Open Access
ARTICLE
A Secure Framework for WSN-IoT Using Deep Learning for Enhanced Intrusion Detection
1 School of Computer Science & Engineering, Vellore Institute of Technology, Chennai Campus, Chennai, 600127, India
2 School of Electronics & Engineering, Vellore Institute of Technology, Chennai Campus, Chennai, 600127, India
3 Department of Computer Sciences and Engineering, College of Applied Science, King Saud University, Riyadh, 11543, Saudi Arabia
4 Department of Computer Engineering, College of Computer and Information Sciences, King Saud University, Riyadh, 11543, Saudi Arabia
* Corresponding Author: Chandraumakantham Om Kumar. Email:
Computers, Materials & Continua 2024, 81(1), 471-501. https://doi.org/10.32604/cmc.2024.054966
Received 12 June 2024; Accepted 31 July 2024; Issue published 15 October 2024
Abstract
The security of the wireless sensor network-Internet of Things (WSN-IoT) network is more challenging due to its randomness and self-organized nature. Intrusion detection is one of the key methodologies utilized to ensure the security of the network. Conventional intrusion detection mechanisms have issues such as higher misclassification rates, increased model complexity, insignificant feature extraction, increased training time, increased run time complexity, computation overhead, failure to identify new attacks, increased energy consumption, and a variety of other factors that limit the performance of the intrusion system model. In this research a security framework for WSN-IoT, through a deep learning technique is introduced using Modified Fuzzy-Adaptive DenseNet (MF_AdaDenseNet) and is benchmarked with datasets like NSL-KDD, UNSWNB15, CIDDS-001, Edge IIoT, Bot IoT. In this, the optimal feature selection using Capturing Dingo Optimization (CDO) is devised to acquire relevant features by removing redundant features. The proposed MF_AdaDenseNet intrusion detection model offers significant benefits by utilizing optimal feature selection with the CDO algorithm. This results in enhanced Detection Capacity with minimal computation complexity, as well as a reduction in False Alarm Rate (FAR) due to the consideration of classification error in the fitness estimation. As a result, the combined CDO-based feature selection and MF_AdaDenseNet intrusion detection mechanism outperform other state-of-the-art techniques, achieving maximal Detection Capacity, precision, recall, and F-Measure of 99.46%, 99.54%, 99.91%, and 99.68%, respectively, along with minimal FAR and Mean Absolute Error (MAE) of 0.9% and 0.11.Keywords
Cite This Article
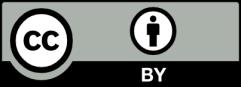
This work is licensed under a Creative Commons Attribution 4.0 International License , which permits unrestricted use, distribution, and reproduction in any medium, provided the original work is properly cited.