Open Access
ARTICLE
Advancing Autoencoder Architectures for Enhanced Anomaly Detection in Multivariate Industrial Time Series
1 Department of Security Convergence, Chung-Ang University, Seoul, 06974, Republic of Korea
2 Department of Computer Science, COMSATS University Islamabad, Attock Campus, Attock, 43600, Pakistan
3 Department of AI and Big Data, Soonchunhyang University, Asan, 31538, Republic of Korea
4 Department of Industrial Security, Chung-Ang University, Seoul, 06974, Republic of Korea
* Corresponding Authors: Jihoon Moon. Email: ; Seungmin Rho. Email:
(This article belongs to the Special Issue: AI and Data Security for the Industrial Internet)
Computers, Materials & Continua 2024, 81(1), 1275-1300. https://doi.org/10.32604/cmc.2024.054826
Received 08 June 2024; Accepted 10 September 2024; Issue published 15 October 2024
Abstract
In the context of rapid digitization in industrial environments, how effective are advanced unsupervised learning models, particularly hybrid autoencoder models, at detecting anomalies in industrial control system (ICS) datasets? This study is crucial because it addresses the challenge of identifying rare and complex anomalous patterns in the vast amounts of time series data generated by Internet of Things (IoT) devices, which can significantly improve the reliability and safety of these systems. In this paper, we propose a hybrid autoencoder model, called ConvBiLSTM-AE, which combines convolutional neural network (CNN) and bidirectional long short-term memory (BiLSTM) to more effectively train complex temporal data patterns in anomaly detection. On the hardware-in-the-loop-based extended industrial control system dataset, the ConvBiLSTM-AE model demonstrated remarkable anomaly detection performance, achieving F1 scores of 0.78 and 0.41 for the first and second datasets, respectively. The results suggest that hybrid autoencoder models are not only viable, but potentially superior alternatives for unsupervised anomaly detection in complex industrial systems, offering a promising approach to improving their reliability and safety.Keywords
Cite This Article
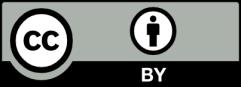
This work is licensed under a Creative Commons Attribution 4.0 International License , which permits unrestricted use, distribution, and reproduction in any medium, provided the original work is properly cited.