Open Access
ARTICLE
A Task Offloading Strategy Based on Multi-Agent Deep Reinforcement Learning for Offshore Wind Farm Scenarios
1 Department of Electrical Engineering, Guizhou University, Guiyang, 550025, China
2 Powerchina Guiyang Engineering Corporation Limited, Guiyang, 550081, China
3 Powerchina Guizhou Engineering Co., Ltd., Guiyang, 550001, China
* Corresponding Author: Xiao Wang. Email:
(This article belongs to the Special Issue: Collaborative Edge Intelligence and Its Emerging Applications)
Computers, Materials & Continua 2024, 81(1), 985-1008. https://doi.org/10.32604/cmc.2024.055614
Received 02 July 2024; Accepted 30 August 2024; Issue published 15 October 2024
Abstract
This research is the first application of Unmanned Aerial Vehicles (UAVs) equipped with Multi-access Edge Computing (MEC) servers to offshore wind farms, providing a new task offloading solution to address the challenge of scarce edge servers in offshore wind farms. The proposed strategy is to offload the computational tasks in this scenario to other MEC servers and compute them proportionally, which effectively reduces the computational pressure on local MEC servers when wind turbine data are abnormal. Finally, the task offloading problem is modeled as a multi-intelligent deep reinforcement learning problem, and a task offloading model based on Multi-Agent Deep Reinforcement Learning (MADRL) is established. The Adaptive Genetic Algorithm (AGA) is used to explore the action space of the Deep Deterministic Policy Gradient (DDPG), which effectively solves the problem of slow convergence of the DDPG algorithm in the high-dimensional action space. The simulation results show that the proposed algorithm, AGA-DDPG, saves approximately 61.8%, 55%, 21%, and 33% of the overall overhead compared to local MEC, random offloading, TD3, and DDPG, respectively. The proposed strategy is potentially important for improving real-time monitoring, big data analysis, and predictive maintenance of offshore wind farm operation and maintenance systems.Keywords
Cite This Article
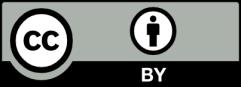
This work is licensed under a Creative Commons Attribution 4.0 International License , which permits unrestricted use, distribution, and reproduction in any medium, provided the original work is properly cited.