Open Access
ARTICLE
Continual Reinforcement Learning for Intelligent Agricultural Management under Climate Changes
1 Department of Mechanical Engineering, Iowa Technology Institute, University of Iowa, Iowa City, IA 52242, USA
2 Department of Electrical and Computer Engineering, University of Iowa, Iowa City, IA 52242, USA
* Corresponding Author: Shaoping Xiao. Email:
Computers, Materials & Continua 2024, 81(1), 1319-1336. https://doi.org/10.32604/cmc.2024.055809
Received 07 July 2024; Accepted 18 September 2024; Issue published 15 October 2024
Abstract
Climate change poses significant challenges to agricultural management, particularly in adapting to extreme weather conditions that impact agricultural production. Existing works with traditional Reinforcement Learning (RL) methods often falter under such extreme conditions. To address this challenge, our study introduces a novel approach by integrating Continual Learning (CL) with RL to form Continual Reinforcement Learning (CRL), enhancing the adaptability of agricultural management strategies. Leveraging the Gym-DSSAT simulation environment, our research enables RL agents to learn optimal fertilization strategies based on variable weather conditions. By incorporating CL algorithms, such as Elastic Weight Consolidation (EWC), with established RL techniques like Deep Q-Networks (DQN), we developed a framework in which agents can learn and retain knowledge across diverse weather scenarios. The CRL approach was tested under climate variability to assess the robustness and adaptability of the induced policies, particularly under extreme weather events like severe droughts. Our results showed that continually learned policies exhibited superior adaptability and performance compared to optimal policies learned through the conventional RL methods, especially in challenging conditions of reduced rainfall and increased temperatures. This pioneering work, which combines CL with RL to generate adaptive policies for agricultural management, is expected to make significant advancements in precision agriculture in the era of climate change.Keywords
Cite This Article
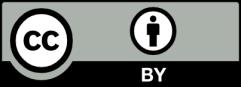
This work is licensed under a Creative Commons Attribution 4.0 International License , which permits unrestricted use, distribution, and reproduction in any medium, provided the original work is properly cited.