Open Access
ARTICLE
Multi-Label Feature Selection Based on Improved Ant Colony Optimization Algorithm with Dynamic Redundancy and Label Dependence
1 School of Computer, Hubei University of Technology, Wuhan, 430068, China
2 Xining Big Data Service Administration, Xining, 810000, China
3 Wuhan Fiberhome Technical Services Co., Ltd., Wuhan, 430205, China
* Corresponding Author: Zhiwei Ye. Email:
(This article belongs to the Special Issue: Metaheuristic-Driven Optimization Algorithms: Methods and Applications)
Computers, Materials & Continua 2024, 81(1), 1157-1175. https://doi.org/10.32604/cmc.2024.055080
Received 16 June 2024; Accepted 01 September 2024; Issue published 15 October 2024
Abstract
The world produces vast quantities of high-dimensional multi-semantic data. However, extracting valuable information from such a large amount of high-dimensional and multi-label data is undoubtedly arduous and challenging. Feature selection aims to mitigate the adverse impacts of high dimensionality in multi-label data by eliminating redundant and irrelevant features. The ant colony optimization algorithm has demonstrated encouraging outcomes in multi-label feature selection, because of its simplicity, efficiency, and similarity to reinforcement learning. Nevertheless, existing methods do not consider crucial correlation information, such as dynamic redundancy and label correlation. To tackle these concerns, the paper proposes a multi-label feature selection technique based on ant colony optimization algorithm (MFACO), focusing on dynamic redundancy and label correlation. Initially, the dynamic redundancy is assessed between the selected feature subset and potential features. Meanwhile, the ant colony optimization algorithm extracts label correlation from the label set, which is then combined into the heuristic factor as label weights. Experimental results demonstrate that our proposed strategies can effectively enhance the optimal search ability of ant colony, outperforming the other algorithms involved in the paper.Keywords
Cite This Article
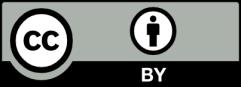
This work is licensed under a Creative Commons Attribution 4.0 International License , which permits unrestricted use, distribution, and reproduction in any medium, provided the original work is properly cited.