Open Access
ARTICLE
Research on Tensor Multi-Clustering Distributed Incremental Updating Method for Big Data
1 School of Computer Science, Nanjing University of Posts and Telecommunications, Nanjing, 210023, China
2 Ministry of Science and Technology Innovation, China Communications Services Corporation Limited, Beijing, 100071, China
3 School of Artificial Intelligence, The University of Manchester, Manchester, M13 9PL, UK
4 Ministry of Technology Innovation, China Telecom Artificial Intelligence Technology (Beijing) Corporation Limited, Beijing, 100032, China
5 Ministry of Science and Technology Innovation, Zhongbo Information Technology Research Institute Corporation Limited, Nanjing, 210012, China
6 Jiangsu Postal Big Data Technology and Application Engineering Research Center, Nanjing University of Posts and Telecommunications, Nanjing, 210003, China
* Corresponding Author: Peng Li. Email:
Computers, Materials & Continua 2024, 81(1), 1409-1432. https://doi.org/10.32604/cmc.2024.055406
Received 26 June 2024; Accepted 10 September 2024; Issue published 15 October 2024
Abstract
The scale and complexity of big data are growing continuously, posing severe challenges to traditional data processing methods, especially in the field of clustering analysis. To address this issue, this paper introduces a new method named Big Data Tensor Multi-Cluster Distributed Incremental Update (BDTMCDIncreUpdate), which combines distributed computing, storage technology, and incremental update techniques to provide an efficient and effective means for clustering analysis. Firstly, the original dataset is divided into multiple sub-blocks, and distributed computing resources are utilized to process the sub-blocks in parallel, enhancing efficiency. Then, initial clustering is performed on each sub-block using tensor-based multi-clustering techniques to obtain preliminary results. When new data arrives, incremental update technology is employed to update the core tensor and factor matrix, ensuring that the clustering model can adapt to changes in data. Finally, by combining the updated core tensor and factor matrix with historical computational results, refined clustering results are obtained, achieving real-time adaptation to dynamic data. Through experimental simulation on the Aminer dataset, the BDTMCDIncreUpdate method has demonstrated outstanding performance in terms of accuracy (ACC) and normalized mutual information (NMI) metrics, achieving an accuracy rate of 90% and an NMI score of 0.85, which outperforms existing methods such as TClusInitUpdate and TKLClusUpdate in most scenarios. Therefore, the BDTMCDIncreUpdate method offers an innovative solution to the field of big data analysis, integrating distributed computing, incremental updates, and tensor-based multi-clustering techniques. It not only improves the efficiency and scalability in processing large-scale high-dimensional datasets but also has been validated for its effectiveness and accuracy through experiments. This method shows great potential in real-world applications where dynamic data growth is common, and it is of significant importance for advancing the development of data analysis technology.Keywords
Cite This Article
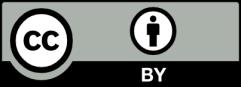
This work is licensed under a Creative Commons Attribution 4.0 International License , which permits unrestricted use, distribution, and reproduction in any medium, provided the original work is properly cited.