Open Access
ARTICLE
Border Sensitive Knowledge Distillation for Rice Panicle Detection in UAV Images
School of Computer Science and Engineering, VIT (Vellore Institute of Technology) Vellore, Vellore, 632014, India
* Corresponding Author: Sendhil Kumar K.S.. Email:
(This article belongs to the Special Issue: Multimodal Learning in Image Processing)
Computers, Materials & Continua 2024, 81(1), 827-842. https://doi.org/10.32604/cmc.2024.054768
Received 06 June 2024; Accepted 11 August 2024; Issue published 15 October 2024
Abstract
Research on panicle detection is one of the most important aspects of paddy phenotypic analysis. A phenotyping method that uses unmanned aerial vehicles can be an excellent alternative to field-based methods. Nevertheless, it entails many other challenges, including different illuminations, panicle sizes, shape distortions, partial occlusions, and complex backgrounds. Object detection algorithms are directly affected by these factors. This work proposes a model for detecting panicles called Border Sensitive Knowledge Distillation (BSKD). It is designed to prioritize the preservation of knowledge in border areas through the use of feature distillation. Our feature-based knowledge distillation method allows us to compress the model without sacrificing its effectiveness. An imitation mask is used to distinguish panicle-related foreground features from irrelevant background features. A significant improvement in Unmanned Aerial Vehicle (UAV) images is achieved when students imitate the teacher’s features. On the UAV rice imagery dataset, the proposed BSKD model shows superior performance with 76.3% mAP, 88.3% precision, 90.1% recall and 92.6% F1 score.Keywords
Cite This Article
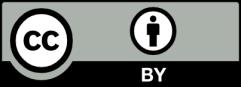
This work is licensed under a Creative Commons Attribution 4.0 International License , which permits unrestricted use, distribution, and reproduction in any medium, provided the original work is properly cited.