Open Access
ARTICLE
Hierarchical Optimization Method for Federated Learning with Feature Alignment and Decision Fusion
1 College of Computer Science and Engineering, North Minzu University, Yinchuan, 750021, China
2 The Key Laboratory of Images & Graphics Intelligent Processing of State Ethnic Affairs Commission, North Minzu University, Yinchuan, 750021, China
* Corresponding Authors: Ke Li. Email: ; Xiaofeng Wang. Email:
Computers, Materials & Continua 2024, 81(1), 1391-1407. https://doi.org/10.32604/cmc.2024.054484
Received 29 May 2024; Accepted 13 September 2024; Issue published 15 October 2024
Abstract
In the realm of data privacy protection, federated learning aims to collaboratively train a global model. However, heterogeneous data between clients presents challenges, often resulting in slow convergence and inadequate accuracy of the global model. Utilizing shared feature representations alongside customized classifiers for individual clients emerges as a promising personalized solution. Nonetheless, previous research has frequently neglected the integration of global knowledge into local representation learning and the synergy between global and local classifiers, thereby limiting model performance. To tackle these issues, this study proposes a hierarchical optimization method for federated learning with feature alignment and the fusion of classification decisions (FedFCD). FedFCD regularizes the relationship between global and local feature representations to achieve alignment and incorporates decision information from the global classifier, facilitating the late fusion of decision outputs from both global and local classifiers. Additionally, FedFCD employs a hierarchical optimization strategy to flexibly optimize model parameters. Through experiments on the Fashion-MNIST, CIFAR-10 and CIFAR-100 datasets, we demonstrate the effectiveness and superiority of FedFCD. For instance, on the CIFAR-100 dataset, FedFCD exhibited a significant improvement in average test accuracy by 6.83% compared to four outstanding personalized federated learning approaches. Furthermore, extended experiments confirm the robustness of FedFCD across various hyperparameter values.Keywords
Cite This Article
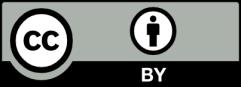
This work is licensed under a Creative Commons Attribution 4.0 International License , which permits unrestricted use, distribution, and reproduction in any medium, provided the original work is properly cited.