Open Access
ARTICLE
Blockchain-Enabled Federated Learning with Differential Privacy for Internet of Vehicles
1 School of Information Science and Technology, Zhengzhou Normal University, Zhengzhou, 450044, China
2 School of Electrical, Computer and Telecommunications Engineering, University of Wollongong, Wollongong, NSW 2500, Australia
* Corresponding Author: Zhijuan Jia. Email:
(This article belongs to the Special Issue: Trustworthy Wireless Computing Power Networks Assisted by Blockchain)
Computers, Materials & Continua 2024, 81(1), 1581-1593. https://doi.org/10.32604/cmc.2024.055557
Received 01 July 2024; Accepted 14 September 2024; Issue published 15 October 2024
Abstract
The rapid evolution of artificial intelligence (AI) technologies has significantly propelled the advancement of the Internet of Vehicles (IoV). With AI support, represented by machine learning technology, vehicles gain the capability to make intelligent decisions. As a distributed learning paradigm, federated learning (FL) has emerged as a preferred solution in IoV. Compared to traditional centralized machine learning, FL reduces communication overhead and improves privacy protection. Despite these benefits, FL still faces some security and privacy concerns, such as poisoning attacks and inference attacks, prompting exploration into blockchain integration to enhance its security posture. This paper introduces a novel blockchain-enabled federated learning (BCFL) scheme with differential privacy (DP) tailored for IoV. In order to meet the performance demanding IoV environment, the proposed methodology integrates a consortium blockchain with Practical Byzantine Fault Tolerance (PBFT) consensus, which offers superior efficiency over the conventional public blockchains. In addition, the proposed approach utilizes the Differentially Private Stochastic Gradient Descent (DP-SGD) algorithm in the local training process of FL for enhanced privacy protection. Experiment results indicate that the integration of blockchain elevates the security level of FL in that the proposed approach effectively safeguards FL against poisoning attacks. On the other hand, the additional overhead associated with blockchain integration is also limited to a moderate level to meet the efficiency criteria of IoV. Furthermore, by incorporating DP, the proposed approach is shown to have the (-) privacy guarantee while maintaining an acceptable level of model accuracy. This enhancement effectively mitigates the threat of inference attacks on private information.Keywords
Cite This Article
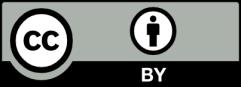
This work is licensed under a Creative Commons Attribution 4.0 International License , which permits unrestricted use, distribution, and reproduction in any medium, provided the original work is properly cited.