Open Access
REVIEW
Wearable Healthcare and Continuous Vital Sign Monitoring with IoT Integration
1 Department of Arts, Communications and Social Sciences, University Canada West, Vancouver, BC V6Z 0E5, Canada
2 GUS Institute, Global University Systems, London, EC1N 2LX, UK
3 Hamta Group, Hamta Business Corporation, Vancouver, BC V6E 1C9, Canada
4 Q Minded, Quark Minded Technology Inc., Vancouver, BC V6E 1C9, Canada
* Corresponding Author: Hamed Taherdoost. Email:
Computers, Materials & Continua 2024, 81(1), 79-104. https://doi.org/10.32604/cmc.2024.054378
Received 27 May 2024; Accepted 14 September 2024; Issue published 15 October 2024
Abstract
Technical and accessibility issues in hospitals often prevent patients from receiving optimal mental and physical health care, which is essential for independent living, especially as societies age and chronic diseases like diabetes and cardiovascular disease become more common. Recent advances in the Internet of Things (IoT)-enabled wearable devices offer potential solutions for remote health monitoring and everyday activity recognition, gaining significant attention in personalized healthcare. This paper comprehensively reviews wearable healthcare technology integrated with the IoT for continuous vital sign monitoring. Relevant papers were extracted and analyzed using a systematic numerical review method, covering various aspects such as sports monitoring, disease detection, patient monitoring, and medical diagnosis. The review highlights the transformative impact of IoT-enabled wearable devices in healthcare, facilitating real-time monitoring of vital signs, including blood pressure, temperature, oxygen levels, and heart rate. Results from the reviewed papers demonstrate high accuracy and efficiency in predicting health conditions, improving sports performance, enhancing patient care, and diagnosing diseases. The integration of IoT in wearable healthcare devices enables remote patient monitoring, personalized care, and efficient data transmission, ultimately transcending traditional boundaries of healthcare and leading to better patient outcomes.Keywords
Nomenclature
AI | Artificial Intelligence |
CGM | Continuous Glucose Monitoring |
FDA | Food and Drug Administration |
GDPR | General Data Protection Regulation |
HIPAA | Health Insurance Portability and Accountability Act |
IoT | Internet of Things |
WMS | Wearable Monitoring Systems |
Due to advancements in intelligent hardware, big data, and the internet, wearable healthcare technology has grown in popularity over the past few years [1–3]. Wearable medical devices may aid individuals in their pursuit of a healthier lifestyle and provide healthcare professionals with an uninterrupted flow of data. Nevertheless, the wearable medical device sector faces several significant obstacles that impede the expansion of wearable applications. These include challenges in developing user-friendly solutions, concerns regarding security and privacy, the absence of industry standards, and numerous technical impediments [4].
Scientists need to consider crucial aspects encompassing devices and metric choices when conducting sensor research. The utilization of wearable devices to generate data for advanced health research has significantly increased in demand over the past few years [5,6]. Consumer-grade, noninvasive wearables may offer numerous benefits for health research; they are typically unseen and inexpensive compared to research devices considered gold standards, convenient to wear, and accessible to consumers. Nevertheless, knowledge gaps have also generated apprehensions regarding the potential health hazards that wearables may present, either by inexplicably discouraging healthy behavior or by discouraging individuals from striving for objectives. To direct future clinical applications of wearable technology, it is crucial to have a more comprehensive comprehension of the characteristics that contribute to successful wearable implementation in clinical settings [7,8].
By providing personalized, timely, and cost-effective interventions that ultimately result in improved health outcomes and a higher standard of care in long-term care settings, incorporating the Internet of Things (IoT) into ubiquitous healthcare can disrupt the industry. In addition to recording dietary and medication information, wearable IoT care systems can monitor personal physiological indicators, including heart rate, blood pressure, and body temperature. The gathered data can be conveyed using wireless transmission to a central monitoring station for analysis and processing. This enables healthcare practitioners to obtain significant insights into the health condition of individuals and identify any irregularities or anomalies within the data. It furnishes valuable diagnostic and prognostic capabilities. The ubiquitous IoT system’s capabilities are further enhanced by incorporating AI algorithms for data analysis. AI algorithms can detect anomalies, patterns, and trends within data, offering significant diagnostic and prognostic capabilities [9–13].
The IoT is a crucial component in the early detection and intervention of diseases. Integrating sensor systems facilitates enhanced patient monitoring, reducing testing, avoidable consultations, and financial savings [14,15]. In an emergency, IoT applications can assist in rapidly notifying and warning physicians or caregivers of elderly individuals. Despite the manifest benefits of IoT technologies in the healthcare sector, including the mitigation of health problems such as medical errors, failures, and ineffectual workflows, IoT systems still need to achieve full integration within healthcare organizations. Adopting IoT applications necessitates a methodical process capable of discerning technological advancements; technology adoption is a well-established field of study in information systems, with particular emphasis on IoT adoption [16].
For the management of future pandemics, the IoT-based ubiquitous health surveillance system is a promising solution [17]. Eliminating the requirement for manual surveillance of patients in quarantine alleviates the workload of healthcare providers by remotely monitoring the precise whereabouts and physiological parameters of quarantined individuals. Healthcare providers can enhance their management of the COVID-19 pandemic by using the system, which can identify patients needing medical attention [18]. The gathered information may be submitted to a cloud-based database for healthcare providers to conduct remote monitoring. Early warning functions for medical emergencies and the ability to display real-time health data for multiple patients are capabilities of the monitor terminal. By doing so, the system effectively mitigates the workload of healthcare providers by eliminating the necessity for manual patient monitoring during quarantine [19]. Hussain Mir et al. [20] employed classification-based machine learning models to experiment with the COVID-19 primary novel dataset. Cross-validation ensured model consistency for the suggested models. Experimental findings show that our models have good accuracy, with Support Vector Machine reaching 97.767%. Experiments show that the suggested conceptual model will help discover and predict COVID-19 suspects and monitor patients to give emergency care in medical emergencies.
Implementing ubiquitous wireless devices for continuous vital sign monitoring holds promise for revolutionizing patient care by facilitating prompt intervention in the event of clinical deterioration and thereby enhancing overall patient outcomes. Multiple scholarly investigations have underscored the advantages and difficulties of continuously monitoring vital signs within hospital environments. The potential of ubiquitous monitoring systems to enhance the identification of deterioration and patient outcomes, particularly among high-risk patients, was underscored in a systematic review and meta-analysis by Areia et al. [21]. Nevertheless, the evaluation also identified constraints and impediments to execution, including the potential repercussions of cables and static devices on patient mobility and autonomy [21]. A survey-based study by Becking-Verhaar et al. [22] unveiled that nurses, on the whole, hold a favorable view of continuous vital sign monitoring. They highlight its benefits, including detecting patients who are deteriorating early on and saving time, which is especially beneficial during evening and night duties. A qualitative study by Leenen et al. [23] using the Behavior Change Wheel framework emphasized the significance of clinical assessment by nurses in conjunction with device measurements and the novelty of monitoring vital sign trends onwards.
Although the prospective advantages of continuous vital sign monitoring are apparent, scholarly literature has also underscored the difficulties associated with device precision, dependability, and the requirement for sufficient education and assistance for healthcare practitioners. The significance of resolving these obstacles to guarantee the effective integration and execution of continuous vital sign monitoring in clinical practice is emphasized by the studies. Moreover, the available evidence indicates that proficiently implementing continuous vital sign monitoring can result in timely intervention, early identification of deterioration, and enhanced patient outcomes; thus, it contributes to the paradigm shift in patient care.
Due to the rising demand for remote health monitoring solutions, this review rigorously analyzes the integration of IoT with wearable healthcare technology, filling a significant gap in the sector. This work is essential and unique since we focus on continuous vital sign monitoring and technical obstacles and breakthroughs. This article critically analyzes the potential for significant change that can result from integrating the IoT with ubiquitous healthcare technology, particularly emphasizing continuous vital sign monitoring. By combining IoT and wearables, real-time information on heart rate, blood pressure, temperature, and blood oxygen levels can be obtained, resulting in enhanced patient care, earlier detection of complications, and decreased healthcare costs. The discourse encompasses technological intricacies, such as the ability to transmit and communicate data in real-time and practical implementations in glucose monitoring, cancer treatment tracking, and post-operative care. The literature review, technological analysis, and evaluation of benefits and applications comprise the review, which concludes with insights into the challenges, implications, and prospects of this healthcare integration.
This study advances the area by offering a thorough overview of IoT-enabled wearable healthcare technology for continuous vital sign monitoring. Our contributions include (1) a detailed analysis of the underlying technologies, algorithms, and data processing methods; (2) a comparative study of existing methodologies; (3) an examination of applications and performance; and (4) a discussion of ethical, regulatory, and user experience issues.
2 Applications in Specific Health Monitoring Areas
In the medical field, wearable wireless devices for continuous vital sign monitoring are becoming increasingly common. Wearable devices can make early intervention possible by enabling the prompt diagnosis of clinical deterioration [24,25]. Patient outcomes are enhanced when automated alarms are combined with ongoing monitoring in the event of a deterioration [26]. To avoid reducing the patient’s movement, wearable technology should ideally be wireless, portable, and attached to an area of the body that is easily accessible [27]. These wearables may also be used to continuously monitor patients’ vital signs at home, reducing hospital stays and avoiding unanticipated readmissions [28].
