Open Access
ARTICLE
Research on Fine-Grained Recognition Method for Sensitive Information in Social Networks Based on CLIP
1 School of Computer Science, Zhongyuan University of Technology, Zhengzhou, 450007, China
2 Henan Key Laboratory of Cyberspace Situation Awareness, Zhengzhou, 450001, China
* Corresponding Author: Fangfang Shan. Email:
Computers, Materials & Continua 2024, 81(1), 1565-1580. https://doi.org/10.32604/cmc.2024.056008
Received 12 July 2024; Accepted 11 September 2024; Issue published 15 October 2024
Abstract
With the emergence and development of social networks, people can stay in touch with friends, family, and colleagues more quickly and conveniently, regardless of their location. This ubiquitous digital internet environment has also led to large-scale disclosure of personal privacy. Due to the complexity and subtlety of sensitive information, traditional sensitive information identification technologies cannot thoroughly address the characteristics of each piece of data, thus weakening the deep connections between text and images. In this context, this paper adopts the CLIP model as a modality discriminator. By using comparative learning between sensitive image descriptions and images, the similarity between the images and the sensitive descriptions is obtained to determine whether the images contain sensitive information. This provides the basis for identifying sensitive information using different modalities. Specifically, if the original data does not contain sensitive information, only single-modality text-sensitive information identification is performed; if the original data contains sensitive information, multi-modality sensitive information identification is conducted. This approach allows for differentiated processing of each piece of data, thereby achieving more accurate sensitive information identification. The aforementioned modality discriminator can address the limitations of existing sensitive information identification technologies, making the identification of sensitive information from the original data more appropriate and precise.Keywords
Cite This Article
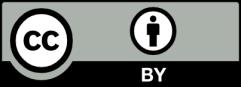
This work is licensed under a Creative Commons Attribution 4.0 International License , which permits unrestricted use, distribution, and reproduction in any medium, provided the original work is properly cited.