Open Access
ARTICLE
Re-Distributing Facial Features for Engagement Prediction with ModernTCN
1 College of Information and Artificial Intelligence, Nanchang Institute of Science and Technology, Nanchang, 330108, China
2 School of Electrical and Information Engineering, Wuhan Institute of Technology, Wuhan, 430205, China
3 School of Electronic Information Engineering, Wuhan Donghu University, Wuhan, 430212, China
* Corresponding Authors: Qian Li. Email: ; Changhui Hou. Email:
(This article belongs to the Special Issue: The Latest Deep Learning Architectures for Artificial Intelligence Applications)
Computers, Materials & Continua 2024, 81(1), 369-391. https://doi.org/10.32604/cmc.2024.054982
Received 13 June 2024; Accepted 22 August 2024; Issue published 15 October 2024
Abstract
Automatically detecting learners’ engagement levels helps to develop more effective online teaching and assessment programs, allowing teachers to provide timely feedback and make personalized adjustments based on students’ needs to enhance teaching effectiveness. Traditional approaches mainly rely on single-frame multimodal facial spatial information, neglecting temporal emotional and behavioural features, with accuracy affected by significant pose variations. Additionally, convolutional padding can erode feature maps, affecting feature extraction’s representational capacity. To address these issues, we propose a hybrid neural network architecture, the redistributing facial features and temporal convolutional network (RefEIP). This network consists of three key components: first, utilizing the spatial attention mechanism large kernel attention (LKA) to automatically capture local patches and mitigate the effects of pose variations; second, employing the feature organization and weight distribution (FOWD) module to redistribute feature weights and eliminate the impact of white features and enhancing representation in facial feature maps. Finally, we analyse the temporal changes in video frames through the modern temporal convolutional network (ModernTCN) module to detect engagement levels. We constructed a near-infrared engagement video dataset (NEVD) to better validate the efficiency of the RefEIP network. Through extensive experiments and in-depth studies, we evaluated these methods on the NEVD and the Database for Affect in Situations of Elicitation (DAiSEE), achieving an accuracy of 90.8% on NEVD and 61.2% on DAiSEE in the four-class classification task, indicating significant advantages in addressing engagement video analysis problems.Keywords
Cite This Article
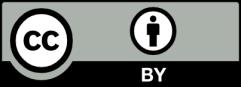
This work is licensed under a Creative Commons Attribution 4.0 International License , which permits unrestricted use, distribution, and reproduction in any medium, provided the original work is properly cited.