It is standard procedure in intensive care, medium care, operating rooms, and recovery wards to monitor the vital signs of inpatients continuously. Vital signs are, however, only periodically checked on patients released to the general ward—typically just once or twice a day. Most wearable wireless continuous monitoring devices undergo feasibility and clinical validation testing. Currently, no excellent, sizable, well-monitored trials on wearable wireless technology demonstrate a discernible benefit in patient outcomes. Studies of this kind are required to support administrators and healthcare practitioners in making decisions about the use of wearable technology for continuous vital sign monitoring [29].
Current monitoring procedures may be enhanced by wearable monitoring systems (WMS). Nevertheless, there are several obstacles to deployment in the hospital setting, and the clinical impact of WMS on deterioration identification and patient outcomes still needs to be discovered. Though one research indicates it would be possible and cost-effective in surgical wards, with the potential to enhance patient outcomes compared to intermittent monitoring, continuous monitoring is not widely employed in the ward environment [21]. Wearable technology may offer a bridge between occasional manual measures and bedside wired monitors and a substitute for static wired continuous monitoring [30]. Wearable monitors make continuous vital sign monitoring, wireless data transmission to the electronic health record, and a decrease in unscheduled readmissions possible [31].
The use of wearable devices to control and track blood glucose levels in diabetics is known as glucose monitoring (Fig. 1). The notion of using fully noninvasive technology, like wearable devices, to control and track blood glucose levels in individuals with diabetes is still relatively new and in its early stages of development. The prevalent home electronic glucose meters need the person with diabetes to do an intrusive self-prick to draw blood, which exposes the individual to infections in addition to the stress and pain of having to undergo this procedure again, which is frequently required many times a day. The efficacy of wearable devices employing artificial intelligence (AI) for blood glucose level forecasting or prediction has been the subject of several studies [32]. By far, the most used sensor was the accelerometer; the glucose sensor came in second and was used to measure glucose in 46% of the most current research published [33]. One possible breakthrough in diabetes management is wearable continuous glucose monitoring (CGM) devices [34].
Figure 1: Glucose monitoring workflow using wearable technology and IoT integration
Machine learning approaches with noninvasive technologies like wearable devices can comprehend and draw useful conclusions. When utilized appropriately, these technologies can enhance patients’ quality of life without intrusive procedures, and they are readily available and reasonably priced [32]. The global proliferation of wearable and mobile technology presents novel prospects for researchers to track diabetes-associated indicators, including blood glucose levels, physical activity levels, and insulin dosages [35–37]. Diabetes treatment may undergo a revolution thanks to wearable CGM sensors [38]. Scientific research is stimulated by the development of CGM technology and applications [34].
Although much research utilizing wearable devices for diabetes has been published, few reports employ machine learning, and even fewer utilize wearable devices for blood glucose forecasting [32]. Patients’ broad usage of them may be hampered by the field’s complexity and quick changes. This article aims to give healthcare professionals a general understanding of CGM technology, discuss applying CGM data in clinical practice, and offer advice on incorporating CGM into daily work [39]. More research would be required to determine how accurate sweat detection is for determining the threshold glucose level [40].
Cancer treatment tracking, which involves monitoring the efficacy and the patient’s response to cancer treatments, is essential to cancer care. It has been demonstrated that patient-reported outcomes and symptom monitoring are valuable components of cancer care, given that they provide up-to-date information regarding the patient’s quality of life and symptoms. Symptom monitoring via weekly web-based patient-reported symptom monitoring treatment for lung cancer was associated with increased survival, according to a systematic literature review [41]. By utilizing patient-reported outcomes and symptom surveillance, medical professionals can more effectively identify and treat symptoms in their early stages, thereby improving patient outcomes [41].
Smartphone applications have also been created to assist cancer patients in monitoring their treatment progress. Numerous digital health applications for cancer were created to assist patients in monitoring their symptoms, medications, and appointments, according to a content analysis [42]. Patients may experience improved outcomes due to the organization and awareness of their treatment progress that these applications facilitate. However, a comprehensive evaluation of these applications’ efficacy in enhancing cancer treatment outcomes remains pending.
To enhance the efficacy of cancer treatment, novel methodologies and techniques have been devised, including gene therapy and stem cell therapy. The methodologies above encompass the insertion of exogenous genes directly into noncancerous tumors and the utilization of stem cells for regenerative medicine, therapeutic delivery, drug targeting, and immune cell generation [43]. Although preclinical studies have yielded encouraging results for these novel approaches, additional research is required to assess their efficacy in clinical environments.
In cancer care, big data and predictive analytics have also been utilized to assess patient risk. Healthcare providers can utilize these tools to identify patients who are at an elevated risk of developing cancer or enduring unfavorable treatment outcomes [44]. Healthcare providers can produce individualized treatment plans for every patient by leveraging big data and predictive analytics. This results in enhanced patient outcomes and improved quality of life.
Postoperative care is essential to the patient’s recuperation process, and several variables influence its quality and satisfaction. Involving patients in decisions regarding the timing of their interactions with healthcare services may result in a more person-centered approach to postoperative care. Furthermore, preoperative assessment should encompass variables such as the age, sex, mental health condition, and health literacy abilities of the patients, as these aspects may potentially hinder their recuperation after the procedure. In addition, using valid and dependable instruments for preoperative screening, providing pertinent preoperative and postoperative information, and establishing an accessible support system can all contribute to an individual’s enhanced recovery following surgery [45].
Patient-reported symptom monitoring has been linked to enhanced survival rates in cancer care. Furthermore, it may have further ramifications for clinical practice, including promoting patient disease awareness and improving patient-physician communication and relationships [46]. The significance of effective postoperative care and monitoring to prevent and manage surgical site infections, a major concern in postoperative recovery, is highlighted. The utilization of IoT and ubiquitous sensors in post-operative monitoring and patient care is illustrated in Fig. 2.
Figure 2: Post-surgery care flowchart with IoT and wearable sensors
3 Technological Nuances of Wearable Gadgets
Currently, vital signs are monitored using wearable devices with sensors such as heart rate, blood pressure, body temperature, electrocardiogram, and pulse oximetry. These devices collect, process, and store extended periods of physiological and activity data from the individual wearing them. The captured data is then transmitted to a robust remote computer or a cloud-based implementation, where the sensor data is analyzed, deconstructed, and meaningful conclusions are drawn, interpreted, and displayed to the user [47,48]. Concerns regarding security, power consumption, connectivity capability, and design limitations are the primary evaluation obstacles for wearable technology. The powering procedure presents the greatest challenge in fabricating implantable and peripheral devices. The capability of wearable devices is typically limited due to the restricted communication area for wireless transmissions. The issue of wearable interface security is still unresolved. These devices compromise the privacy and security of their consumers [48].
Real-time health surveillance systems based on the IoT can save the patient’s life by constantly monitoring a patient’s vital signs. Software, a data-acquisition module, and a microcontroller comprise these systems. The data from the patient’s electrocardiogram, heart rate, blood pressure, body temperature, and pulse oximetry, routinely recorded and stored by the system, are transmitted to the physician’s mobile phone for analysis. An alert system is also incorporated into the system; if the patient’s vital signs become aberrant, a message is transmitted to the physician’s cell phone [49].
The implementation of wearable sensors is widespread in aiding the transition of patient care from the clinic to the patient’s vehicle. By analyzing patients’ vital signs, their functionality can continuously quantify progress through treatment regimens. Wearable devices offer the benefit of presenting objective data regarding motion and condensing this data into a format that healthcare professionals can readily comprehend. They can also be implemented in remote areas where healthcare access is limited or patients are too ailing or disabled to visit a clinic or hospital physically.
3.1 Real-Time Data Transmission Capabilities
The rapid influx and substantial volume of real-time data frequently necessitate the implementation of effective processing and analysis methodologies [50]. Wearable devices produce substantial volumes of data that necessitate real-time transmission and processing to furnish precise insights and facilitate decision-making [51].
Rapid response times and minimal latency are essential for real-time applications. AI models need to be devised and optimized in real-time scenarios to deliver prompt insights and actions. Complex algorithms and high computational demands can impede real-time performance. Real-time data may contain outliers, be chaotic, or be incomplete. Guaranteeing data quality becomes arduous when data cleansing and validation time constraints arise. For AI models to effectively process chaotic data and generate precise predictions or decisions, they need to possess robustness [50].
Scalability is frequently necessary for real-time AI applications to manage massive amounts of incoming data [52]. It can be difficult to scale AI models and infrastructure in response to increased workload due to resource limitations such as network bandwidth and memory. Real-time data need to be synchronized for precise analysis and decision-making, regardless of its origin. Synchronizing and integrating data streams in real-time from diverse sources can prove intricate, especially when confronted with data in varying formats or time zones.
Real-time updating or retraining of AI models can present significant challenges. Ongoing learning and model adaptation might be necessary to integrate fresh data and modify model parameters in response to dynamic environments. It is critical to strike a balance between the requirement for real-time updates and the stability of the model. The ability to efficiently analyze and visualize real-time data to derive significant insights and facilitate decision-making is essential to real-time data transmission capabilities [53–55]. The utilization of interactive visualization tools and real-time analytics techniques is necessary for timely data processing and presentation.
Preliminary research indicates that wearable devices can collect objective, real-time activity data to evaluate the physical function and performance status in real time. This is a useful tool for prognostic and clinical decision-making, as subjective assessment of performance status is limited. Preliminary results indicate that daily step counts are positively correlated with patient-reported outcomes (such as tiredness, quality of life, and depressive symptoms) and provider-assessed performance status and that higher daily step counts are associated with lower probabilities of adverse events [56].
3.2 Communication Capabilities
Wearable devices communicate with healthcare providers using wireless technologies such as Bluetooth, Wi-Fi, and cellular networks [57,58]. By enabling remote monitoring and real-time data transfer, these technologies decrease the need for human monitoring while increasing efficiency.
