Open Access
ARTICLE
Automatic Extraction of Medical Latent Variables from ECG Signals Utilizing a Mutual Information-Based Technique and Capsular Neural Networks for Arrhythmia Detection
Department of Computer Engineering and Information Technology, Razi University, Kermanshah, 6714414971, Iran
* Corresponding Author: Fardin Abdali-Mohammadi. Email:
(This article belongs to the Special Issue: Emerging Trends and Applications of Deep Learning for Biomedical Signal and Image Processing)
Computers, Materials & Continua 2024, 81(1), 971-983. https://doi.org/10.32604/cmc.2024.053817
Received 10 May 2024; Accepted 03 September 2024; Issue published 15 October 2024
Abstract
From a medical perspective, the 12 leads of the heart in an electrocardiogram (ECG) signal have functional dependencies with each other. Therefore, all these leads report different aspects of an arrhythmia. Their differences lie in the level of highlighting and displaying information about that arrhythmia. For example, although all leads show traces of atrial excitation, this function is more evident in lead II than in any other lead. In this article, a new model was proposed using ECG functional and structural dependencies between heart leads. In the prescreening stage, the ECG signals are segmented from the QRS point so that further analyzes can be performed on these segments in a more detailed manner. The mutual information indices were used to assess the relationship between leads. In order to calculate mutual information, the correlation between the 12 ECG leads has been calculated. The output of this step is a matrix containing all mutual information. Furthermore, to calculate the structural information of ECG signals, a capsule neural network was implemented to aid physicians in the automatic classification of cardiac arrhythmias. The architecture of this capsule neural network has been modified to perform the classification task. In the experimental results section, the proposed model was used to classify arrhythmias in ECG signals from the Chapman dataset. Numerical evaluations showed that this model has a precision of 97.02%, recall of 96.13%, F1-score of 96.57% and accuracy of 97.38%, indicating acceptable performance compared to other state-of-the-art methods. The proposed method shows an average accuracy of 2% superiority over similar works.Keywords
Cite This Article
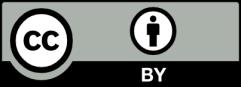
This work is licensed under a Creative Commons Attribution 4.0 International License , which permits unrestricted use, distribution, and reproduction in any medium, provided the original work is properly cited.