Open Access
ARTICLE
APSO-CNN-SE: An Adaptive Convolutional Neural Network Approach for IoT Intrusion Detection
College of Big Data and Information Engineering, Guizhou University, Guizhou, 550025, China
* Corresponding Author: Damin Zhang. Email:
Computers, Materials & Continua 2024, 81(1), 567-601. https://doi.org/10.32604/cmc.2024.055007
Received 13 June 2024; Accepted 22 August 2024; Issue published 15 October 2024
Abstract
The surge in connected devices and massive data aggregation has expanded the scale of the Internet of Things (IoT) networks. The proliferation of unknown attacks and related risks, such as zero-day attacks and Distributed Denial of Service (DDoS) attacks triggered by botnets, have resulted in information leakage and property damage. Therefore, developing an efficient and realistic intrusion detection system (IDS) is critical for ensuring IoT network security. In recent years, traditional machine learning techniques have struggled to learn the complex associations between multidimensional features in network traffic, and the excellent performance of deep learning techniques, as an advanced version of machine learning, has led to their widespread application in intrusion detection. In this paper, we propose an Adaptive Particle Swarm Optimization Convolutional Neural Network Squeeze-and-Excitation (APSO-CNN-SE) model for implementing IoT network intrusion detection. A 2D CNN backbone is initially constructed to extract spatial features from network traffic. Subsequently, a squeeze-and-excitation channel attention mechanism is introduced and embedded into the CNN to focus on critical feature channels. Lastly, the weights and biases in the CNN-SE are extracted to initialize the population individuals of the APSO. As the number of iterations increases, the population’s position vector is continuously updated, and the cross-entropy loss function value is minimized to produce the ideal network architecture. We evaluated the models experimentally using binary and multiclassification on the UNSW-NB15 and NSL-KDD datasets, comparing and analyzing the evaluation metrics derived from each model. Compared to the base CNN model, the results demonstrate that APSO-CNN-SE enhances the binary classification detection accuracy by 1.84% and 3.53% and the multiclassification detection accuracy by 1.56% and 2.73% on the two datasets, respectively. Additionally, the model outperforms the existing models like DT, KNN, LR, SVM, LSTM, etc., in terms of accuracy and fitting performance. This means that the model can identify potential attacks or anomalies more precisely, improving the overall security and stability of the IoT environment.Keywords
Cite This Article
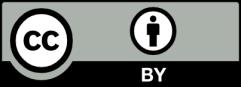
This work is licensed under a Creative Commons Attribution 4.0 International License , which permits unrestricted use, distribution, and reproduction in any medium, provided the original work is properly cited.