Open Access
ARTICLE
Adaptive Successive POI Recommendation via Trajectory Sequences Processing and Long Short-Term Preference Learning
1 School of Computer Science and Engineering, Changshu Institute of Technology, Changshu, 215500, China
2 Suzhou Industrial Intelligence and Big Data Laboratory, Suzhou, 215000, China
3 School of Information Science and Engineering, Yanshan University, Qinhuangdao, 066004, China
4 College of Electronic and Information Engineering, Guangdong Ocean University, Zhanjiang, 524088, China
* Corresponding Author: Feng Li. Email:
Computers, Materials & Continua 2024, 81(1), 685-706. https://doi.org/10.32604/cmc.2024.055141
Received 18 June 2024; Accepted 27 August 2024; Issue published 15 October 2024
Abstract
Point-of-interest (POI) recommendations in location-based social networks (LBSNs) have developed rapidly by incorporating feature information and deep learning methods. However, most studies have failed to accurately reflect different users’ preferences, in particular, the short-term preferences of inactive users. To better learn user preferences, in this study, we propose a long-short-term-preference-based adaptive successive POI recommendation (LSTP-ASR) method by combining trajectory sequence processing, long short-term preference learning, and spatiotemporal context. First, the check-in trajectory sequences are adaptively divided into recent and historical sequences according to a dynamic time window. Subsequently, an adaptive filling strategy is used to expand the recent check-in sequences of users with inactive check-in behavior using those of similar active users. We further propose an adaptive learning model to accurately extract long short-term preferences of users to establish an efficient successive POI recommendation system. A spatiotemporal-context-based recurrent neural network and temporal-context-based long short-term memory network are used to model the users’ recent and historical check-in trajectory sequences, respectively. Extensive experiments on the Foursquare and Gowalla datasets reveal that the proposed method outperforms several other baseline methods in terms of three evaluation metrics. More specifically, LSTP-ASR outperforms the previously best baseline method (RTPM) with a 17.15% and 20.62% average improvement on the Foursquare and Gowalla datasets in terms of the Fβ metric, respectively.Keywords
Cite This Article
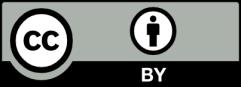
This work is licensed under a Creative Commons Attribution 4.0 International License , which permits unrestricted use, distribution, and reproduction in any medium, provided the original work is properly cited.