Communication methods like Web Socket are employed to minimize overload and lower server bandwidth needs. These protocols provide efficient and safe data transfer while enabling real-time data transmission [59]. Real-time data processing with edge computing eases the load on cloud servers and speeds up response times [51]. Healthcare practitioners may now monitor patients remotely thanks to cloud-based storage, which eliminates the need for manual monitoring and boosts productivity [59].
Data is displayed, and healthcare practitioners are alerted to aberrant vital signs via mobile applications and alerts. Family members can also utilize these systems to keep an eye on the health of their loved ones [57]. Security procedures are put in place to safeguard patient data [59]. Confidentiality should be guaranteed by all parties involved, and communications should be encrypted and secure. Personal monitoring devices ought to discreetly verify the identification of the user by biometrics or important physiological indicators [57].
3.3 Integration with IoT platforms
The purpose of healthcare wearable IoT devices is to provide continuous vital sign monitoring, thereby reducing the workload of medical professionals and enhancing patient outcomes. Integrating these devices with IoT platforms facilitates real-time data transmission and permits remote monitoring [11].
Platforms for the IoT enable data processing and analytics, which aid in making decisions and provide insights into patient health [51]. These platforms employ sophisticated analytics methodologies such as AI and machine learning to scrutinize substantial quantities of data and deliver practical insights [11].
Strict security protocols are in place to safeguard patient information. All parties involved should ensure the confidentiality of communications through encryption and security measures. To verify user identity, personal monitoring devices should discreetly employ biometrics or critical physiological signs [60,61].
3.4 Data Accuracy and Validation
The reliability of data retrieved from wearable devices is highly dependent on the accuracy of their embedded sensors. Calibration procedures, whether involving software algorithms or physical standards, need to be performed regularly to compensate for drift in sensors and other variables that can impact the accuracy of measurements [62–64]. For a wide range of wearable device applications, accurate and dependable data gathering relies on proper sensor calibration [62–65].
Validation studies are crucial because they guarantee that wearable technology can deliver precise and trustworthy data for applications in healthcare. Measurement errors may result in incorrect diagnosis, inappropriate treatment, or lost opportunities for early intervention [66,67]. Skin tone, body composition, and environmental factors are potential biases, limits, or confounding factors that may affect device performance and are identified through validation studies [68,69].
Researchers and healthcare practitioners should carefully analyze wearable device validation studies and performance parameters for their intended use cases to ensure data accuracy and reliability. For reliable monitoring across user characteristics, transparent validation testing in diverse populations in lab and real-world settings is essential. Following regulatory clearances and industry standards can also help patients and providers trust wearable devices and their data [69].
Commercial wearable devices for measuring health parameters have been tested for validity and reliability. A review by Fuller et al. [70] found that commercial wearable devices can measure steps and heart rate in controlled laboratory settings, but accuracy varies by manufacturer and device type. The review revealed that Apple Watch and Garmin devices had higher step-counting accuracy, while Fitbit, Samsung, and Withings devices had a mean error of ±3%. In controlled conditions, Apple Watch, Fitbit, and Garmin devices measured heart rate within safe limits.
In real life, many factors can impair wearable device accuracy. Hrabovska et al. [71] list numerous problems, including data quality (noise, missing data), data integration (combining data from multiple sources), data privacy and security, and technical restrictions. Data analytics, machine learning, and sophisticated approaches are needed to gain insights from wearable device data. Research-grade and consumer devices have been found to differ in accuracy; consumer devices tend to be more accurate while at rest and less accurate when engaged in an activity [72].
The seamless integration of IoT within healthcare ecosystems is characterized by various challenges that need to be acknowledged. These require a symbiotic relationship between regulatory architects, healthcare professionals, and the broader research community to cultivate solutions that are both pragmatically viable and innovative [16].
While current approaches to IoT-enabled wearable healthcare technology have shown much promise, they also have some serious disadvantages. Variabilities in sensor accuracy and data-gathering methods are common problems with wearable technology, which can result in inconsistent or perhaps false health information. The implementation of wearable medical technology risks intensifying pre-existing technical and digital inequalities, resulting in uneven opportunities for reaping the advantages of these advancements. Another major area for improvement with IoT-enabled wearable healthcare devices is interoperability. Integrating wearable data with other health information systems is difficult due to a lack of standards across various platforms and devices [66].
As health monitoring systems based on IoT technologies are developed, security, privacy, and scalability concerns have been pointed out [73]. While the digitalization of healthcare in developing nations has made high-quality healthcare more accessible, it also highlights the need for improved data management and security solutions to address issues with privacy, security, affordability, and quality of care [74].
Wearable sensor accuracy and dependability can vary; some investigations have found inconsistent readings compared to clinical-grade equipment [47]. This calls into question the reliability of the information used to determine diagnoses and courses of treatment.
If not adequately secured, the sensitive health data these devices capture is susceptible to breaches, which could put patients at risk for identity theft, discrimination, or other negative outcomes [75]. Numerous wearable gadgets are on the market without explicit data protection requirements because regulatory supervision has not kept up with the quick development of wearable technologies. IoT healthcare devices are too expensive for general adoption, particularly in environments with limited resources. Devices could not work with public health insurance or need pricey subscriptions [76,77].
Traditional risk assessment methods may not effectively manage sophisticated cyber-attacks in real-time, underscoring the necessity of dynamic risk assessment approaches to safeguard users from security risks in Medical IoT devices [78]. The significance of secure data authentication and device wearability issues is underscored by the rise in connected medical devices, such as wearable smart infusion pumps, which raises concerns about cybersecurity threats and the potential disruption of device performance [79]. Safeguarding patients’ data in healthcare IoT systems is of the utmost importance, and it has resulted in the implementation of secure techniques such as blockchain to prevent security violations and protect electronic health records [80].
For our survey, an extensive literature search was conducted using Scopus with carefully chosen keywords (“wearable” AND “IoT” OR “Internet of Things” AND “health” OR “healthcare” OR “medical”) specifically targeting titles of papers. The initial search, performed in February 2024, yielded 445 papers. Subsequently, a systematic screening process was employed, narrowing the selection to journal articles published between 2019 and 2024. After applying inclusion and exclusion criteria, 63 papers were identified. A detailed review of abstracts led to the final selection of 20 papers deemed most relevant to wearable healthcare technology, IoT integration, and continuous vital sign monitoring (Fig. 3).
Figure 3: PRISMA flowchart
This method for wearable healthcare technologies and IoT integration has drawbacks. Scopus may be biased since other databases may have relevant publications that were not found in this search. The keywords may accidentally reject research employing alternative terminology, restricting the review’s scope. Focusing on journal publications and the 2019–2024 timeframe may skew findings from non-journal sources and earlier or later works. Abstracts for article selection and single-stage screening may add subjectivity and omit important facts, while the quality evaluation procedure is subjective and may miss key insights from lower-ranked journals. These limitations need to be acknowledged and addressed to interpret the findings and ensure review validity and reliability appropriately. Meta-analyses and longitudinal studies should be included in future research to manage the variability in patient outcomes and device performance effectively.
The collection of papers explores the multifaceted applications of wearable healthcare technology integrated with the IoT (Fig. 4).
Figure 4: Classification of papers
5.1 Monitoring Sports Performance and Health
The first set of papers focuses on leveraging IoT-based smart wearable systems for monitoring sports persons. Li et al. [81] introduced an IoT-smart wearable system achieving high accuracy (98.22%) in predicting sports person’s health, demonstrating the potential for enhancing sports performance through real-time health data. Keserwani et al. [82] emphasized the real-time analysis of wearable sensor data in healthcare, showcasing ongoing monitoring capabilities for better treatment, particularly in preventing burnout.
5.2 Wearable IoT for Disease Detection and Recommendation
Bokharaei et al. [83] and Zhang et al. [84] presented innovative approaches for disease detection and recommendation of wearable IoT devices. Bokharaei et al. [83] proposed a recommender framework using machine learning and social media sentiment analysis to recommend relevant wearable IoT devices for patients, achieving high accuracy (up to 95%). Zhang et al. [84] introduced wearable triboelectric sensors for gait analysis and waist motion capture, demonstrating potential applications in lower-limb and waist rehabilitation with high accuracy (98.4%).
5.3 Maternal Healthcare and Wearable IoT Platforms
Li et al. [85] and Wu et al. [86] contribute to the domain of maternal healthcare and wearable IoT platforms. Li et al. [85] proposed an IoT platform for smart maternal healthcare services, aiming to mitigate the workload of medical staff and improve obstetrical treatment. Wu et al. [86] designed a compact wearable sensor patch for health monitoring, emphasizing its feasibility for IoT-connected healthcare applications and remote monitoring.
5.4 IoT in Disease Prediction and Monitoring
The next cluster of papers explores the integration of IoT in disease prediction and monitoring. Ramasamy et al. [87] emphasized secure smart wearable computing through AI-enabled IoT for health monitoring. Nasser et al. [88] presented a cloud&deep learning-based wearable approach for monitoring diabetes, achieving high accuracy. Al-Makhadmeh et al. [89] utilized IoT for heart disease prediction, achieving a remarkable accuracy of 99.03%. Pradhan et al. [90] proposed a disease prediction and symptom recognition model using IoT, showing enhanced forecast rates and precision.
