Open Access
ARTICLE
LKMT: Linguistics Knowledge-Driven Multi-Task Neural Machine Translation for Urdu and English
1 Faculty of Information Engineering and Automation, Kunming University of Science and Technology, Kunming, 650500, China
2 Yunnan Key Laboratory of Artificial Intelligence, Kunming University of Science and Technology, Kunming, 650500, China
* Corresponding Author: Zhengtao Yu. Email:
(This article belongs to the Special Issue: Advancements in Natural Language Processing (NLP) and Fuzzy Logic)
Computers, Materials & Continua 2024, 81(1), 951-969. https://doi.org/10.32604/cmc.2024.054673
Received 04 June 2024; Accepted 26 August 2024; Issue published 15 October 2024
Abstract
Thanks to the strong representation capability of pre-trained language models, supervised machine translation models have achieved outstanding performance. However, the performances of these models drop sharply when the scale of the parallel training corpus is limited. Considering the pre-trained language model has a strong ability for monolingual representation, it is the key challenge for machine translation to construct the in-depth relationship between the source and target language by injecting the lexical and syntactic information into pre-trained language models. To alleviate the dependence on the parallel corpus, we propose a Linguistics Knowledge-Driven Multi-Task (LKMT) approach to inject part-of-speech and syntactic knowledge into pre-trained models, thus enhancing the machine translation performance. On the one hand, we integrate part-of-speech and dependency labels into the embedding layer and exploit large-scale monolingual corpus to update all parameters of pre-trained language models, thus ensuring the updated language model contains potential lexical and syntactic information. On the other hand, we leverage an extra self-attention layer to explicitly inject linguistic knowledge into the pre-trained language model-enhanced machine translation model. Experiments on the benchmark dataset show that our proposed LKMT approach improves the Urdu-English translation accuracy by 1.97 points and the English-Urdu translation accuracy by 2.42 points, highlighting the effectiveness of our LKMT framework. Detailed ablation experiments confirm the positive impact of part-of-speech and dependency parsing on machine translation.Keywords
Cite This Article
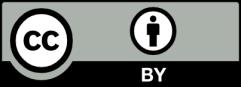
This work is licensed under a Creative Commons Attribution 4.0 International License , which permits unrestricted use, distribution, and reproduction in any medium, provided the original work is properly cited.