Open Access
ARTICLE
A Facial Expression Recognition Method Integrating Uncertainty Estimation and Active Learning
1 School of Computer Science and Engineering, Chongqing University of Technology, Chongqing, 400054, China
2 Data Space Research Institute of Hefei Comprehensive National Science Center, Hefei, 230088, China
* Corresponding Author: Jianxun Zhang. Email:
Computers, Materials & Continua 2024, 81(1), 533-548. https://doi.org/10.32604/cmc.2024.054644
Received 03 June 2024; Accepted 23 August 2024; Issue published 15 October 2024
Abstract
The effectiveness of facial expression recognition (FER) algorithms hinges on the model’s quality and the availability of a substantial amount of labeled expression data. However, labeling large datasets demands significant human, time, and financial resources. Although active learning methods have mitigated the dependency on extensive labeled data, a cold-start problem persists in small to medium-sized expression recognition datasets. This issue arises because the initial labeled data often fails to represent the full spectrum of facial expression characteristics. This paper introduces an active learning approach that integrates uncertainty estimation, aiming to improve the precision of facial expression recognition regardless of dataset scale variations. The method is divided into two primary phases. First, the model undergoes self-supervised pre-training using contrastive learning and uncertainty estimation to bolster its feature extraction capabilities. Second, the model is fine-tuned using the prior knowledge obtained from the pre-training phase to significantly improve recognition accuracy. In the pre-training phase, the model employs contrastive learning to extract fundamental feature representations from the complete unlabeled dataset. These features are then weighted through a self-attention mechanism with rank regularization. Subsequently, data from the low-weighted set is relabeled to further refine the model’s feature extraction ability. The pre-trained model is then utilized in active learning to select and label information-rich samples more efficiently. Experimental results demonstrate that the proposed method significantly outperforms existing approaches, achieving an improvement in recognition accuracy of 5.09% and 3.82% over the best existing active learning methods, Margin, and Least Confidence methods, respectively, and a 1.61% improvement compared to the conventional segmented active learning method.Keywords
Cite This Article
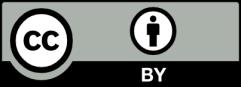
This work is licensed under a Creative Commons Attribution 4.0 International License , which permits unrestricted use, distribution, and reproduction in any medium, provided the original work is properly cited.