5.5 Innovative Technologies for Healthcare
The following papers cover various healthcare applications using innovative technologies. These include wearable health monitoring, IoT-connected e-textiles for neonatal medical monitoring [91,92], remote monitoring of COVID-19 patients [93], dynamic radio protocol selection for energy-constrained wearable IoT healthcare [94], automatic blood pressure monitoring using IoT [95], lung cancer diagnosis using IoT data [96], human activity recognition [97], energy-efficient IoT devices for healthcare data management [98], and a deep belief network for heart disease diagnosis [99]. Each study contributes to the broader landscape of technological advancements in healthcare
6.1 Implications of Continuous Vital Sign Monitoring
Continuous vital sign monitoring through wearable healthcare technology integrated with IoT has transformative implications across various healthcare domains. Wearable devices, such as the IoT-based Smart Wearable System for sports monitoring, enhance sports performance and contribute to injury prevention and overall athlete health. Real-time analysis of wearable sensor data in healthcare, as demonstrated by systems like those presented by Keserwani et al. [82], facilitated early intervention by sending alarms to healthcare professionals in case of abnormal vital signs. This continuous monitoring leads to better patient outcomes and reduces the likelihood of burnout for healthcare providers.
Integrating wearable IoT devices extends beyond individual health to data-driven approaches for disease classification and recommendation frameworks. Bokharaei et al. [83] introduced a wearable IoT intelligent recommender framework that uses machine learning algorithms to classify diseases and recommend suitable wearable devices based on social media sentiment analysis. This approach, validated by healthcare specialists, showcases a potential accuracy rate of up to 95% in recommending wearable devices for specific patient needs. These recommendations benefit patients and aid healthcare professionals in making informed decisions regarding personalized patient care.
Innovations such as triboelectric sensors for gait analysis and waist motion capture [84] and compact wearable sensor patches for health monitoring [86] highlight the versatility of continuous monitoring technologies. These devices provide insights into rehabilitation, user recognition, and motion monitoring, emphasizing their potential in physical therapy and rehabilitation robotics. The secure and predictive nature of IoT-connected healthcare, as seen in studies predicting diabetes [88] and remotely monitoring COVID-19 patients [93], underscores the broader impact on public health and disease management.
Energy-efficient solutions, like the proposed energy-efficient healthcare data management method by Ahamed et al. [100], address the practical challenges of continuous monitoring by minimizing energy consumption. Such efficiency is essential for the long-term use of wearable devices, ensuring sustained health monitoring without significant disruptions. As wearable IoT technologies evolve, their applications in disease detection, personalized healthcare recommendations, and remote monitoring are poised to revolutionize the healthcare landscape, offering a holistic approach to patient well-being and healthcare management.
6.2 Challenges and Limitations in Wearable Healthcare Technology
Wearable healthcare technology has the potential to revolutionize the healthcare industry by providing real-time monitoring of patient’s health conditions. However, several challenges and limitations need to be addressed to ensure the effectiveness and reliability of these technologies (Table 1). One of the main challenges is the accuracy and reliability of the data collected by wearable devices. The accuracy of the data collected by these devices is critical for accurate diagnosis and treatment of patients. For instance, Li et al. [81] developed an IoT-based smart wearable system for monitoring sports person activity to improve sports person health and performance healthily. The experimental result shows that the IoT-smart wearable system achieves the highest accuracy of 98.22% and efficiently predicts the sportsperson’s health to improve performance reliably.
Another challenge is the integration of wearable devices with existing healthcare systems. Integrating wearable devices with existing healthcare systems is essential to ensure that the data collected by these devices is used effectively to improve patient outcomes. Keserwani et al. [82] proposed a real-time analysis of wearable sensor data using IoT and machine learning in healthcare. This study aims to reduce the likelihood of burnout while providing better treatment to patients. The prototype facilitates the doctor’s ongoing monitoring of patient data. The use of IoT with machine learning can help us solve this issue.
Privacy and security concerns are also significant challenges in wearable healthcare technology. The data collected by wearable devices is sensitive and needs to be protected from unauthorized access. Ramasamy et al. [87] proposed secure smart wearable computing through AI-enabled IoT and Cyber-Physical Systems for Health Monitoring. The proposed system uses AI-enabled IoT and Cyber-Physical Systems to ensure the security and privacy of the data collected by wearable devices.
The cost of wearable devices is another limitation that needs to be addressed. The cost of wearable devices is a significant barrier to widespread healthcare adoption. Bokharaei et al. [83] proposed a wearable IoT intelligent recommender framework for a smarter healthcare approach. The proposed framework can help accelerate the transformation of smart hospitals. It can assist doctors in finding and suggesting the right wearable IoT for their patients smartly and efficiently during treatment for various diseases. Furthermore, wearable device manufacturers can also use the outcome of the proposed platform to develop personalized wearable devices for patients in the future.
Table 2 compares methods for integrating IoT-enabled wearable healthcare devices to meet significant problems. The issues are data privacy, battery life, accuracy, user comfort, and cost. We examine two methods for each problem, highlighting their results and supporting them with research. Cryptography and data transmission are secure but difficult to implement, while anonymization and data minimization are simpler but less secure. High-capacity rechargeable batteries increase device utilization but add weight, whereas body movement energy collecting is sustainable but unreliable. This extensive comparison, supported by references, shows the trade-offs and synergies between different techniques, helping readers grasp their potential and limitations in wearable healthcare technology.
Wearable healthcare technology and the IoT create serious ethical challenges. Data privacy, informed consent, and abuse of sensitive health information are the key concerns. Wearable technology collects sensitive health data that could violate privacy if breached. Strong encryption can be employed during transmission and storage to reduce this. Informed consent requires consumers to understand the data being gathered, how it will be used, and who will have access to it. Users need to opt in and out of consent easily. Data misuse, such as sharing data without permission and using it for unintended ends, requires strict rules and morality. User confidence and privacy rights depend on morality in developing, deploying, and overseeing these technologies.
To ensure patient safety, data security, and device efficacy, IoT-enabled wearable healthcare devices need to comply with a complicated set of standards and laws. The GDPR (General Data Protection Regulation) in Europe, HIPAA (Health Insurance Portability and Accountability Act) in the United States, and FDA (Food and Drug Administration) medical device standards are important rules [101,102]. Clinical trials to prove safety and efficacy are needed to meet regulatory standards. For smooth integration and functionality, healthcare systems need to be interoperable and follow defined communication protocols [103]. Documentation and audits must be updated to stay compliant and address regulatory changes. Compliance strategies must also include constant security monitoring and updating to protect patient data from new cyber threats [101,104].
Long-term wearable healthcare device success depends on energy efficiency and patient compliance. Energy-efficient design needs to include low-power sensors and optimized data transfer methods to improve battery life and reduce recharging. This improves user convenience and the possibility of continued device use, which is essential for continuous health monitoring. Innovative energy harvesting methods like body heat or kinetic energy can boost device operability. Wearables need to be lightweight, unobtrusive, and user-friendly to ensure patient compliance. Patients of all ages and tech levels should be able to use gadgets with simple and accessible user interfaces. Patient feedback should be used to improve device design and functionality, improving compliance and health outcomes over time [4,105,106].
The IoT and wearable healthcare technology have recently advanced, which has been well documented in current studies. Despite these developments, there are still several important gaps and difficulties that recent research has started to address. While recent research highlights the high accuracy of AI-driven prediction models, for instance, thorough studies on integrating them with IoT platforms for seamless data processing and transmission still need to be completed. Standardizing communication protocols and ensuring secure data transmission across various healthcare ecosystems still need much work [107]. Recent works have focused on the junction of ethical considerations and technological progress [47,108]. Through synthesizing these findings, this review not only provides a summary of the current state-of-the-art but also highlights important gaps in the literature, thereby offering a roadmap for future research and development in IoT-integrated wearable healthcare equipment.
6.3 Prospects and Potential Advancements in the Field
Wearable healthcare technology, coupled with IoT integration, holds substantial promise for the future of patient care and health monitoring. The integration of these technologies allows for continuous vital sign monitoring, providing real-time data on parameters such as blood pressure, temperature, blood oxygen levels, and heart rate. This transformative approach facilitates early problem detection, resulting in improved patient care and potential cost savings for the healthcare system. The advantages extend beyond mere data collection; wearables enable better patient interaction, real-time data processing, and remote monitoring, empowering healthcare professionals with individualized patient care capabilities.
In specific health monitoring areas, such as glucose monitoring, cancer treatment tracking, and post-surgery care, wearable IoT devices are proving to be instrumental. The papers discussed highlight innovative applications, including a smart wearable system for sports and physical health, real-time analysis of wearable sensor data for healthcare, and a wearable IoT intelligent recommender framework for personalized healthcare recommendations. Furthermore, the integration of triboelectric sensors for gait analysis and waist motion capture, the development of an IoT platform for smart maternal healthcare services, and the design of a rigid-flex wearable health monitoring sensor patch showcase the diverse applications and potential advancements in the field.
The future holds exciting prospects, with researchers exploring novel approaches like using wearable IoT devices for disease prediction, such as heart disease and diabetes. Additionally, the development of secure smart wearable computing through AI-enabled IoT and cyber-physical systems, advancements in deep learning models for health monitoring, and the creation of energy-efficient IoT devices for healthcare data management showcase the breadth of possibilities. The continuous evolution of wearable healthcare technology with IoT integration is poised to transcend traditional healthcare boundaries, ushering in a new era of personalized and efficient healthcare delivery. Future advancements in IoT-based wearable systems may include:
• Enhanced data processing and analysis capabilities, such as real-time machine learning algorithms and edge computing.
• Improved wearable device design, including flexible and lightweight materials, and energy-efficient components.
• Integration of multiple sensors and data sources for more comprehensive health monitoring.
• Development of personalized wearable devices based on individual health profiles and preferences.
• Expansion of wearable systems to cover a broader range of health conditions and applications.
• Enhanced data security and privacy measures to protect sensitive health information.
