Open Access
ARTICLE
Data-Driven Decision-Making for Bank Target Marketing Using Supervised Learning Classifiers on Imbalanced Big Data
1 School of Computer Science and Informatics, De Montfort University, Leicester, LE1 9BH, UK
2 Faculty of Computer Science and Information Technology, Universiti Tun Hussein Onn Malaysia, Johor, 86400, Malaysia
* Corresponding Author: Abdulghani Ali Ahmed. Email:
Computers, Materials & Continua 2024, 81(1), 1703-1728. https://doi.org/10.32604/cmc.2024.055192
Received 20 June 2024; Accepted 26 August 2024; Issue published 15 October 2024
Abstract
Integrating machine learning and data mining is crucial for processing big data and extracting valuable insights to enhance decision-making. However, imbalanced target variables within big data present technical challenges that hinder the performance of supervised learning classifiers on key evaluation metrics, limiting their overall effectiveness. This study presents a comprehensive review of both common and recently developed Supervised Learning Classifiers (SLCs) and evaluates their performance in data-driven decision-making. The evaluation uses various metrics, with a particular focus on the Harmonic Mean Score (F-1 score) on an imbalanced real-world bank target marketing dataset. The findings indicate that grid-search random forest and random-search random forest excel in Precision and area under the curve, while Extreme Gradient Boosting (XGBoost) outperforms other traditional classifiers in terms of F-1 score. Employing oversampling methods to address the imbalanced data shows significant performance improvement in XGBoost, delivering superior results across all metrics, particularly when using the SMOTE variant known as the BorderlineSMOTE2 technique. The study concludes several key factors for effectively addressing the challenges of supervised learning with imbalanced datasets. These factors include the importance of selecting appropriate datasets for training and testing, choosing the right classifiers, employing effective techniques for processing and handling imbalanced datasets, and identifying suitable metrics for performance evaluation. Additionally, factors also entail the utilisation of effective exploratory data analysis in conjunction with visualisation techniques to yield insights conducive to data-driven decision-making.Keywords
Cite This Article
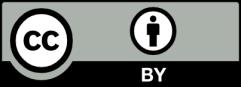
This work is licensed under a Creative Commons Attribution 4.0 International License , which permits unrestricted use, distribution, and reproduction in any medium, provided the original work is properly cited.