topic identification to mitigate topic interference in subsequent sentiment analysis. In the context of sentiment analysis, we develop an expanded sentiment lexicon based on domain-specific corpora by leveraging techniques such as word-frequency analysis and word embedding. Furthermore, we introduce a sentiment recognition method based on both ontology-derived sentiment features and sentiment lexicons. We evaluate the performance of our system using a dataset of 10,500 restaurant reviews, focusing on sentiment classification accuracy. The incorporation of specialized lexicons and ontology structures enables the framework to discern subtle sentiment variations and context-specific expressions, thereby improving the overall sentiment-analysis performance. Experimental results demonstrate that the integration of ontology-based methods and deep learning models significantly improves sentiment analysis accuracy.Keywords
Cite This Article
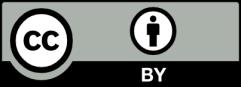
This work is licensed under a Creative Commons Attribution 4.0 International License , which permits unrestricted use, distribution, and reproduction in any medium, provided the original work is properly cited.