Open Access
ARTICLE
Efficient and Cost-Effective Vehicle Detection in Foggy Weather for Edge/Fog-Enabled Traffic Surveillance and Collision Avoidance Systems
1 Department of Computer Science, National Textile University, Faisalabad, 37610, Pakistan
2 College of Computer and Information Sciences, Imam Mohammad Ibn Saud Islamic University (IMSIU), Riyadh, 11432, Saudi Arabia
3 Department of Computer Science, University of Agriculture, Faisalabad, 38000, Pakistan
* Corresponding Author: Qaisar Abbas. Email:
Computers, Materials & Continua 2024, 81(1), 911-931. https://doi.org/10.32604/cmc.2024.055049
Received 14 June 2024; Accepted 02 September 2024; Issue published 15 October 2024
Abstract
Vision-based vehicle detection in adverse weather conditions such as fog, haze, and mist is a challenging research area in the fields of autonomous vehicles, collision avoidance, and Internet of Things (IoT)-enabled edge/fog computing traffic surveillance and monitoring systems. Efficient and cost-effective vehicle detection at high accuracy and speed in foggy weather is essential to avoiding road traffic collisions in real-time. To evaluate vision-based vehicle detection performance in foggy weather conditions, state-of-the-art Vehicle Detection in Adverse Weather Nature (DAWN) and Foggy Driving (FD) datasets are self-annotated using the YOLO LABEL tool and customized to four vehicle detection classes: cars, buses, motorcycles, and trucks. The state-of-the-art single-stage deep learning algorithms YOLO-V5, and YOLO-V8 are considered for the task of vehicle detection. Furthermore, YOLO-V5s is enhanced by introducing attention modules Convolutional Block Attention Module (CBAM), Normalized-based Attention Module (NAM), and Simple Attention Module (SimAM) after the SPPF module as well as YOLO-V5l with BiFPN. Their vehicle detection accuracy parameters and running speed is validated on cloud (Google Colab) and edge (local) systems. The mAP50 score of YOLO-V5n is 72.60%, YOLO-V5s is 75.20%, YOLO-V5m is 73.40%, and YOLO-V5l is 77.30%; and YOLO-V8n is 60.20%, YOLO-V8s is 73.50%, YOLO-V8m is 73.80%, and YOLO-V8l is 72.60% on DAWN dataset. The mAP50 score of YOLO-V5n is 43.90%, YOLO-V5s is 40.10%, YOLO-V5m is 49.70%, and YOLO-V5l is 57.30%; and YOLO-V8n is 41.60%, YOLO-V8s is 46.90%, YOLO-V8m is 42.90%, and YOLO-V8l is 44.80% on FD dataset. The vehicle detection speed of YOLO-V5n is 59 Frame Per Seconds (FPS), YOLO-V5s is 47 FPS, YOLO-V5m is 38 FPS, and YOLO-V5l is 30 FPS; and YOLO-V8n is 185 FPS, YOLO-V8s is 109 FPS, YOLO-V8m is 72 FPS, and YOLO-V8l is 63 FPS on DAWN dataset. The vehicle detection speed of YOLO-V5n is 26 FPS, YOLO-V5s is 24 FPS, YOLO-V5m is 22 FPS, and YOLO-V5l is 17 FPS; and YOLO-V8n is 313 FPS, YOLO-V8s is 182 FPS, YOLO-V8m is 99 FPS, and YOLO-V8l is 60 FPS on FD dataset. YOLO-V5s, YOLO-V5s variants and YOLO-V5l_BiFPN, and YOLO-V8 algorithms are efficient and cost-effective solution for real-time vision-based vehicle detection in foggy weather.Keywords
Cite This Article
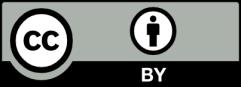
This work is licensed under a Creative Commons Attribution 4.0 International License , which permits unrestricted use, distribution, and reproduction in any medium, provided the original work is properly cited.