Open Access
ARTICLE
HQNN-SFOP: Hybrid Quantum Neural Networks with Signal Feature Overlay Projection for Drone Detection Using Radar Return Signals—A Simulation
School of Cyberspace Security, Information Engineering University, Zhengzhou, 450001, China
* Corresponding Author: Zheng Shan. Email:
(This article belongs to the Special Issue: Advanced Artificial Intelligence and Machine Learning Frameworks for Signal and Image Processing Applications)
Computers, Materials & Continua 2024, 81(1), 1363-1390. https://doi.org/10.32604/cmc.2024.054055
Received 17 May 2024; Accepted 14 September 2024; Issue published 15 October 2024
Abstract
With the wide application of drone technology, there is an increasing demand for the detection of radar return signals from drones. Existing detection methods mainly rely on time-frequency domain feature extraction and classical machine learning algorithms for image recognition. This method suffers from the problem of large dimensionality of image features, which leads to large input data size and noise affecting learning. Therefore, this paper proposes to extract signal time-domain statistical features for radar return signals from drones and reduce the feature dimension from 512 × 4 to 16 dimensions. However, the downscaled feature data makes the accuracy of traditional machine learning algorithms decrease, so we propose a new hybrid quantum neural network with signal feature overlay projection (HQNN-SFOP), which reduces the dimensionality of the signal by extracting the statistical features in the time domain of the signal, introduces the signal feature overlay projection to enhance the expression ability of quantum computation on the signal features, and introduces the quantum circuits to improve the neural network’s ability to obtain the inline relationship of features, thus improving the accuracy and migration generalization ability of drone detection. In order to validate the effectiveness of the proposed method, we experimented with the method using the MM model that combines the real parameters of five commercial drones and random drones parameters to generate data to simulate a realistic environment. The results show that the method based on statistical features in the time domain of the signal is able to extract features at smaller scales and obtain higher accuracy on a dataset with an SNR of 10 dB. On the time-domain feature data set, HQNN-SFOP obtains the highest accuracy compared to other conventional methods. In addition, HQNN-SFOP has good migration generalization ability on five commercial drones and random drones data at different SNR conditions. Our method verifies the feasibility and effectiveness of signal detection methods based on quantum computation and experimentally demonstrates that the advantages of quantum computation for information processing are still valid in the field of signal processing, it provides a highly efficient method for the drone detection using radar return signals.Keywords
Cite This Article
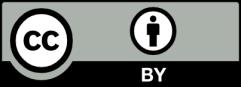
This work is licensed under a Creative Commons Attribution 4.0 International License , which permits unrestricted use, distribution, and reproduction in any medium, provided the original work is properly cited.