Open Access
ARTICLE
ResMHA-Net: Enhancing Glioma Segmentation and Survival Prediction Using a Novel Deep Learning Framework
1 Department of Computer Science, School of Engineering and Technology, Islamic University of Science and Technology, Awantipora, 192122, India
2 Faculty of Computing and Technology, Al-Baha University, Alaqiq, 65779-7738, Saudi Arabia
3 Research Groups in Intelligent Machines, National School of Engineers of Sfax, University of Sfax, Sfax, 3038, Tunisia
4 Department of Electrical Engineering, College of Engineering, King Khalid University, Abha, 61421, Saudi Arabia
5 Department of Computer Science and Engineering, Thapar Institute of Engineering and Technology, Patiala, 147004, Punjab
6 Department of Electrical and Instrumentation Engineering, Thapar Institute of Engineering and Technology, Patiala, 147004, Punjab
7 Department of AI and Software, Gachon University, Seongnam-si, 13120, Republic of Korea
* Corresponding Authors: Novsheena Rasool. Email: ; Muhammad Shahid Anwar. Email:
(This article belongs to the Special Issue: Medical Imaging Based Disease Diagnosis Using AI)
Computers, Materials & Continua 2024, 81(1), 885-909. https://doi.org/10.32604/cmc.2024.055900
Received 10 July 2024; Accepted 28 August 2024; Issue published 15 October 2024
Abstract
Gliomas are aggressive brain tumors known for their heterogeneity, unclear borders, and diverse locations on Magnetic Resonance Imaging (MRI) scans. These factors present significant challenges for MRI-based segmentation, a crucial step for effective treatment planning and monitoring of glioma progression. This study proposes a novel deep learning framework, ResNet Multi-Head Attention U-Net (ResMHA-Net), to address these challenges and enhance glioma segmentation accuracy. ResMHA-Net leverages the strengths of both residual blocks from the ResNet architecture and multi-head attention mechanisms. This powerful combination empowers the network to prioritize informative regions within the 3D MRI data and capture long-range dependencies. By doing so, ResMHA-Net effectively segments intricate glioma sub-regions and reduces the impact of uncertain tumor boundaries. We rigorously trained and validated ResMHA-Net on the BraTS 2018, 2019, 2020 and 2021 datasets. Notably, ResMHA-Net achieved superior segmentation accuracy on the BraTS 2021 dataset compared to the previous years, demonstrating its remarkable adaptability and robustness across diverse datasets. Furthermore, we collected the predicted masks obtained from three datasets to enhance survival prediction, effectively augmenting the dataset size. Radiomic features were then extracted from these predicted masks and, along with clinical data, were used to train a novel ensemble learning-based machine learning model for survival prediction. This model employs a voting mechanism aggregating predictions from multiple models, leading to significant improvements over existing methods. This ensemble approach capitalizes on the strengths of various models, resulting in more accurate and reliable predictions for patient survival. Importantly, we achieved an impressive accuracy of 73% for overall survival (OS) prediction.Keywords
Cite This Article
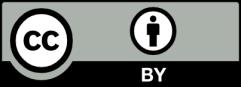
This work is licensed under a Creative Commons Attribution 4.0 International License , which permits unrestricted use, distribution, and reproduction in any medium, provided the original work is properly cited.