Open Access
ARTICLE
Stroke Electroencephalogram Data Synthesizing through Progressive Efficient Self-Attention Generative Adversarial Network
College of Electronic Information Engineering, Taiyuan University of Technology, Taiyuan, 030024, China
* Corresponding Author: Suzhe Wang. Email:
Computers, Materials & Continua 2024, 81(1), 1177-1196. https://doi.org/10.32604/cmc.2024.056016
Received 12 July 2024; Accepted 09 September 2024; Issue published 15 October 2024
Abstract
Early and timely diagnosis of stroke is critical for effective treatment, and the electroencephalogram (EEG) offers a low-cost, non-invasive solution. However, the shortage of high-quality patient EEG data often hampers the accuracy of diagnostic classification methods based on deep learning. To address this issue, our study designed a deep data amplification model named Progressive Conditional Generative Adversarial Network with Efficient Approximating Self Attention (PCGAN-EASA), which incrementally improves the quality of generated EEG features. This network can yield full-scale, fine-grained EEG features from the low-scale, coarse ones. Specially, to overcome the limitations of traditional generative models that fail to generate features tailored to individual patient characteristics, we developed an encoder with an effective approximating self-attention mechanism. This encoder not only automatically extracts relevant features across different patients but also reduces the computational resource consumption. Furthermore, the adversarial loss and reconstruction loss functions were redesigned to better align with the training characteristics of the network and the spatial correlations among electrodes. Extensive experimental results demonstrate that PCGAN-EASA provides the highest generation quality and the lowest computational resource usage compared to several existing approaches. Additionally, it significantly improves the accuracy of subsequent stroke classification tasks.Keywords
Cite This Article
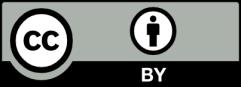
This work is licensed under a Creative Commons Attribution 4.0 International License , which permits unrestricted use, distribution, and reproduction in any medium, provided the original work is properly cited.