• Collaboration between wearable device manufacturers, healthcare providers, and researchers to develop innovative solutions.
The key findings from the reviewed papers underscore the transformative impact of wearable healthcare technology integrated with the IoT. These technologies enable continuous vital sign monitoring, offering real-time health data on parameters such as blood pressure, temperature, blood oxygen levels, and heart rate. Notable achievements include high accuracy rates in predicting sports persons’ health, innovative recommender frameworks for disease detection, and the development of compact, flexible wearable sensor patches for diverse physiological measurements.
The integration of wearable healthcare technology with IoT holds significant importance in revolutionizing patient care. These technologies facilitate better patient interaction, early detection of health issues, and a reduction in healthcare expenses. The ability to provide continuous streams of health data into the digital sphere transcends traditional healthcare boundaries. Wearable IoT devices enable personalized care, and efficient health monitoring in critical areas such as glucose monitoring and cancer treatment tracking, and empower professionals with remote patient monitoring capabilities. The research highlights the potential for these technologies to enhance sports performance, maternal healthcare, disease prediction, and overall well-being.
The outlook for continuous vital sign monitoring in healthcare appears promising and transformative. The ongoing advancements in wearable healthcare technology, coupled with IoT integration, suggest a future where patients and healthcare providers can benefit from seamless, real-time health data. The development of intelligent recommender frameworks, energy-efficient IoT devices, and novel deep-learning models indicate a trend toward more sophisticated and accurate health monitoring solutions. As the field progresses, it is anticipated that wearable devices will play an increasingly integral role in disease prevention, early diagnosis, and personalized treatment plans.
The reviewed papers collectively highlight the transformative potential of wearable healthcare technology with IoT integration. The findings demonstrate the feasibility and efficacy of continuous vital sign monitoring across various healthcare domains. The importance of these technologies lies in their ability to revolutionize patient care, offering real-time data for better decision-making, personalized treatments, and enhanced overall well-being. Looking forward, the continuous evolution of these technologies is likely to shape the future of healthcare, bringing about advancements that contribute to more proactive and personalized approaches to patient health management. As wearables become more sophisticated and widely adopted, their role in healthcare is poised to expand, ushering in an era of connected, data-driven, and patient-centric healthcare solutions.
Acknowledgement: None.
Funding Statement: The author received no specific funding for this study.
Availability of Data and Materials: Not applicable.
Ethics Approval: Not applicable.
Conflicts of Interest: The author declares that they have no conflicts of interest to report regarding the present study.
References
1. F. Firouzi et al., “Internet-of-Things and big data for smarter healthcare: From device to architecture, applications and analytics,” Future Gener. Comput. Syst., vol. 78, no. 2, pp. 583–586, 2018. doi: 10.1016/j.future.2017.09.016. [Google Scholar] [CrossRef]
2. H. Taherdoost, “Blockchain-based Internet of Medical Things,” Appl. Sci., vol. 13, no. 3, 2023, Art. no. 1287. doi: 10.3390/app13031287. [Google Scholar] [CrossRef]
3. C. Wall, V. Hetherington, and A. Godfrey, “Beyond the clinic: The rise of wearables and smartphones in decentralising healthcare,” NPJ Digit. Med., vol. 6, no. 1, 2023, Art. no. 219. doi: 10.1038/s41746-023-00971-z. [Google Scholar] [PubMed] [CrossRef]
4. L. Lu et al., “Wearable health devices in health care: Narrative systematic review,” JMIR Mhealth Uhealth, vol. 8, no. 11, 2020, Art. no. e18907. doi: 10.2196/18907. [Google Scholar] [PubMed] [CrossRef]
5. J. Lee, D. Kim, H. -Y. Ryoo, and B. -S. Shin, “Sustainable wearables: Wearable technology for enhancing the quality of human life,” Sustainability, vol. 8, no. 5, 2016, Art. no. 466. doi: 10.3390/su8050466. [Google Scholar] [CrossRef]
6. N. Niknejad, W. B. Ismail, A. Mardani, H. Liao, and I. Ghani, “A comprehensive overview of smart wearables: The state of the art literature, recent advances, and future challenges,” Eng. Appl. Artif. Intell., vol. 90, 2020, Art. no. 103529. doi: 10.1016/j.engappai.2020.103529. [Google Scholar] [CrossRef]
7. M. Smuck, C. A. Odonkor, J. K. Wilt, N. Schmidt, and M. A. Swiernik, “The emerging clinical role of wearables: Factors for successful implementation in healthcare,” npj Digit. Med., vol. 4, no. 1, pp. 1–8, 2021. doi: 10.1038/s41746-021-00418-3. [Google Scholar] [PubMed] [CrossRef]
8. S. Huhn et al., “The impact of wearable technologies in health research: Scoping review,” JMIR Mhealth Uhealth, vol. 10, no. 1, 2022, Art. no. e34384. doi: 10.2196/34384. [Google Scholar] [PubMed] [CrossRef]
9. W. -H. Wang and W. -S. Hsu, “Integrating artificial intelligence and wearable IoT system in long-term care environments,” Sensors, vol. 23, no. 13, 2023, Art. no. 5913. doi: 10.3390/s23135913. [Google Scholar] [PubMed] [CrossRef]
10. M. Al-rawashdeh, P. Keikhosrokiani, B. Belaton, M. Alawida, and A. Zwiri, “IoT adoption and application for smart healthcare: A systematic review,” Sensors, vol. 22, no. 14, 2022, Art. no. 5377. doi: 10.3390/s22145377. [Google Scholar] [PubMed] [CrossRef]
11. N. Surantha, P. Atmaja, and M. Wicaksono, “A review of wearable internet-of-things device for healthcare,” Procedia Comput. Sci., vol. 179, no. 11, pp. 936–943, 2021. doi: 10.1016/j.procs.2021.01.083. [Google Scholar] [CrossRef]
12. S. Khan, S. Tsutsumi, T. Yairi, and S. Nakasuka, “Robustness of AI-based prognostic and systems health management,” Annu. Rev. Control, vol. 51, no. 17, pp. 130–152, 2021. doi: 10.1016/j.arcontrol.2021.04.001. [Google Scholar] [CrossRef]
13. A. Sonavane, A. Khamparia, and D. Gupta, “A systematic review on the Internet of Medical Things: Techniques, open issues, and future directions,” Comput. Model. Eng. Sci., vol. 137, no. 2, pp. 1525–1550, 2023. doi: 10.32604/cmes.2023.028203. [Google Scholar] [CrossRef]
14. P. K. D. Pramanik et al., “Internet of Things, smart sensors, and pervasive systems: Enabling connected and pervasive healthcare,” in Healthcare Data Analytics and Management. Amsterdam, Netherlands: Elsevier, pp. 1–58, 2019. [Google Scholar]
15. A. S. Albahri et al., “IoT-based telemedicine for disease prevention and health promotion: State-of-the-Art,” J. Netw. Comput. Appl., vol. 173, no. 1, 2021, Art. no. 102873. doi: 10.1016/j.jnca.2020.102873. [Google Scholar] [CrossRef]
16. C. Li, J. Wang, S. Wang, and Y. Zhang, “A review of IoT applications in healthcare,” Neurocomputing, vol. 565, no. 9, 2023, Art. no. 127017. doi: 10.1016/j.neucom.2023.127017. [Google Scholar] [CrossRef]
17. M. Nasajpour, S. Pouriyeh, R. M. Parizi, M. Dorodchi, M. Valero and H. R. Arabnia, “Internet of Things for current COVID-19 and future pandemics: An exploratory study,” J. Healthc. Inform. Res., vol. 4, no. 4, pp. 325–364, 2020. doi: 10.1007/s41666-020-00080-6. [Google Scholar] [PubMed] [CrossRef]
18. M. Javaid and I. H. Khan, “Internet of Things (IoT) enabled healthcare helps to take the challenges of COVID-19 Pandemic,” J. Oral Biol. Craniofac. Res., vol. 11, no. 2, pp. 209–214, 2021. doi: 10.1016/j.jobcr.2021.01.015. [Google Scholar] [PubMed] [CrossRef]
19. J. -Y. Wu, Y. Wang, C. T. S. Ching, H. -M. D. Wang, and L. -D. Liao, “IoT-based wearable health monitoring device and its validation for potential critical and emergency applications,” Front. Public Health, vol. 11, 2023, Art. no. 1188304. doi: 10.3389/fpubh.2023.1188304. [Google Scholar] [PubMed] [CrossRef]
