Open Access
ARTICLE
PUNet: A Semi-Supervised Anomaly Detection Model for Network Anomaly Detection Based on Positive Unlabeled Data
Faculty of Computing, Harbin Institute of Technology, Harbin, 150000, China
* Corresponding Author: Zhaoxin Zhang. Email:
Computers, Materials & Continua 2024, 81(1), 327-343. https://doi.org/10.32604/cmc.2024.054558
Received 31 May 2024; Accepted 19 August 2024; Issue published 15 October 2024
Abstract
Network anomaly detection plays a vital role in safeguarding network security. However, the existing network anomaly detection task is typically based on the one-class zero-positive scenario. This approach is susceptible to overfitting during the training process due to discrepancies in data distribution between the training set and the test set. This phenomenon is known as prediction drift. Additionally, the rarity of anomaly data, often masked by normal data, further complicates network anomaly detection. To address these challenges, we propose the PUNet network, which ingeniously combines the strengths of traditional machine learning and deep learning techniques for anomaly detection. Specifically, PUNet employs a reconstruction-based autoencoder to pre-train normal data, enabling the network to capture potential features and correlations within the data. Subsequently, PUNet integrates a sampling algorithm to construct a pseudo-label candidate set among the outliers based on the reconstruction loss of the samples. This approach effectively mitigates the prediction drift problem by incorporating abnormal samples. Furthermore, PUNet utilizes the CatBoost classifier for anomaly detection to tackle potential data imbalance issues within the candidate set. Extensive experimental evaluations demonstrate that PUNet effectively resolves the prediction drift and data imbalance problems, significantly outperforming competing methods.Keywords
Cite This Article
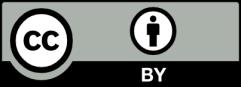
This work is licensed under a Creative Commons Attribution 4.0 International License , which permits unrestricted use, distribution, and reproduction in any medium, provided the original work is properly cited.