Open Access
ARTICLE
Leveraging EfficientNetB3 in a Deep Learning Framework for High-Accuracy MRI Tumor Classification
1 Department of Computer Science & Engineering, Faculty of Engineering and Technology, JAIN (Deemed-to-be University), Bengaluru, 562112, India
2 School of Computer Science and Engineering, VIT-AP University, Amaravati, 522241, India
3 Department of Computer Science & Technology, Madanapalle Institute of Technology & Science, Madanapalle, 517325, India
4 School of Science, Engineering and Environment, University of Salford, Manchester, M5 4WT, UK
5 School of Computer Science Engineering & Information Systems (SCORE), Vellore Institute of Technology (VIT), Vellore, 632014, India
6 Management Information System Department, College of Business Administration, King Saud University, Riyadh, 11531, Saudi Arabia
7 Adjunct Research Faculty, Centre for Research Impact & Outcome, Chitkara University, Rajpura, Punjab, 140401, India
* Corresponding Author: Surbhi Bhatia Khan. Email:
(This article belongs to the Special Issue: Medical Imaging Based Disease Diagnosis Using AI)
Computers, Materials & Continua 2024, 81(1), 867-883. https://doi.org/10.32604/cmc.2024.053563
Received 04 May 2024; Accepted 07 August 2024; Issue published 15 October 2024
Abstract
Brain tumor is a global issue due to which several people suffer, and its early diagnosis can help in the treatment in a more efficient manner. Identifying different types of brain tumors, including gliomas, meningiomas, pituitary tumors, as well as confirming the absence of tumors, poses a significant challenge using MRI images. Current approaches predominantly rely on traditional machine learning and basic deep learning methods for image classification. These methods often rely on manual feature extraction and basic convolutional neural networks (CNNs). The limitations include inadequate accuracy, poor generalization of new data, and limited ability to manage the high variability in MRI images. Utilizing the EfficientNetB3 architecture, this study presents a groundbreaking approach in the computational engineering domain, enhancing MRI-based brain tumor classification. Our approach highlights a major advancement in employing sophisticated machine learning techniques within Computer Science and Engineering, showcasing a highly accurate framework with significant potential for healthcare technologies. The model achieves an outstanding 99% accuracy, exhibiting balanced precision, recall, and F1-scores across all tumor types, as detailed in the classification report. This successful implementation demonstrates the model’s potential as an essential tool for diagnosing and classifying brain tumors, marking a notable improvement over current methods. The integration of such advanced computational techniques in medical diagnostics can significantly enhance accuracy and efficiency, paving the way for wider application. This research highlights the revolutionary impact of deep learning technologies in improving diagnostic processes and patient outcomes in neuro-oncology.Keywords
Cite This Article
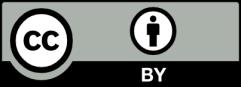
This work is licensed under a Creative Commons Attribution 4.0 International License , which permits unrestricted use, distribution, and reproduction in any medium, provided the original work is properly cited.