20. M. Hussain Mir, S. Jamwal, U. Iqbal, A. Mehbodniya, J. Webber and U. Hafiz Khan, “A novel edge-assisted IoT-ML-based smart healthcare framework for COVID-19,” Comput. Model. Eng. Sci., vol. 137, no. 3, pp. 2529–2565, 2023. doi: 10.32604/cmes.2023.027173. [Google Scholar] [CrossRef]
21. C. Areia et al., “The impact of wearable continuous vital sign monitoring on deterioration detection and clinical outcomes in hospitalised patients: A systematic review and meta-analysis,” Crit. Care, vol. 25, no. 1, 2021, Art. no. 351. doi: 10.1186/s13054-021-03766-4. [Google Scholar] [PubMed] [CrossRef]
22. F. L. Becking-Verhaar, R. P. H. Verweij, M. de Vries, H. Vermeulen, H. van Goor and G. J. Huisman-de Waal, “Continuous vital signs monitoring with a wireless device on a general ward: A survey to explore nurses’ experiences in a post-implementation period,” Int. J. Environ. Res. Public Health, vol. 20, no. 10, 2023, Art. no. 5794. doi: 10.3390/ijerph20105794. [Google Scholar] [PubMed] [CrossRef]
23. J. P. L. Leenen, E. M. Dijkman, A. van Hout, C. J. Kalkman, L. Schoonhoven and G. A. Patijn, “Nurses’ experiences with continuous vital sign monitoring on the general surgical ward: A qualitative study based on the Behaviour Change Wheel,” BMC Nurs., vol. 21, no. 1, 2022, Art. no. 60. doi: 10.1186/s12912-022-00837-x. [Google Scholar] [PubMed] [CrossRef]
24. D. Dias and J. P. S. Cunha, “Wearable health devices—Vital sign monitoring, systems and technologies,” Sensors, vol. 18, no. 8, 2018, Art. no. 2414. doi: 10.3390/s18082414. [Google Scholar] [PubMed] [CrossRef]
25. M. M. Baig, S. Afifi, H. GholamHosseini, and E. Ullah, “Deterioration to decision: A comprehensive literature review of rapid response applications for deteriorating patients in acute care settings,” Health Technol., vol. 10, no. 3, pp. 567–573, 2020. doi: 10.1007/s12553-019-00403-7. [Google Scholar] [CrossRef]
26. R. Blythe, R. Parsons, N. M. White, D. Cook, and S. McPhail, “A scoping review of real-time automated clinical deterioration alerts and evidence of impacts on hospitalised patient outcomes,” BMJ Qual. Saf., vol. 31, no. 10, pp. 725–734, 2022. doi: 10.1136/bmjqs-2021-014527. [Google Scholar] [PubMed] [CrossRef]
27. T. Amin, R. J. Mobbs, N. Mostafa, L. W. Sy, and W. J. Choy, “Wearable devices for patient monitoring in the early postoperative period: A literature review,” Mhealth, vol. 7, no. 50, 2021. doi: 10.21037/mhealth-20-131. [Google Scholar] [PubMed] [CrossRef]
28. J. P. Leenen, V. Ardesch, and G. Patijn, “Remote home monitoring of continuous vital sign measurements by wearables in patients discharged after colorectal surgery: Observational feasibility study,” JMIR Perioper. Med., vol. 6, 2023, Art. no. e45113. doi: 10.2196/45113. [Google Scholar] [PubMed] [CrossRef]
29. J. P. L. Leenen, H. J. M. Rasing, C. J. Kalkman, L. Schoonhoven, and G. A. Patijn, “Process evaluation of a wireless wearable continuous vital signs monitoring intervention in 2 general hospital wards: Mixed methods study,” JMIR Nurs., vol. 6, 2023, Art. no. e44061. doi: 10.2196/44061. [Google Scholar] [PubMed] [CrossRef]
30. I. Ahmad et al., “Emerging technologies for next generation remote health care and assisted living,” IEEE Access, vol. 10, no. 3, pp. 56094–56132, 2022. doi: 10.1109/ACCESS.2022.3177278. [Google Scholar] [CrossRef]
31. C. S. Webster, T. W. Scheeren, and Y. I. Wan, “Patient monitoring, wearable devices, and the healthcare information ecosystem,” Br. J. Anaesth., vol. 128, no. 5, pp. 756–758, 2022. doi: 10.1016/j.bja.2022.02.034. [Google Scholar] [PubMed] [CrossRef]
32. A. Ahmed, S. Aziz, A. Abd-alrazaq, F. Farooq, M. Househ and J. Sheikh, “The effectiveness of wearable devices using artificial intelligence for blood glucose level forecasting or prediction: Systematic review,” J. Med. Internet Res., vol. 25, no. 2, 2023, Art. no. e40259. doi: 10.2196/40259. [Google Scholar] [PubMed] [CrossRef]
33. C. Rodriguez-León, C. Villalonga, M. Munoz-Torres, J. R. Ruiz, and O. Banos, “Mobile and wearable technology for the monitoring of diabetes-related parameters: Systematic review,” JMIR Mhealth Uhealth, vol. 9, no. 6, 2021, Art. no. e25138. doi: 10.2196/25138. [Google Scholar] [PubMed] [CrossRef]
34. G. Cappon, G. Acciaroli, M. Vettoretti, A. Facchinetti, and G. Sparacino, “Wearable continuous glucose monitoring sensors: A revolution in diabetes treatment,” Electronics, vol. 6, no. 3, 2017, Art. no. 65. doi: 10.3390/electronics6030065. [Google Scholar] [CrossRef]
35. W. H. SK, S. Pal Singh, and P. Johri, “A comprehensive review on securities and privacy preservation in IoT healthcare application for diabetics,” in 2021 Second Int. Conf. Electron. Sustain. Commun. Syst. (ICESC), Coimbatore, India, IEEE, 2021, pp. 706–711. doi: 10.1109/ICESC51422.2021.9532855. [Google Scholar] [CrossRef]
36. I. Odenigbo, “AR Dancee: An augmented reality-based mobile persuasive app for promoting physical activity through dancing,” 2023. Accessed: Apr. 10, 2024. [Online]. Available: http://hdl.handle.net/10222/82882 [Google Scholar]
37. H. S. Pramanik, A. Pal, M. Kirtania, T. Chakravarty, and A. Ghose, “Smartphone-based sensors in health and wellness monitoring–Perspectives and assessment of the emerging future,” in Smartphone-Based Detection Devices: Emerging Trends in Analytical Techniques, 2021, pp. 375–398. doi: 10.1016/B978-0-12-823696-3.00018-0. [Google Scholar] [CrossRef]
38. C. Chen, X. -L. Zhao, Z. -H. Li, Z. -G. Zhu, S. -H. Qian and A. Flewitt, “Current and emerging technology for continuous glucose monitoring,” Sensors, vol. 17, no. 1, 2017, Art. no. 182. doi: 10.3390/s17010182. [Google Scholar] [PubMed] [CrossRef]
39. D. F. Kruger, S. V. Edelman, D. A. Hinnen, and C. G. Parkin, “Reference guide for integrating continuous glucose monitoring into clinical practice,” Diabetes Educ., vol. 45, no. 1_suppl, pp. 3S–20S, 2019. doi: 10.1177/0145721718818066. [Google Scholar] [PubMed] [CrossRef]
40. A. A. Smith, R. Li, and Z. T. H. Tse, “Reshaping healthcare with wearable biosensors,” Sci. Rep., vol. 13, no. 1, 2023, Art. no. 4998. doi: 10.1038/s41598-022-26951-z. [Google Scholar] [PubMed] [CrossRef]
41. L. Lizan, L. Perez-Carbonell, and M. Comellas, “Additional value of patient-reported symptom monitoring in cancer care: A systematic review of the literature,” Cancers, vol. 13, no. 18, 2021, Art. no. 4615. doi: 10.3390/cancers13184615. [Google Scholar] [PubMed] [CrossRef]
42. D. H. Charbonneau et al., “Smartphone apps for cancer: A content analysis of the digital health marketplace,” Digit. Health, vol. 6, 2020. doi: 10.1177/2055207620905413. [Google Scholar] [PubMed] [CrossRef]
43. D. T. Debela et al., “New approaches and procedures for cancer treatment: Current perspectives,” SAGE Open Med., vol. 9, no. 6, 2021. doi: 10.1177/20503121211034366. [Google Scholar] [PubMed] [CrossRef]
44. R. B. Parikh, A. Gdowski, D. A. Patt, A. Hertler, C. Mermel and J. E. Bekelman, “Using big data and predictive analytics to determine patient risk in oncology,” Am. Soc. Clin. Oncol. Educ. Book, vol. 39, no. 39, pp. e53–e58, 2019. doi: 10.1200/EDBK_238891. [Google Scholar] [PubMed] [CrossRef]
45. M. Jaensson, K. Dahlberg, and U. Nilsson, “Factors influencing day surgery patients’ quality of postoperative recovery and satisfaction with recovery: A narrative review,” Perioper. Med., vol. 8, no. 1, pp. 1–7, 2019. doi: 10.1186/s13741-019-0115-1. [Google Scholar] [PubMed] [CrossRef]
46. S. Timmermans, “The engaged patient: The relevance of patient-physician communication for twenty-first-century health,” J. Health Soc. Behav., vol. 61, no. 3, pp. 259–273, 2020. doi: 10.1177/0022146520943514. [Google Scholar] [PubMed] [CrossRef]
47. K. Zovko, L. Šerić, T. Perković, H. Belani, P. Šolić, “IoT and health monitoring wearable devices as enabling technologies for sustainable enhancement of life quality in smart environments,” J. Clean. Prod., vol. 413, 2023, Art. no. 137506. doi: 10.1016/j.jclepro.2023.137506. [Google Scholar] [CrossRef]
48. V. Vijayan, J. P. Connolly, J. Condell, N. McKelvey, P. Gardiner, “Review of wearable devices and data collection considerations for connected health,” Sensors, vol. 21, no. 16, 2021, Art. no. 5589. doi: 10.3390/s21165589. [Google Scholar] [PubMed] [CrossRef]
