Open Access
ARTICLE
Adversarial Defense Technology for Small Infrared Targets
1 College of Automation Engineering, Nanjing University of Aeronautics and Astronautics, Nanjing, 210000, China
2 Shanghai Electro-Mechanical Engineering Institute, Shanghai, 201109, China
* Corresponding Author: Yali Xue. Email:
Computers, Materials & Continua 2024, 81(1), 1235-1250. https://doi.org/10.32604/cmc.2024.056075
Received 13 July 2024; Accepted 03 September 2024; Issue published 15 October 2024
Abstract
With the rapid development of deep learning-based detection algorithms, deep learning is widely used in the field of infrared small target detection. However, well-designed adversarial samples can fool human visual perception, directly causing a serious decline in the detection quality of the recognition model. In this paper, an adversarial defense technology for small infrared targets is proposed to improve model robustness. The adversarial samples with strong migration can not only improve the generalization of defense technology, but also save the training cost. Therefore, this study adopts the concept of maximizing multidimensional feature distortion, applying noise to clean samples to serve as subsequent training samples. On this basis, this study proposes an inverse perturbation elimination method based on Generative Adversarial Networks (GAN) to realize the adversarial defense, and design the generator and discriminator for infrared small targets, aiming to make both of them compete with each other to continuously improve the performance of the model, find out the commonalities and differences between the adversarial samples and the original samples. Through experimental verification, our defense algorithm is not only able to cope with multiple attacks but also performs well on different recognition models compared to commonly used defense algorithms, making it a plug-and-play efficient adversarial defense technique.Keywords
Cite This Article
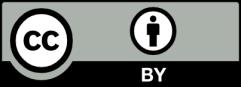
This work is licensed under a Creative Commons Attribution 4.0 International License , which permits unrestricted use, distribution, and reproduction in any medium, provided the original work is properly cited.