Open Access
ARTICLE
Multi-UAV Collaborative Mission Planning Method for Self-Organized Sensor Data Acquisition
1 School of Information Science and Technology, Beijing Forestry University, Beijing, 100083, China
2 Engineering Research Center for Forestry-Oriented Intelligent Information Processing of National Forestry and Grassland Administration, Beijing, 100083, China
3 State Key Laboratory of Efficient Production of Forest Resources, Beijing, 100083, China
4 Division of Biostatistics, Department of Population Heath, Grossman School of Medicine, New York University, New York, NY 10016, USA
* Corresponding Author: Xiaohui Cui. Email:
(This article belongs to the Special Issue: AI-Assisted Energy Harvesting Techniques and its Applications in Wireless Sensor Networks)
Computers, Materials & Continua 2024, 81(1), 1529-1563. https://doi.org/10.32604/cmc.2024.055402
Received 26 June 2024; Accepted 14 September 2024; Issue published 15 October 2024
Abstract
In recent years, sensor technology has been widely used in the defense and control of sensitive areas in cities, or in various scenarios such as early warning of forest fires, monitoring of forest pests and diseases, and protection of endangered animals. Deploying sensors to collect data and then utilizing unmanned aerial vehicle (UAV) to collect the data stored in the sensors has replaced traditional manual data collection as the dominant method. The current strategies for efficient data collection in above scenarios are still imperfect, and the low quality of the collected data and the excessive energy consumed by UAV flights are still the main problems faced in data collection. With regards this, this paper proposes a multi-UAV mission planning method for self-organized sensor data acquisition by comprehensively utilizing the techniques of self-organized sensor clustering, multi-UAV mission area allocation, and sub-area data acquisition scheme optimization. The improved α-hop clustering method utilizes the average transmission distance to reduce the size of the collection sensors, and the K-Dimensional method is used to form a multi-UAV cooperative workspace, and then, the genetic algorithm is used to trade-off the speed with the age of information (AoI) of the collected information and the energy consumption to form the multi-UAV data collection operation scheme. The combined optimization scheme in paper improves the performance by 95.56% and 58.21%, respectively, compared to the traditional baseline model. In order to verify the excellent generalization and applicability of the proposed method in real scenarios, the simulation test is conducted by introducing the digital elevation model data of the real terrain, and the results show that the relative error values of the proposed method and the performance test of the actual flight of the UAV are within the error interval of ±10%. Then, the advantages and disadvantages of the present method with the existing mainstream schemes are tested, and the results show that the present method has a huge advantage in terms of space and time complexity, and at the same time, the accuracy for data extraction is relatively improved by 10.46% and 12.71%. Finally, by eliminating the clustering process and the subtask assignment process, the AoI performance decreases by 3.46× and 4.45×, and the energy performance decreases by 3.52× and 4.47×. This paper presents a comprehensive and detailed proactive optimization of the existing challenges faced in the field of data acquisition by means of a series of combinatorial optimizations.Keywords
Cite This Article
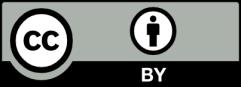
This work is licensed under a Creative Commons Attribution 4.0 International License , which permits unrestricted use, distribution, and reproduction in any medium, provided the original work is properly cited.