49. S. Abdulmalek et al., “IoT-based healthcare-monitoring system towards improving quality of life: A review,” Healthcare, vol. 10, no. 10, 2022. doi: 10.3390/healthcare10101993. [Google Scholar] [PubMed] [CrossRef]
50. A. Aldoseri, K. N. Al-Khalifa, and A. M. Hamouda, “Re-Thinking data strategy and integration for artificial intelligence: Concepts, opportunities, and challenges,” Appl. Sci., vol. 13, no. 12, 2023, Art. no. 7082. doi: 10.3390/app13127082. [Google Scholar] [CrossRef]
51. W. A. Günther, M. H. Rezazade Mehrizi, M. Huysman, and F. Feldberg, “Debating big data: A literature review on realizing value from big data,” J. Strateg. Inf. Syst., vol. 26, no. 3, pp. 191–209, 2017. doi: 10.1016/j.jsis.2017.07.003. [Google Scholar] [CrossRef]
52. E. Baccour et al., “Pervasive AI for IoT applications: A survey on resource-efficient distributed artificial intelligence,” IEEE Commun. Surv. Tutor., vol. 24, no. 4, pp. 2366–2418, 2022. doi: 10.1109/COMST.2022.3200740. [Google Scholar] [CrossRef]
53. J. M. Tien, “Internet of Things, real-time decision making, and artificial intelligence,” Ann. Data Sci., vol. 4, no. 2, pp. 149–178, 2017. doi: 10.1007/s40745-017-0112-5. [Google Scholar] [CrossRef]
54. Y. Wang, L. Kung, and T. A. Byrd, “Big data analytics: Understanding its capabilities and potential benefits for healthcare organizations,” Technol. Forecast. Soc. Change, vol. 126, no. 4, pp. 3–13, 2018. doi: 10.1016/j.techfore.2015.12.019. [Google Scholar] [CrossRef]
55. F. Pigni, G. Piccoli, and R. Watson, “Digital data streams: Creating value from the real-time flow of big data,” Calif. Manage. Rev., vol. 58, no. 3, pp. 5–25, 2016. doi: 10.1525/cmr.2016.58.3.5. [Google Scholar] [CrossRef]
56. Y. Liao, C. Thompson, S. Peterson, J. Mandrola, and M. S. Beg, “The future of wearable technologies and remote monitoring in health care,” Am. Soc. Clin. Oncol. Educ. Book, vol. 39, pp. 115–121, 2019. doi: 10.1200/EDBK_238919. [Google Scholar] [PubMed] [CrossRef]
57. T. Loncar-Turukalo, E. Zdravevski, J. M. da Silva, I. Chouvarda, V. Trajkovik, “Literature on wearable technology for connected health: Scoping review of research trends, advances, and barriers,” J. Med. Internet Res., vol. 21, no. 9, 2019, Art. no. e14017. doi: 10.2196/14017. [Google Scholar] [PubMed] [CrossRef]
58. G. Gardašević, K. Katzis, D. Bajić, L. Berbakov, “Emerging wireless sensor networks and Internet of Things technologies—Foundations of smart healthcare,” Sensors, vol. 20, no. 13, 2020, Art. no. 3619. doi: 10.3390/s20133619. [Google Scholar] [PubMed] [CrossRef]
59. J. J. Ferreira, C. I. Fernandes, H. G. Rammal, and P. M. Veiga, “Wearable technology and consumer interaction: A systematic review and research agenda,” Comput. Human Behav., vol. 118, no. 32021. doi: 10.1016/j.chb.2021.106710. [Google Scholar] [CrossRef]
60. M. S. Obaidat, I. Traore, and I. Woungang, “Biometric security and Internet of Things (IoT),” Biom.-Based Phys. Cybersecur. Syst., vol. 17, no. 4, pp. 477–509, 2019. doi: 10.1007/978-3-319-98734-7_19. [Google Scholar] [CrossRef]
61. J. Ashbourn, Biometrics: Advanced Identity Verification: The Complete Guide. Berlin, Germany: Springer, 2014. [Google Scholar]
62. S. Y. Hayoun et al., “Physics and semantic informed multi-sensor calibration via optimization theory and self-supervised learning,” Sci. Rep., vol. 14, no. 1, 2024, Art. no. 2541. doi: 10.1038/s41598-024-53009-z. [Google Scholar] [PubMed] [CrossRef]
63. A. Sabir and A. Zakriti, “Simplification of calibration of low-cost MARG sensors without high-precision laboratory equipment,” Int. Rev. Appl. Sci. Eng., vol. 14, no. 2, pp. 170–182, 2023. doi: 10.1556/1848.2022.00469. [Google Scholar] [CrossRef]
64. Q. Wang, J. Cui, Y. Tang, L. Pang, K. Chen and B. Zhang, “Research on a precision calibration model of a flexible strain sensor based on a variable section cantilever beam,” Sensors, vol. 23, no. 10, 2023, Art. no. 4778. doi: 10.3390/s23104778. [Google Scholar] [PubMed] [CrossRef]
65. O. Samuelsson, E. U. Lindblom, A. Björk, and B. Carlsson, “To calibrate or not to calibrate, that is the question,” Water Res., vol. 229, no. 14, 2023, Art. no. 119338. doi: 10.1016/j.watres.2022.119338. [Google Scholar] [PubMed] [CrossRef]
66. S. Canali, V. Schiaffonati, and A. Aliverti, “Challenges and recommendations for wearable devices in digital health: Data quality, interoperability, health equity fairness,” PLOS Digit. Health, vol. 1, no. 10, 2022, Art. no. e0000104. doi: 10.1371/journal.pdig.0000104. [Google Scholar] [PubMed] [CrossRef]
67. S. Barteit et al., “Feasibility, acceptability and validation of wearable devices for climate change and health research in the low-resource contexts of Burkina Faso and Kenya: Study protocol,” PLoS One, vol. 16, no. 9, 2021, Art. no. e0257170. doi: 10.1371/journal.pone.0257170. [Google Scholar] [PubMed] [CrossRef]
68. L. G. Roos and G. M. Slavich, “Wearable technologies for health research: Opportunities, limitations, and practical and conceptual considerations,” Brain Behav. Immun., vol. 113, no. 1, pp. 444–452, 2023. doi: 10.1016/j.bbi.2023.08.008. [Google Scholar] [PubMed] [CrossRef]
69. J. R. Walter, S. Xu, and J. A. Rogers, “From lab to life: How wearable devices can improve health equity,” Nat. Commun., vol. 15, no. 1, 2024, Art. no. 123. doi: 10.1038/s41467-023-44634-9. [Google Scholar] [PubMed] [CrossRef]
70. D. Fuller et al., “Reliability and validity of commercially available wearable devices for measuring steps, energy expenditure, and heart rate: Systematic review,” JMIR Mhealth Uhealth, vol. 8, no. 9, 2020, Art. no. e18694. doi: 10.2196/18694. [Google Scholar] [PubMed] [CrossRef]
71. N. Hrabovska, E. Kajati, and I. Zolotova, “A validation study to confirm the accuracy of wearable devices based on health data analysis,” Electronics, vol. 12, no. 11, 2023, Art. no. 2536. doi: 10.3390/electronics12112536. [Google Scholar] [CrossRef]
72. B. Bent, B. A. Goldstein, W. A. Kibbe, and J. P. Dunn, “Investigating sources of inaccuracy in wearable optical heart rate sensors,” NPJ Digit. Med., vol. 3, no. 1, 2020, Art. no. 18. doi: 10.1038/s41746-020-0226-6. [Google Scholar] [PubMed] [CrossRef]
73. B. Yadav, A. Gupta, N. Sharma, and Y. Sharma, “IoT-enabled health monitoring systems: Transforming healthcare delivery,” Int. J. Res. Appl. Sci. Eng. Technol., vol. 12, no. 11, pp. 1108–1116, 2024. doi: 10.22214/ijraset.2024.58516. [Google Scholar] [CrossRef]
74. S. Narula, N. R. Sharma, and G. Nagpal, “Role of IoT in healthcare inclusion in developing countries: A systematic literature review,” in 2023 Int. Conf. Sustain. Emerg. Innovat. Eng. Technol. (ICSEIET), Ghaziabad, India, IEEE, 2023, pp. 869–875. doi: 10.1109/ICSEIET58677.2023.10303631. [Google Scholar] [CrossRef]
75. S. Kouchaki, X. Ding, and S. Sanei, “AI- and IoT-enabled solutions for healthcare,” Sensor, vol. 24, no. 8, 2024, Art. no. 2607. doi: 10.3390/s24082607. [Google Scholar] [PubMed] [CrossRef]
76. A. M. Rahmani et al., “Exploiting smart e-Health gateways at the edge of healthcare Internet-of-Things: A fog computing approach,” Future Gener. Comput. Syst., vol. 78, no. 7, pp. 641–658, 2018. doi: 10.1016/j.future.2017.02.014. [Google Scholar] [CrossRef]
77. M. Usak, M. Kubiatko, M. S. Shabbir, O. Viktorovna Dudnik, K. Jermsittiparsert and L. Rajabion, “Health care service delivery based on the Internet of Things: A systematic and comprehensive study,” Int. J. Commun. Syst., vol. 33, no. 2, 2020, Art. no. e4179. doi: 10.1002/dac.4179. [Google Scholar] [CrossRef]
78. R. M. Czekster, P. Grace, C. Marcon, F. Hessel, and S. C. Cazella, “Challenges and opportunities for conducting dynamic risk assessments in medical IoT,” Appl. Sci., vol. 13, no. 13, 2023, Art. no. 7406. doi: 10.3390/app13137406. [Google Scholar] [CrossRef]
79. Y. Vaseghi, B. Behara, and M. Delrobaei, “Towards evaluating the security of wearable devices in the Internet of Medical Things,” in 2023 11th RSI Int. Conf. Robot. Mechatron. (ICRoM), Tehran, Islamic Republic of Iran, IEEE, 2023, pp. 526–531. doi: 10.1109/ICRoM60803.2023.10412353. [Google Scholar] [CrossRef]
80. N. Chauhan and R. K. Dwivedi, “A secure design of the healthcare IoT system using blockchain technology,” in 2022 9th Int. Conf. Comput. Sustain. Global Develop. (INDIACom), New Delhi, India, IEEE, 2022, pp. 704–709. doi: 10.23919/INDIACom54597.2022.9763187. [Google Scholar] [CrossRef]
81. F. Li, O. S. Martínez, and R. S. Aiswarya, “Internet of Things-based smart wearable system to monitor sports person health,” Technol. Health Care, vol. 29, no. 6, pp. 1249–1262, 2021. doi: 10.3233/THC-213004. [Google Scholar] [PubMed] [CrossRef]
82. H. Keserwani, S. V. Kakade, S. K. Sharma, M. Manchanda, and G. F. Nama, “Real-time analysis of wearable sensor data using IoT and machine learning in healthcare,” Int. J. Intell. Syst. Appl. Eng., vol. 11, no. 7s, pp. 85–90, 2023. [Google Scholar]
83. M. Bokharaei Nia, M. Afshar Kazemi, C. Valmohammadi, and G. Abbaspour, “Wearable IoT intelligent recommender framework for a smarter healthcare approach,” Libr. Hi Tech., vol. 41, no. 4, pp. 1238–1261, 2023. doi: 10.1108/LHT-04-2021-0151. [Google Scholar] [CrossRef]
84. Q. Zhang et al., “Wearable triboelectric sensors enabled gait analysis and waist motion capture for IoT-based smart healthcare applications,” Adv. Sci., vol. 9, no. 4, 2022. doi: 10.1002/advs.202103694. [Google Scholar] [PubMed] [CrossRef]
85. X. Li, Y. Lu, X. Fu, and Y. Qi, “Building the Internet of Things platform for smart maternal healthcare services with wearable devices and cloud computing,” Future Gener. Comput. Syst., vol. 118, no. 8, pp. 282–296, 2021. doi: 10.1016/j.future.2021.01.016. [Google Scholar] [CrossRef]
86. T. Wu, F. Wu, C. Qiu, J. -M. Redoute, and M. R. Yuce, “A rigid-flex wearable health monitoring sensor patch for IoT-connected healthcare applications,” IEEE Internet Things J., vol. 7, no. 8, pp. 6932–6945, 2020. doi: 10.1109/JIOT.2020.2977164. [Google Scholar] [CrossRef]
87. L. K. Ramasamy, F. Khan, M. Shah, B. V. V. S. Prasad, C. Iwendi and C. Biamba, “Secure smart wearable computing through artificial intelligence-enabled Internet of Things and cyber-physical systems for health monitoring,” Sensors, vol. 22, no. 3, 2022. doi: 10.3390/s22031076. [Google Scholar] [PubMed] [CrossRef]
88. A. R. Nasser et al., “IoT and cloud computing in health-care: A new wearable device and cloud-based deep learning algorithm for monitoring of diabetes,” Electronics, vol. 10, no. 21, 2021. doi: 10.3390/electronics10212719. [Google Scholar] [CrossRef]
89. Z. Al-Makhadmeh and A. Tolba, “Utilizing IoT wearable medical device for heart disease prediction using higher order Boltzmann model: A classification approach,” Measure.: J. Int. Measure. Confed., vol. 147, no. 2, 2019. doi: 10.1016/j.measurement.2019.07.043. [Google Scholar] [CrossRef]
90. M. R. Pradhan, K. Ateeq, and B. Mago, “Wearable device based on IoT in the healthcare system for disease detection and symptom recognition,” Int. J. Artif. Intell. Tools, vol. 30, no. 6–8, pp. 6–8, 2021. doi: 10.1142/S021821302140011X. [Google Scholar] [CrossRef]
91. M. Manas, A. Sinha, S. Sharma, and M. R. Mahboob, “A novel approach for IoT based wearable health monitoring and messaging system,” J. Ambient Intell. Humaniz. Comput., vol. 10, no. 7, pp. 2817–2828, 2019. doi: 10.1007/s12652-018-1101-z. [Google Scholar] [CrossRef]
92. G. Cay et al., “NeoWear: An IoT-connected e-textile wearable for neonatal medical monitoring,” Pervasive Mob. Comput., vol. 86, no. 1, 2022, Art. no. 101679. doi: 10.1016/j.pmcj.2022.101679. [Google Scholar] [CrossRef]
93. M. M. Jaber et al., “Remotely monitoring COVID-19 patient health condition using metaheuristics convolute networks from IoT-based wearable device health data,” Sensors, vol. 22, no. 3, 2022. doi: 10.3390/s22031205. [Google Scholar] [PubMed] [CrossRef]
94. S. Misra, A. Roy, C. Roy, and A. Mukherjee, “Dynamic radio protocol selection for energy-constrained wearable IoT healthcare,” IEEE J. Sel. Areas Commun., vol. 39, no. 2, pp. 338–345, 2021. doi: 10.1109/JSAC.2020.3020678. [Google Scholar] [CrossRef]
95. A. Kaewpoonsuk et al., “Automatic blood pressure for wearable health monitoring using IoT technology,” ICIC Express Lett. Part B: Appl., vol. 12, no. 5, pp. 479–486, 2021. [Google Scholar]
96. N. Faruqui, M. A. Yousuf, M. Whaiduzzaman, A. K. M. Azad, A. Barros and M. A. Moni, “LungNet: A hybrid deep-CNN model for lung cancer diagnosis using CT and wearable sensor-based medical IoT data,” Comput. Biol. Med., vol. 139, no. 3, 2021. doi: 10.1016/j.compbiomed.2021.104961. [Google Scholar] [PubMed] [CrossRef]
97. A. Iqbal et al., “Wearable Internet-of-Things platform for human activity recognition and health care,” Int. J. Distrib. Sens. Netw., vol. 16, no. 6, 2020. doi: 10.1177/1550147720911561. [Google Scholar] [CrossRef]
98. J. Lou, F. Yang, and C. Lv, “Detection and application of wearable devices based on Internet of Things in human physical health,” Comput. Intell. Neurosci., vol. 2022, no. 1, 2022, Art. no. 5678736. doi: 10.1155/2022/5678736. [Google Scholar] [PubMed] [CrossRef]
99. F. Aliyar Vellameeran and T. Brindha, “A new variant of deep belief network assisted with optimal feature selection for heart disease diagnosis using IoT wearable medical devices,” Comput. Methods Biomech. Biomed. Eng., vol. 25, no. 4, pp. 387–411, 2022. doi: 10.1080/10255842.2021.1955360. [Google Scholar] [PubMed] [CrossRef]
100. B. Ahamed, S. Sellamuthu, P. N. Karri, I. V. Srinivas, A. N. Mohammed Zabeeulla and M. Ashok Kumar, “Design of an energy-efficient IoT device-assisted wearable sensor platform for healthcare data management,” Measure.: Sens., vol. 30, no. 10, 2023. doi: 10.1016/j.measen.2023.100928. [Google Scholar] [CrossRef]
101. J. B. Brönneke, J. Müller, K. Mouratis, J. Hagen, and A. D. Stern, “Regulatory, legal, and market aspects of smart wearables for cardiac monitoring,” Sensors, vol. 21, no. 14, 2021, Art. no. 4937. doi: 10.3390/s21144937. [Google Scholar] [PubMed] [CrossRef]
102. J. Calvillo-Arbizu, I. Román-Martínez, and J. Reina-Tosina, “Internet of Things in health: Requirements, issues, and gaps,” Comput. Methods Programs Biomed., vol. 208, no. 4, 2021. doi: 10.1016/j.cmpb.2021.106231. [Google Scholar] [PubMed] [CrossRef]
103. S. Jabbar, F. Ullah, S. Khalid, M. Khan, and K. Han, “Semantic interoperability in heterogeneous IoT infrastructure for healthcare,” Wirel. Commun. Mob. Comput., vol. 2017, no. 1, 2017, Art. no. 9731806. doi: 10.1155/2017/9731806. [Google Scholar] [CrossRef]
104. R. Alharbi and H. Almagwashi, “The privacy requirements for wearable IoT devices in healthcare domain,” in 2019 7th Int. Conf. Future Internet Things and Cloud Workshops (FiCloudW), Istanbul, Turkey, IEEE, 2019, pp. 18–25. doi: 10.1109/FiCloudW.2019.00017. [Google Scholar] [CrossRef]
105. L. Fazzini et al., “Does patient compliance influence wearable cardioverter defibrillator effectiveness? A single-center experience,” J. Clin. Med., vol. 12, no. 14, 2023, Art. no. 7473. doi: 10.3390/jcm12144743. [Google Scholar] [PubMed] [CrossRef]
106. S. O. Ajakwe, C. I. Nwakanma, D. -S. Kim, and J. -M. Lee, “Key wearable device technologies parameters for innovative healthcare delivery in B5G network: A review,” IEEE Access, vol. 10, no. 1, pp. 49956–49974, 2022. doi: 10.1109/ACCESS.2022.3173643. [Google Scholar] [CrossRef]
107. S. D. Mamdiwar, A. R, Z. Shakruwala, U. Chadha, K. Srinivasan, and C. -Y. Chang, “Recent advances on IoT-assisted wearable sensor systems for healthcare monitoring,” Biosensors, vol. 11, no. 10, 2021, Art. no. 372. doi: 10.3390/bios11100372. [Google Scholar] [PubMed] [CrossRef]
108. A. Atianashie Miracle and C. C. Adaobi, “Advancement in the healthcare field of wearable technology and future perspective,” J. Mat Sci Apl Eng, vol. 2, pp. 1–9, 2023. [Google Scholar]
Cite This Article
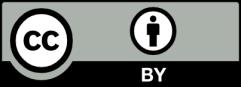
This work is licensed under a Creative Commons Attribution 4.0 International License , which permits unrestricted use, distribution, and reproduction in any medium, provided the original work is properly cited.